Disconnector Fault Diagnosis Based on Multi-Granularity Contrast Learning
Processes(2023)
摘要
Most disconnector fault diagnosis methods have high accuracy in model training. However, it is a challenging task to maintain high accuracy, a faster diagnosis speed, and less computation in practical situations. In this paper, we propose a multi-granularity contrastive learning (MG-CL) framework. First, the original disconnector current data are transformed into two different but related classes: strongly enhanced and weakly enhanced data, by using the strong and weak enhancement modules. Second, we propose the coarse-grained contrastive learning module to preliminarily judge the possibility of faults by learning the features of strongly/weakly enhanced data. Finally, in order to further judge the fault causes, we propose a fine-grained contrastive learning module. By comparing the differences in the data, the final fault type was judged. Our proposed MG-CL framework shows higher accuracy and speed compared with the previous model.
更多查看译文
关键词
fault,diagnosis,multi-granularity
AI 理解论文
溯源树
样例
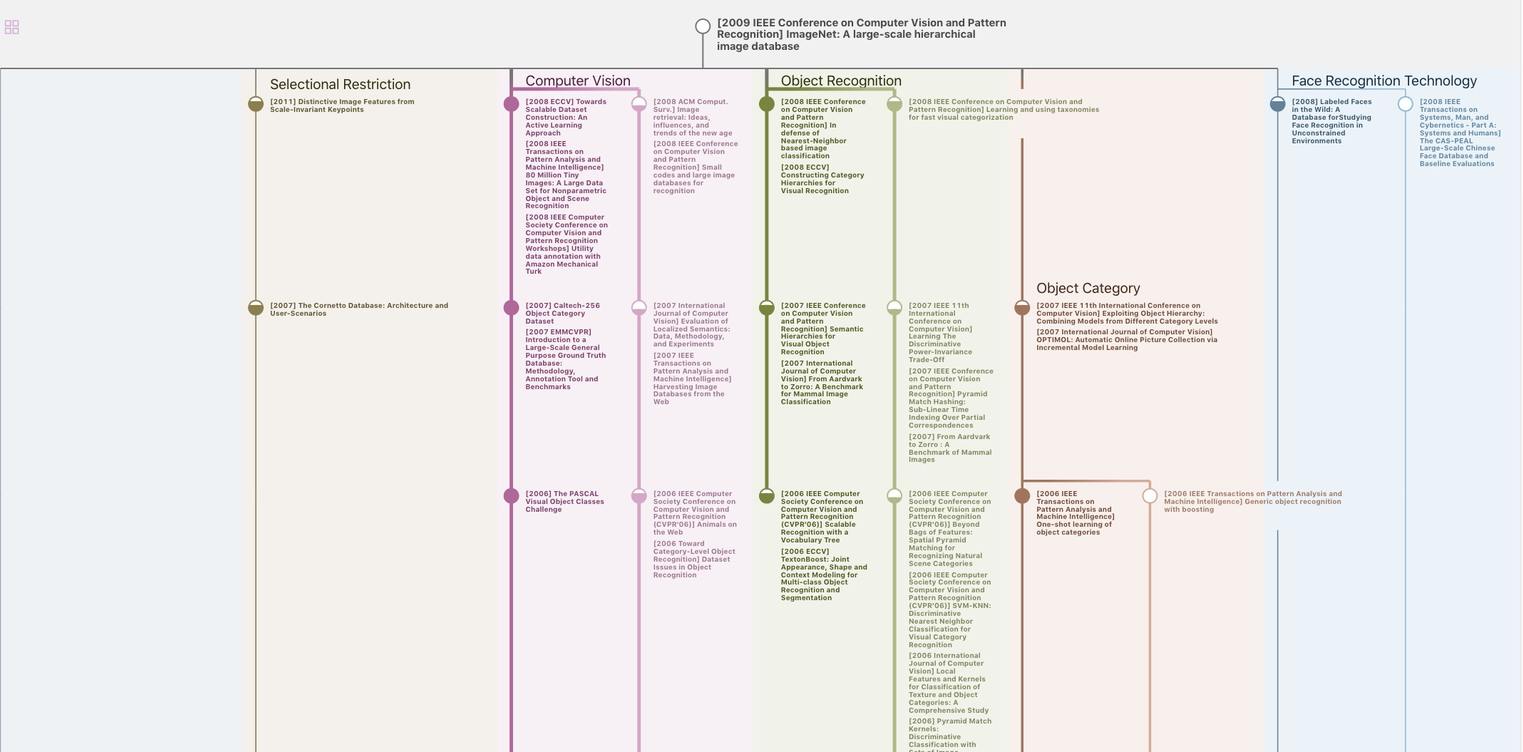
生成溯源树,研究论文发展脉络
Chat Paper
正在生成论文摘要