CoSeNet: A novel approach for optimal segmentation of correlation matrices
DIGITAL SIGNAL PROCESSING(2024)
摘要
In this paper, we propose a novel approach for the optimal identification of correlated segments in noisy correlation matrices. The proposed model is known as CoSeNet (Correlation Segmentation Network) and is based on a four-layer algorithmic architecture that includes several processing layers: input, formatting, re-scaling, and segmentation layer. The proposed model can effectively identify correlated segments in such matrices, better than previous approaches for similar problems. Internally, the proposed model utilizes an overlapping technique and uses pre-trained Machine Learning (ML) algorithms, which makes it robust and generalizable. CoSeNet approach also includes a method that optimizes the parameters of the re-scaling layer using a heuristic algorithm and fitness based on a Window Difference-based metric. The output of the model is a binary noise-free matrix representing optimal segmentation as well as its segmentation points and can be used in a variety of applications, obtaining compromise solutions between efficiency, memory, and speed of the proposed deployment model.
更多查看译文
关键词
Correlation matrices,Segmentation algorithms,Multi-algorithm architecture,Metaheuristic optimization,Machine learning
AI 理解论文
溯源树
样例
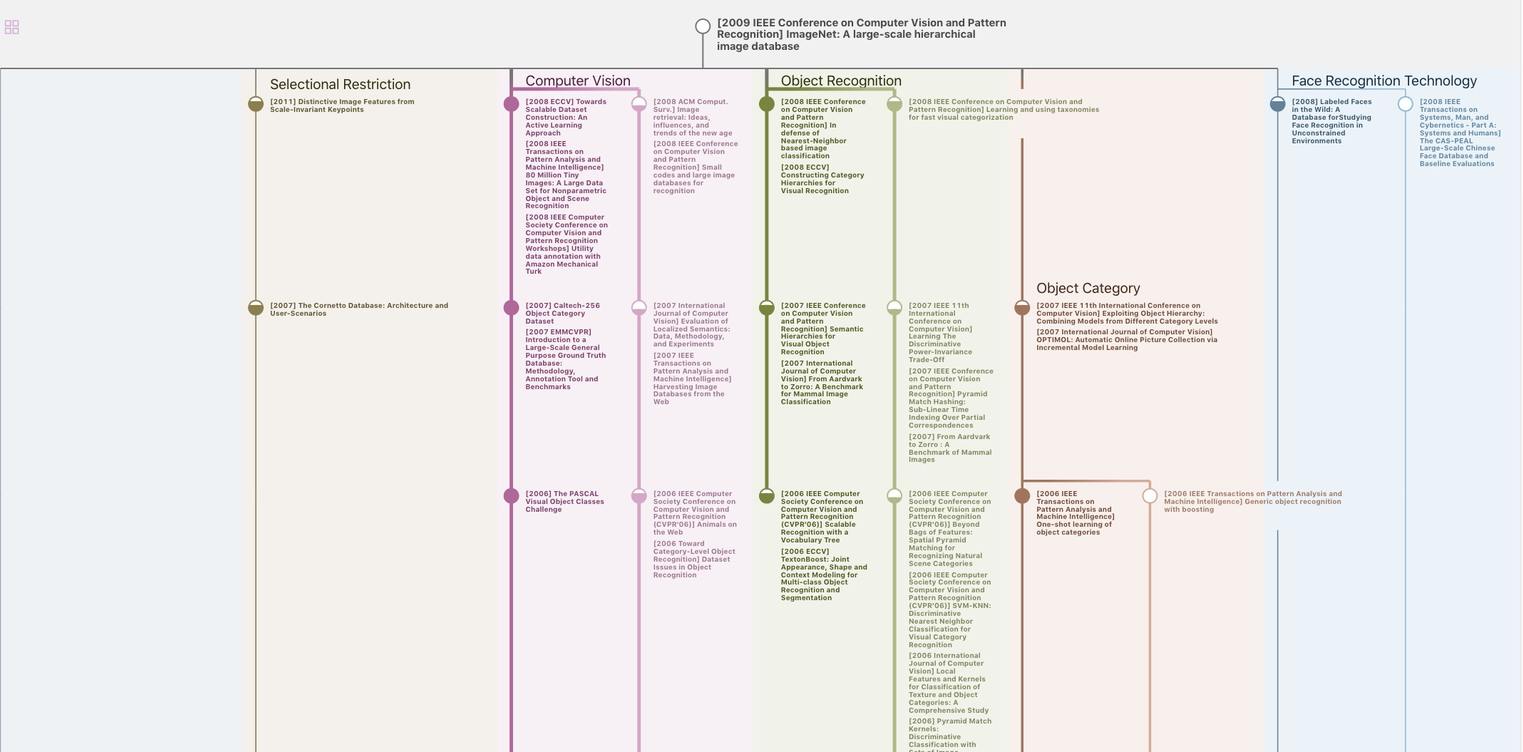
生成溯源树,研究论文发展脉络
Chat Paper
正在生成论文摘要