KORSAL: Key-Point Based Online Real-Time Spatio-Temporal Action Localization
2023 IEEE CANADIAN CONFERENCE ON ELECTRICAL AND COMPUTER ENGINEERING, CCECE(2023)
摘要
Real-time and online action localization in videos poses a critical and formidable challenge. Achieving accurate action localization necessitates the integration of both temporal and spatial information. However, existing approaches rely on computationally intensive 3D convolutional neural network (CNN) architectures or redundant two-stream architectures with optical flow, rendering them unsuitable for real-time, online applications. To address this, we propose a novel approach that leverages fast and efficient key-point-based bounding box prediction for spatial action localization. Additionally, we introduce a tube-linking algorithm that ensures the temporal continuity of action tubes even in the presence of occlusions. By combining temporal and spatial information into a cascaded input for a single network, we eliminate the need for a two-stream architecture, enabling the network to effectively learn from both types of information. Instead of using computationally demanding optical flow, we extract temporal information efficiently using a structural similarity index map. Despite the simplicity of our approach, our lightweight end-to-end architecture achieves state-of-the-art frame mean average precision (mAP) of 74.7% on the challenging UCF101-24 dataset, demonstrating a notable performance gain of 6.4% over previous online methods. Moreover, we achieve state-of-the-art video mAP results compared to both online and offline methods. Furthermore, our model achieves a frame rate of 41.8 FPS (Frames per second), representing a 10.7% improvement over contemporary real-time methods.
更多查看译文
关键词
Action Localization,Spatio-Temporal,Online,Real-time
AI 理解论文
溯源树
样例
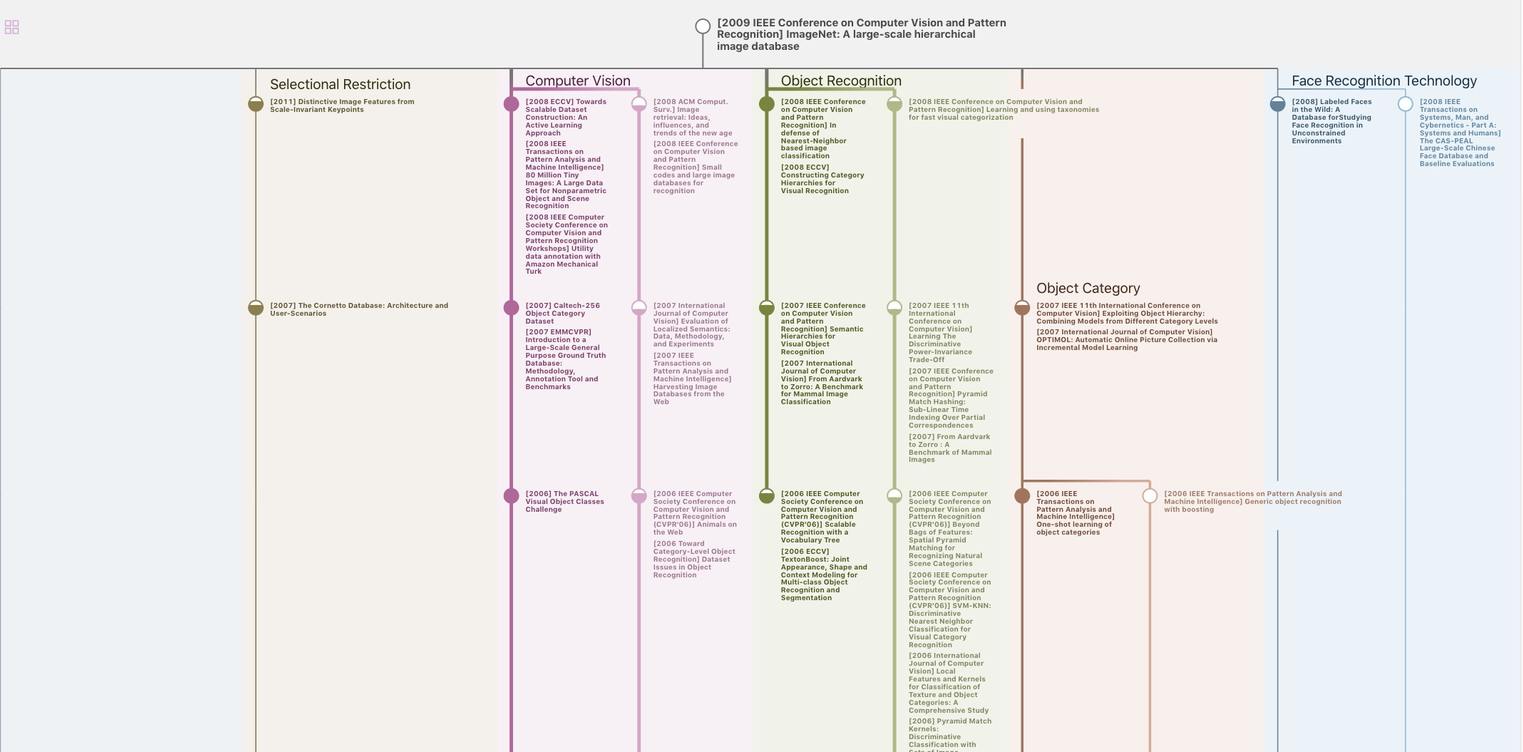
生成溯源树,研究论文发展脉络
Chat Paper
正在生成论文摘要