Automated prediction of acute promyelocytic leukemia from flow cytometry data using a graph neural network pipeline
AMERICAN JOURNAL OF CLINICAL PATHOLOGY(2024)
摘要
Objectives Our study aimed to develop a machine learning (ML) model to accurately classify acute promyelocytic leukemia (APL) from other types of acute myeloid leukemia (other AML) using multicolor flow cytometry (MFC) data. Multicolor flow cytometry is used to determine immunophenotypes that serve as disease signatures for diagnosis.Methods We used a data set of MFC files from 27 patients with APL and 41 patients with other AML, including those with uncommon immunophenotypes. Our ML pipeline involved training a graph neural network (GNN) to output graph-level labels and identifying the most crucial MFC parameters and cells for predictions using an input perturbation method.Results The top-performing GNN achieved 100% accuracy on the training/validation and test sets on classifying APL from other AML and used MFC parameters similarly to expert pathologists. Pipeline performance is amenable to use in a clinical decision support system, and our deep learning architecture readily enables prediction explanations.Conclusions Our ML pipeline shows robust performance on predicting APL and could be used to screen for APL using MFC data. It also allowed for intuitive interrogation of the model's predictions by clinicians.
更多查看译文
关键词
flow cytometry,immunophenotype,graph neural network,machine learning,acute promyelocytic leukemia,acute myeloid leukemia
AI 理解论文
溯源树
样例
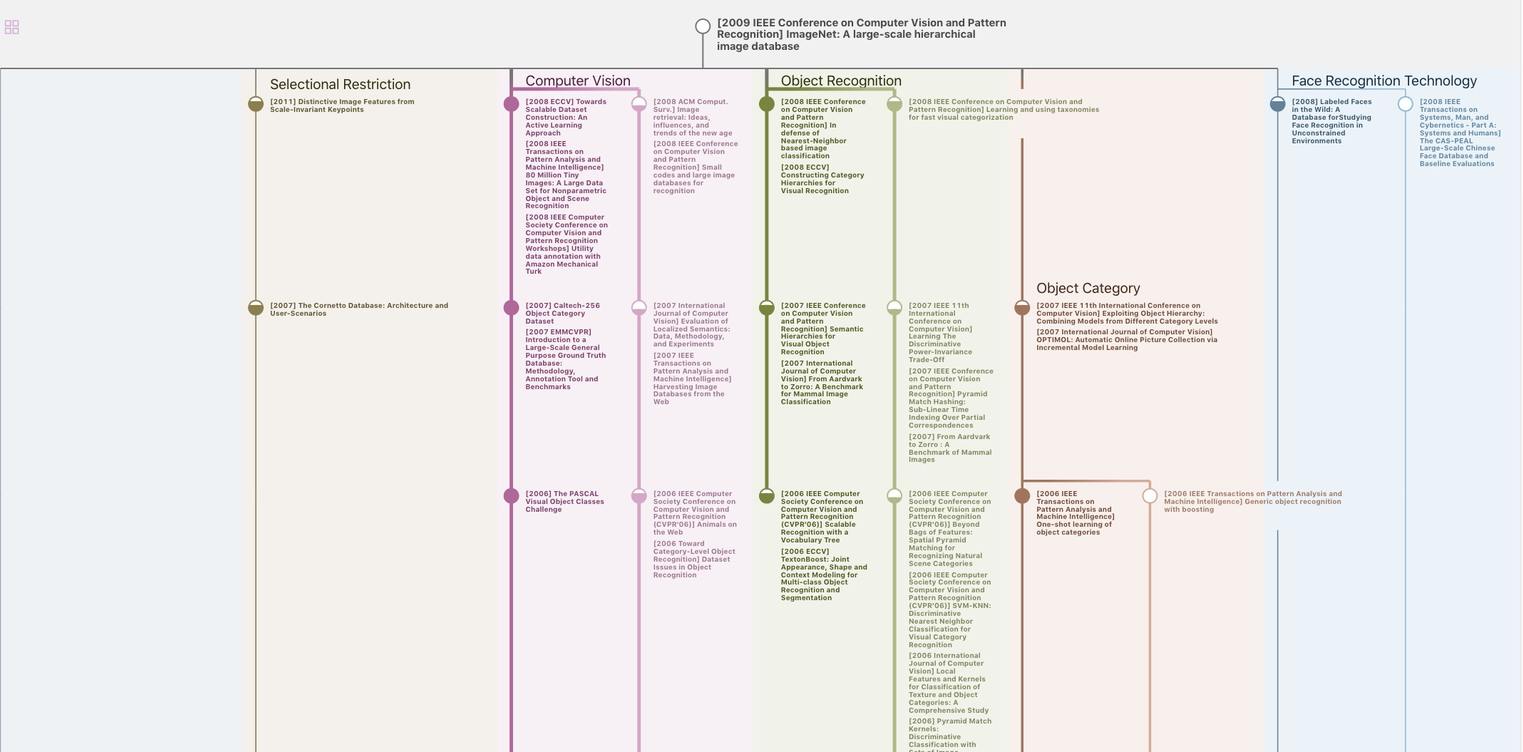
生成溯源树,研究论文发展脉络
Chat Paper
正在生成论文摘要