Enhancing Water Depth Estimation from Satellite Images Using Online Machine Learning: A Case Study Using Baidu Easy-DL with Acoustic Bathymetry and Sentinel-2 Data
Remote Sensing(2023)
摘要
Water depth estimation is paramount in various domains, including navigation, environmental monitoring, and resource management. Traditional depth measurement methods, such as bathymetry, can often be expensive and time-consuming, especially in remote or inaccessible areas. This study delves into the application of machine learning techniques, specifically focusing on the Baidu Easy DL model for water depth estimation leveraging satellite imagery. Utilizing Sentinel-2 satellite data over Rushikonda Beach in India and processing it into remote sensing reflectance using ACOLITE software, this research compares the performance of several machine learning algorithms, including the Stumpf model, Log-Linear model, and the Baidu Easy DL model, for accurate depth estimation. The results indicate that the Easy-DL model outperforms traditional methods, particularly excelling in the 0-11 m depth range. This study showcases the substantial potential of machine learning in remote sensing, offering robust water depth estimates, even in complex coastal environments. Furthermore, it underscores the critical role of comprehensive training datasets and ensemble learning techniques in enhancing accuracy. This research opens avenues for the further exploration of machine learning applications in remote sensing and highlights the promising prospects of online model APIs when streamlining remote sensing data processing.
更多查看译文
关键词
big model,machine learning,Baidu Easy-DL,water depth,satellite-based bathymetry
AI 理解论文
溯源树
样例
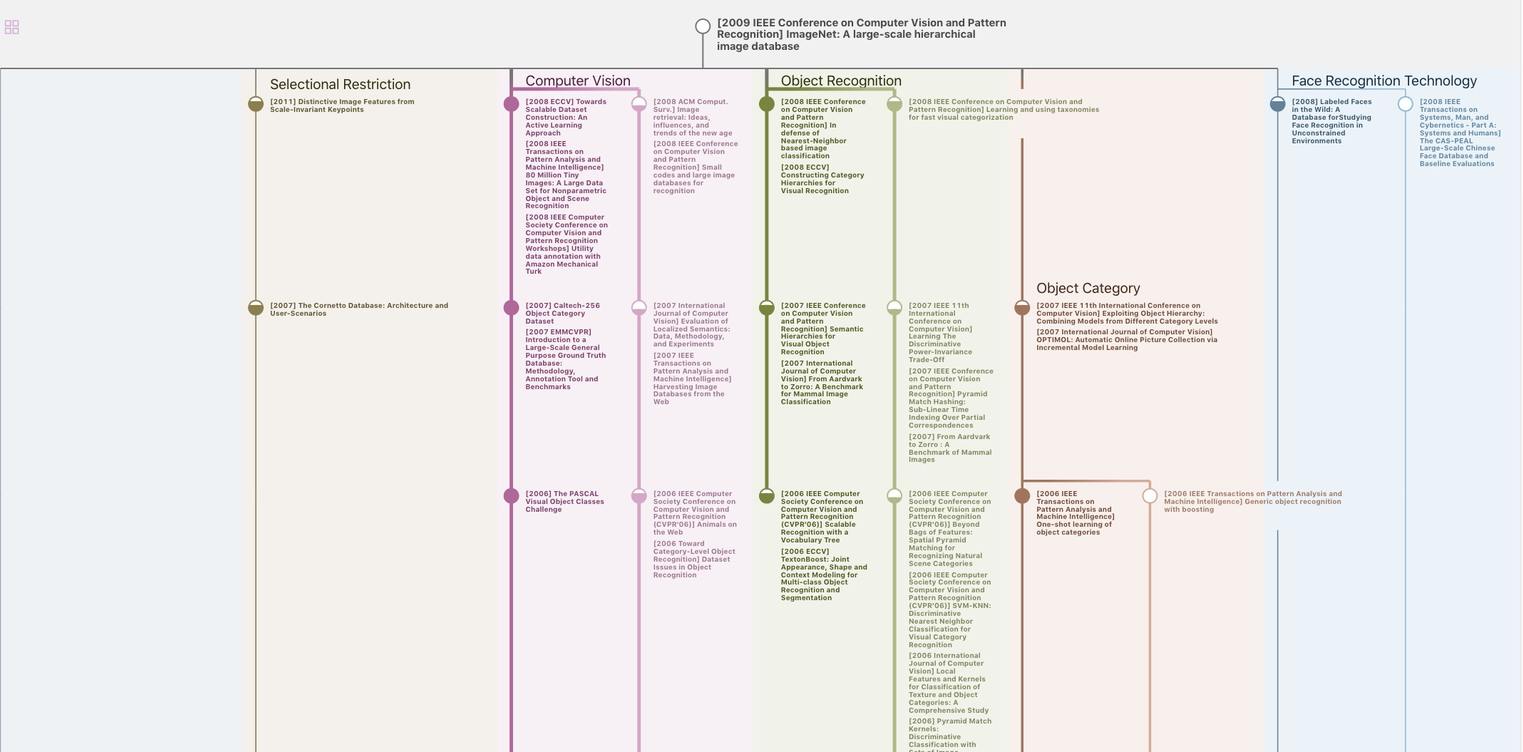
生成溯源树,研究论文发展脉络
Chat Paper
正在生成论文摘要