Short-Range Localization via Bluetooth Using Machine Learning Techniques for Industrial Production Monitoring
Journal of Sensor and Actuator Networks(2023)
摘要
Indoor short-range localization is crucial in many Industry 4.0 applications. Production monitoring for assembly lines, for instance, requires fine-grained positioning for parts or goods in order to keep track of the production process and the stations traversed by each product. Due to the unavailability of the Global Positioning System (GPS) for indoor positioning, a different approach is required. In this paper, we propose a specific design for short-range indoor positioning based on the analysis of the Received Signal Strength Indicator (RSSI) of Bluetooth beacons. To this aim, different machine learning techniques are considered and assessed: regressors, Convolution Neural Network (CNN) and Recurrent Neural Network (RNN). A realistic testbed is created to collect data for the training of the models and to assess the performance of each technique. Our analysis highlights the best models and the most convenient and suitable configuration for indoor localization. Finally, the localization accuracy is calculated in the considered use case, i.e., production monitoring. Our results show that the best performance is obtained using the K-Nearest Neighbors technique, which results in a good performance for general localization and in a high level of accuracy, 99%, for industrial production monitoring.
更多查看译文
关键词
bluetooth,localization,monitoring,industrial,short-range
AI 理解论文
溯源树
样例
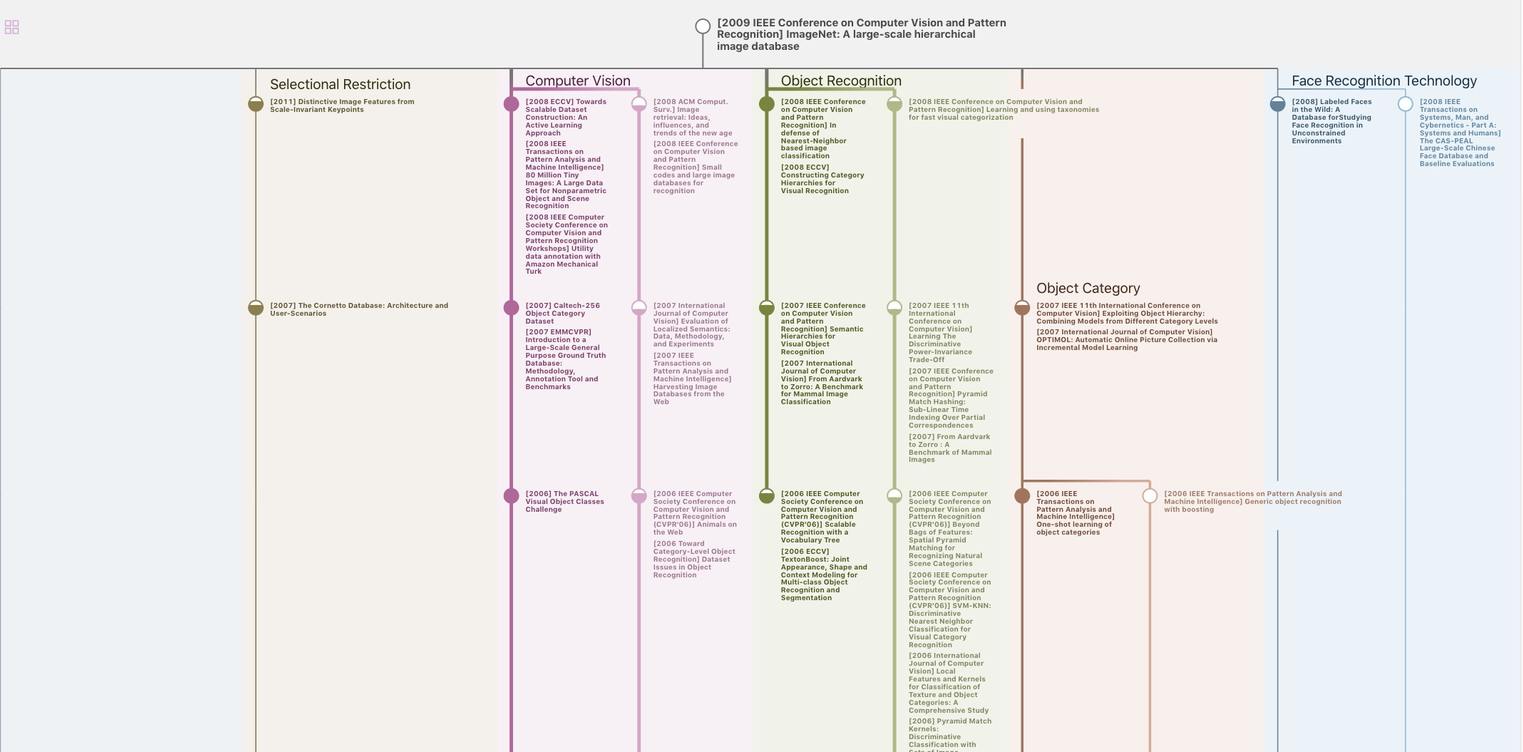
生成溯源树,研究论文发展脉络
Chat Paper
正在生成论文摘要