An Instance- and Label-Based Feature Selection Method in Classification Tasks
Information(2023)
摘要
Feature selection is crucial in classification tasks as it helps to extract relevant information while reducing redundancy. This paper presents a novel method that considers both instance and label correlation. By employing the least squares method, we calculate the linear relationship between each feature and the target variable, resulting in correlation coefficients. Features with high correlation coefficients are selected. Compared to traditional methods, our approach offers two advantages. Firstly, it effectively selects features highly correlated with the target variable from a large feature set, reducing data dimensionality and improving analysis and modeling efficiency. Secondly, our method considers label correlation between features, enhancing the accuracy of selected features and subsequent model performance. Experimental results on three datasets demonstrate the effectiveness of our method in selecting features with high correlation coefficients, leading to superior model performance. Notably, our approach achieves a minimum accuracy improvement of 3.2% for the advanced classifier, lightGBM, surpassing other feature selection methods. In summary, our proposed method, based on instance and label correlation, presents a suitable solution for classification problems.
更多查看译文
关键词
feature selection, manifold learning, classification
AI 理解论文
溯源树
样例
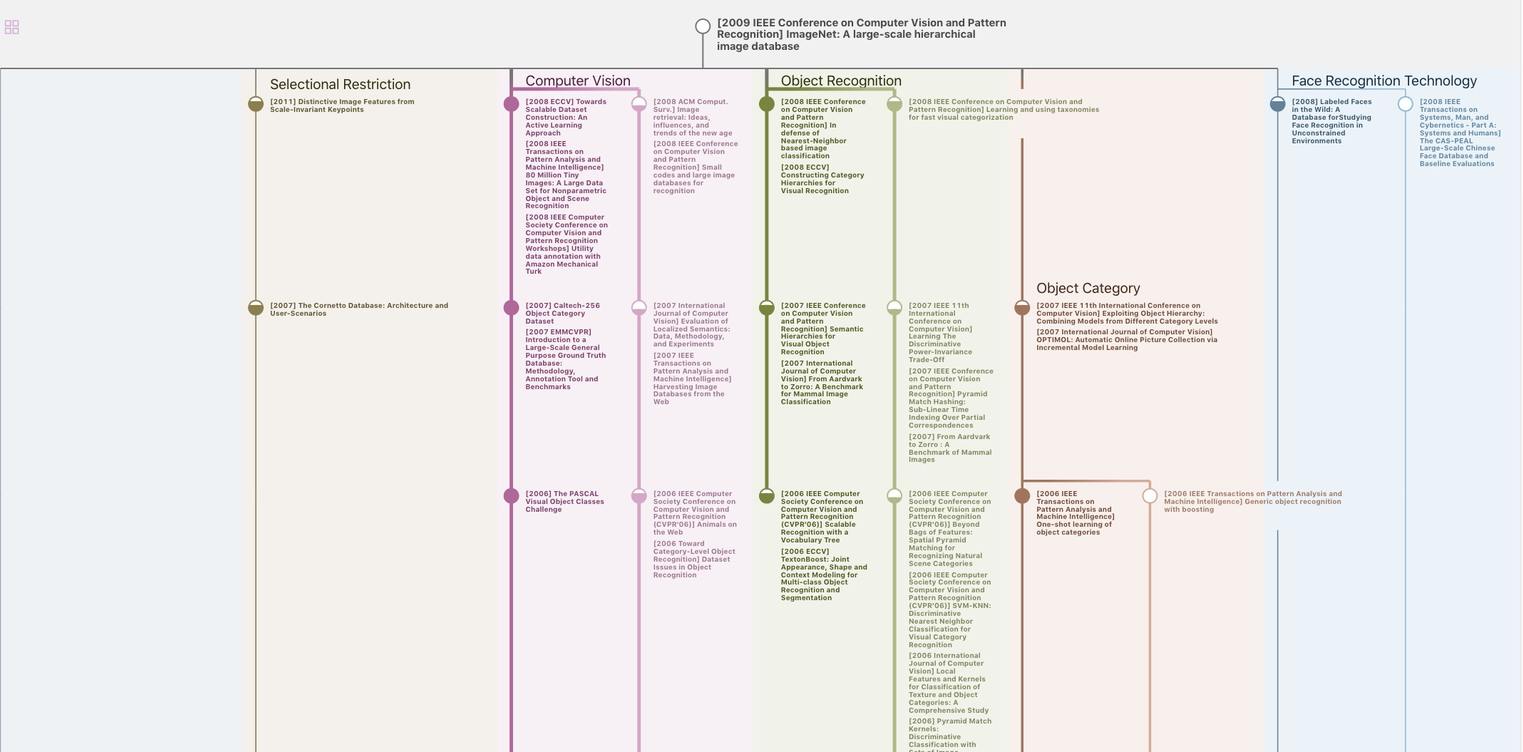
生成溯源树,研究论文发展脉络
Chat Paper
正在生成论文摘要