SFHN: Spatial-Frequency Domain Hybrid Network for Image Super-Resolution
IEEE Transactions on Circuits and Systems for Video Technology(2023)
摘要
Deep convolutional neural networks (CNNs) have demonstrated tremendous success in image super-resolution (SR). According to the frequency principle, the vanilla CNNs fit the target function from low to high frequencies during the training process. It implies an implicit bias that CNNs tend to fit the training data by a low-frequency function. This is detrimental to SR task which essentially recovers the missing high-frequency cues from degraded images. To address this issue, a novel spatial-frequency domain hybrid network (SFHN) is proposed for image SR in this paper. More specifically, it contains multiple spatial-frequency domain hybrid convolution blocks (SFBlocks) for extracting both spatial and frequency components. In particular, frequency information is complementary to spatial one, mitigating the disadvantage of the spatial CNNs in capturing high-frequency content resulting from the inherent bias of the networks. In contrast to convolution blocks in the previous SR methods, the proposed SFBlock is configured with additional frequency-domain convolution branch driven by the Fourier transform, which allows to process frequency content. In addition, we introduce a spectral loss based on Fast Fourier Transform (FFT) to fully leverage the performance of our SFHN, as it prevents the loss of important frequency content during training. Experimental results on public benchmarks demonstrate that our SFHN achieves promising performance superior to the state-of-the-art methods.
更多查看译文
关键词
Single image super-resolution,frequency-domain,hybrid convolution
AI 理解论文
溯源树
样例
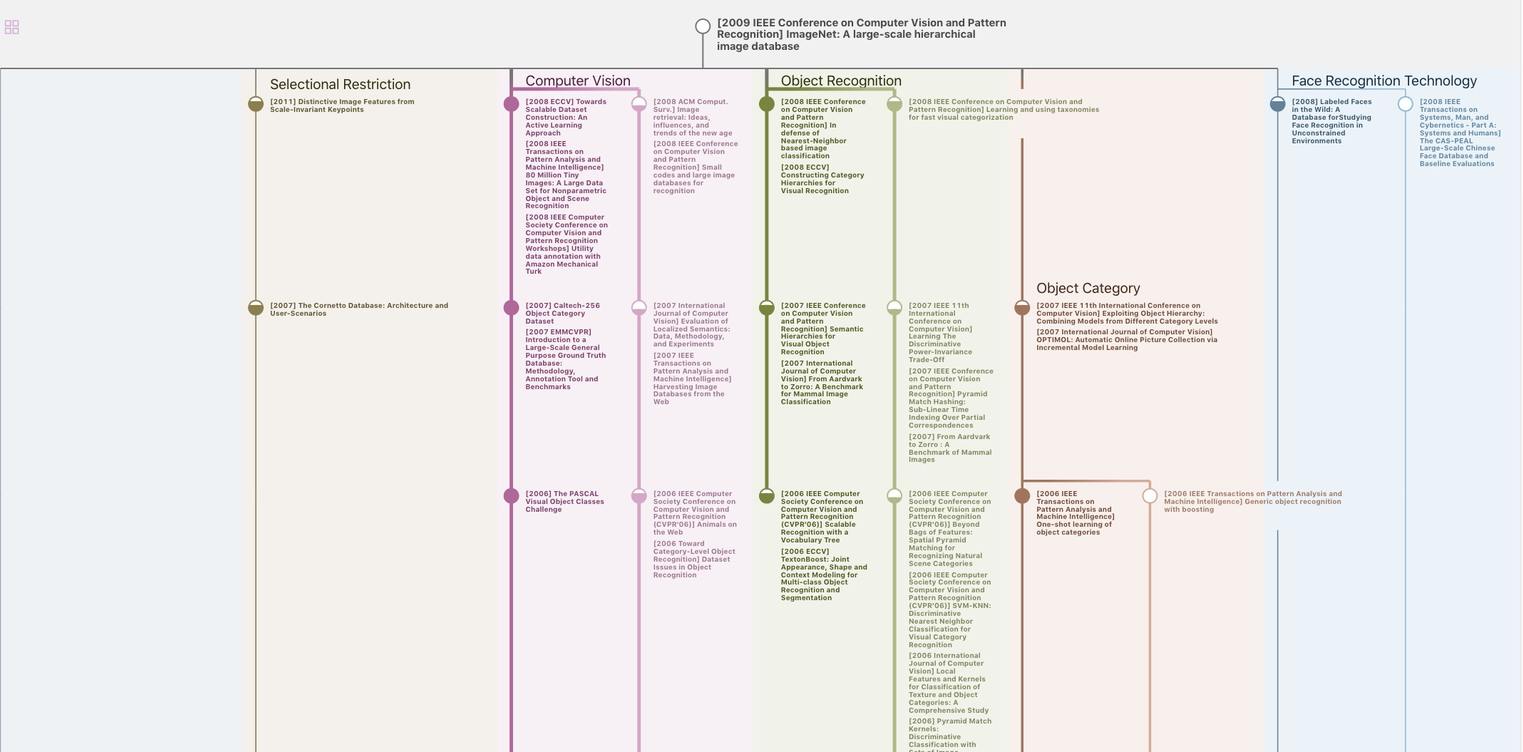
生成溯源树,研究论文发展脉络
Chat Paper
正在生成论文摘要