Explanatory Machine Learning Accelerates the Design of Graphene-Reinforced Aluminium Matrix Composites with Superior Performance
Metals(2023)
摘要
Addressing the exceptional properties of aluminium alloy composites reinforced with graphene, this study presents an interpretable machine learning approach to aid in the rapid and efficient design of such materials. Initially, data on these composites were gathered and optimised in order to create a dataset of composition/process-property. Several machine learning algorithms were used to train various models. The SHAP method was used to interpret and select the best performing model, which happened to be the CatBoost model. The model achieved accurate predictions of hardness and tensile strength, with coefficients of determination of 0.9597 and 0.9882, respectively, and average relative errors of 6.02% and 5.01%, respectively. The results obtained from the SHAP method unveiled the correlation between the composition, process and properties of aluminium alloy composites reinforced with graphene. By comparing the predicted and experimental data in this study, all machine learning models exhibited prediction errors within 10%, confirming their ability to generalise. This study offers valuable insights and support for designing high-performance aluminium matrix composites reinforced with graphene and showcases the implementation of machine learning in materials science.
更多查看译文
关键词
graphene-reinforced aluminium alloy composites, composition/process-property relationship, machine learning, SHAP methods, mechanical properties of composites
AI 理解论文
溯源树
样例
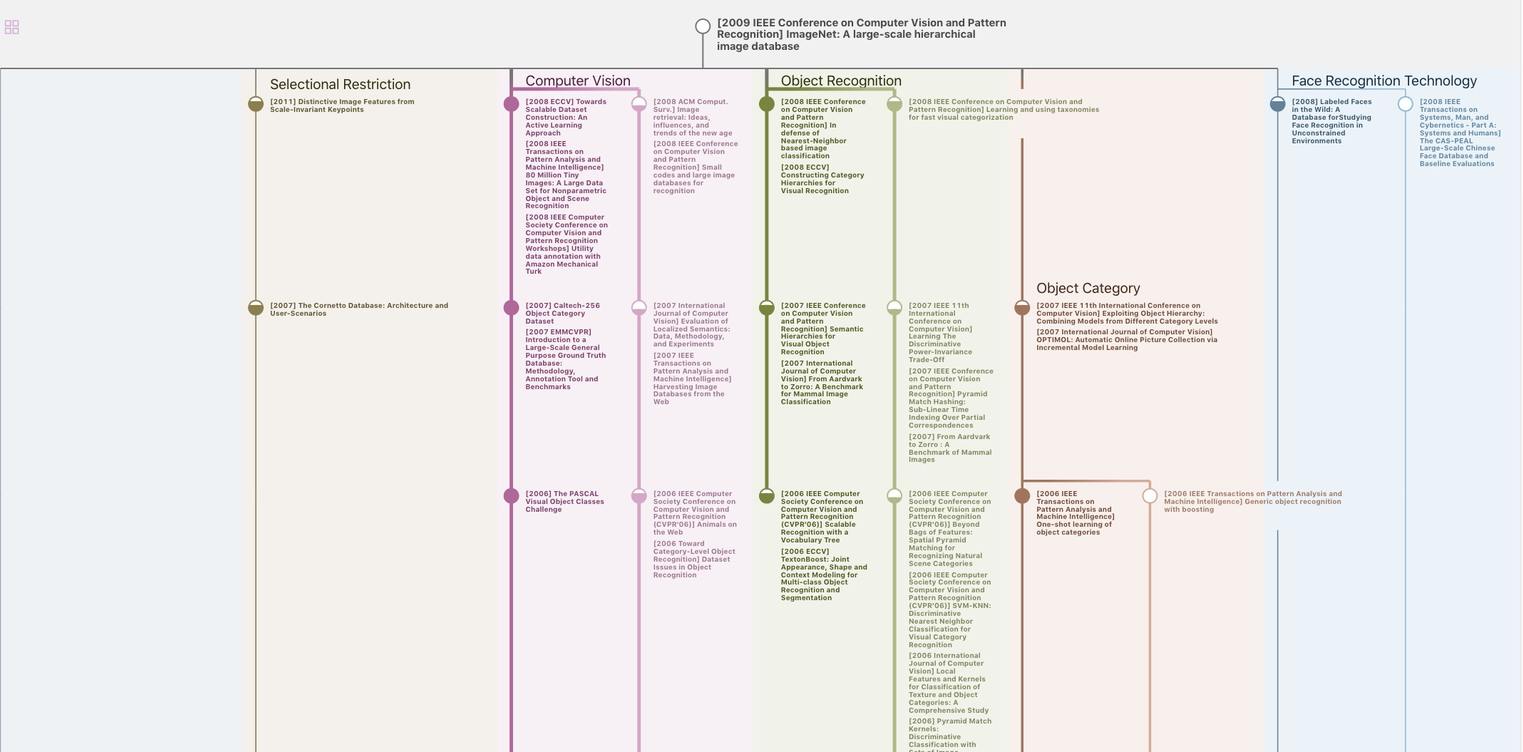
生成溯源树,研究论文发展脉络
Chat Paper
正在生成论文摘要