Tale of Two Cs: Computation vs. Communication Scaling for Future Transformers on Future Hardware
2023 IEEE INTERNATIONAL SYMPOSIUM ON WORKLOAD CHARACTERIZATION, IISWC(2023)
摘要
Scaling neural network models has delivered dramatic quality gains across ML problems. However, this scaling also increased the reliance on efficient distributed training techniques. Accordingly, like other distributed computing scenarios, it is important to understand how compute and communication will scale relative to one another as models scale and hardware evolves? A careful study which answers this question can better guide the design of future systems which can efficiently train future large models. Accordingly, we comprehensively analyze compute vs. communication (Comp-vs.-Comm) scaling for future Transformer models on future hardware, across multiple axes (algorithmic, empirical, hardware evolution). First, our algorithmic analysis shows that compute generally enjoys an edge over communication as models scale. However, these trends are being stressed since device memory capacity scales much slower than model size. We quantify this edge by empirically studying how Comp-vs.-Comm scales for future models on future hardware. To avoid profiling numerous Transformer models across many setups, we extract execution regions and project costs using operator models. This allows a spectrum (hundreds) of future model/hardware scenarios to be accurately studied (< 15% error) and reduces profiling costs by 2100x. Our experiments show that communication will be a significant portion (40-75%) of runtime as models and hardware evolve. Moreover, communication that is often hidden by overlapped computation in today's models cannot be hidden in future, larger models. Overall, this work highlights communication's increasingly large role as models scale, discusses promising techniques to potentially tackle communication, and discusses how our analysis influences their potential improvements.
更多查看译文
关键词
communication scaling,future transformers,hardware
AI 理解论文
溯源树
样例
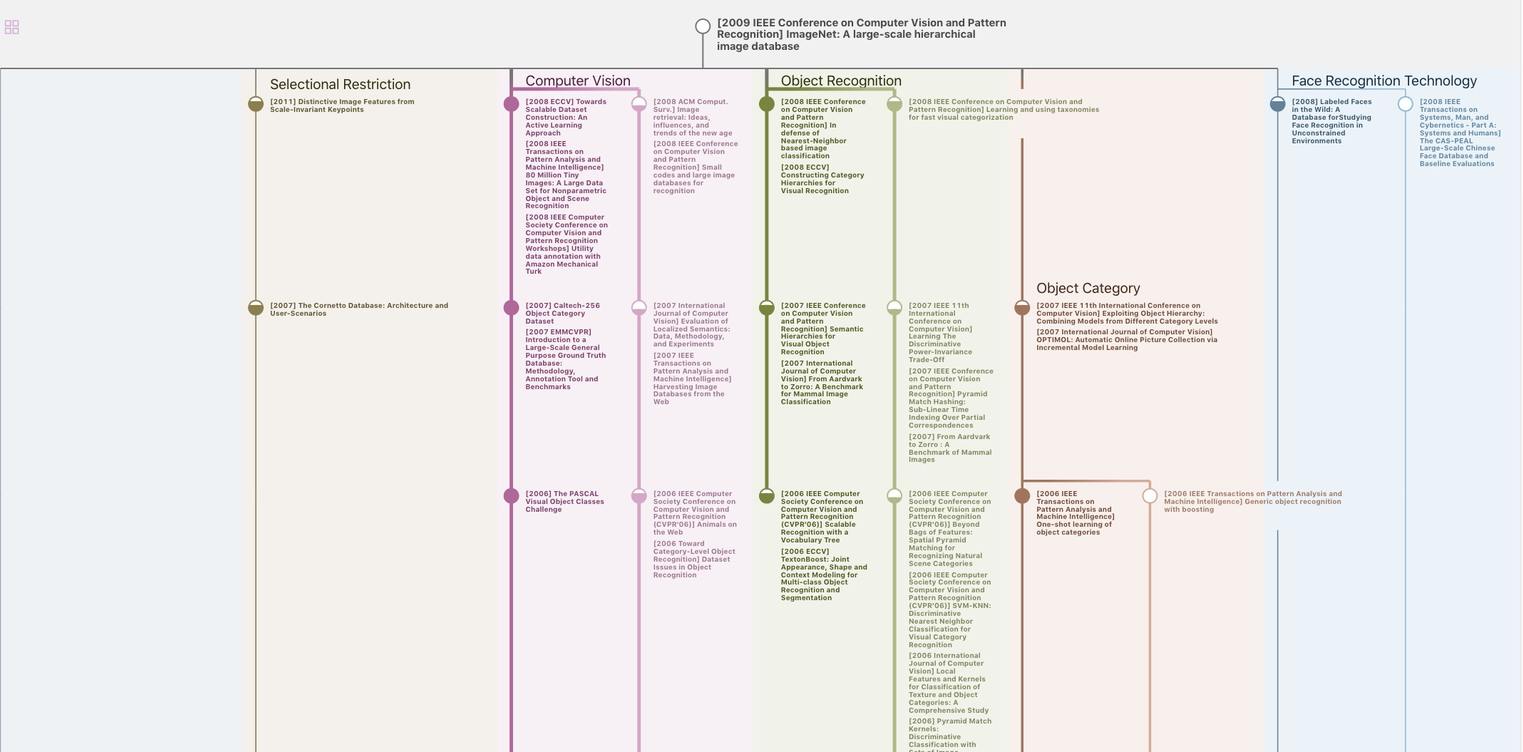
生成溯源树,研究论文发展脉络
Chat Paper
正在生成论文摘要