Cooperative Task Offloading and Service Caching for Digital Twin Edge Networks: A Graph Attention Multi-Agent Reinforcement Learning Approach
IEEE JOURNAL ON SELECTED AREAS IN COMMUNICATIONS(2023)
摘要
Mobile edge computing (MEC) enables various services to be cached in close proximity to the user equipments (UEs), thereby reducing the service delay of many emerging applications. However, the limitation of storage, computation, and radio resources, the dynamics of the decentralized MEC environment, and the complex spatial relationships of service request types and wireless network states between edge nodes make it difficult to realize efficient edge computing services. To address these challenges, this paper integrates the digital twin (DT) technology with a multi-cell MEC network to study an intelligent cooperative task offloading and service caching scheme, aiming at maximizing a quality of services (QoE)-based system utility. Specifically, we first construct a digital twin edge network (DITEN) to reflect the physical MEC system in real-time and provide data for training. With the help of DT technology, it is easy to access data resources in the DITEN to improve the simulation ability and reduce the communication cost. Then, we propose a graph attention-based multi-agent reinforcement learning (GatMARL) algorithm to learn the optimal task offloading and service caching strategies in the DITEN. The GatMARL employs a graph attention-based value decomposition network to capture the potential spatial relationships between edge nodes to learn better attentive cooperation policy. Simulation results demonstrate that the proposed GatMARL algorithm exhibits an effective performance improvement compared with state-of-the-art benchmarks.
更多查看译文
关键词
Edge computing,Mobile edge computing,digital twin,task offloading,service caching,multi-agent reinforcement learning,graph attention network
AI 理解论文
溯源树
样例
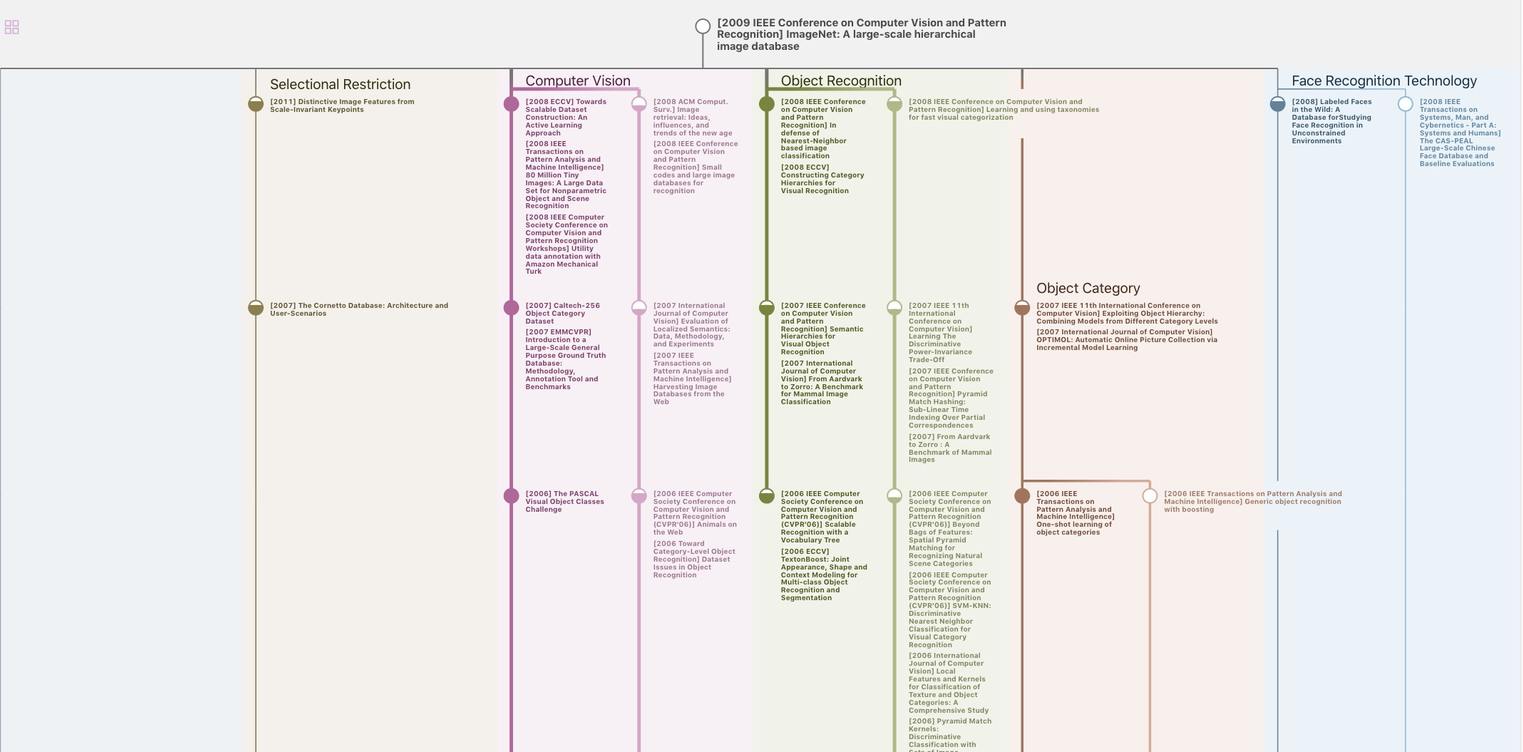
生成溯源树,研究论文发展脉络
Chat Paper
正在生成论文摘要