Interactive Robot Learning from Verbal Correction
CoRR(2023)
摘要
The ability to learn and refine behavior after deployment has become ever more important for robots as we design them to operate in unstructured environments like households. In this work, we design a new learning system based on large language model (LLM), OLAF, that allows everyday users to teach a robot using verbal corrections when the robot makes mistakes, e.g., by saying "Stop what you're doing. You should move closer to the cup." A key feature of OLAF is its ability to update the robot's visuomotor neural policy based on the verbal feedback to avoid repeating mistakes in the future. This is in contrast to existing LLM-based robotic systems, which only follow verbal commands or corrections but not learn from them. We demonstrate the efficacy of our design in experiments where a user teaches a robot to perform long-horizon manipulation tasks both in simulation and on physical hardware, achieving on average 20.0% improvement in policy success rate. Videos and more results are at https://ut-austin-rpl.github.io/olaf/
更多查看译文
关键词
verbal correction,robot,learning,interactive
AI 理解论文
溯源树
样例
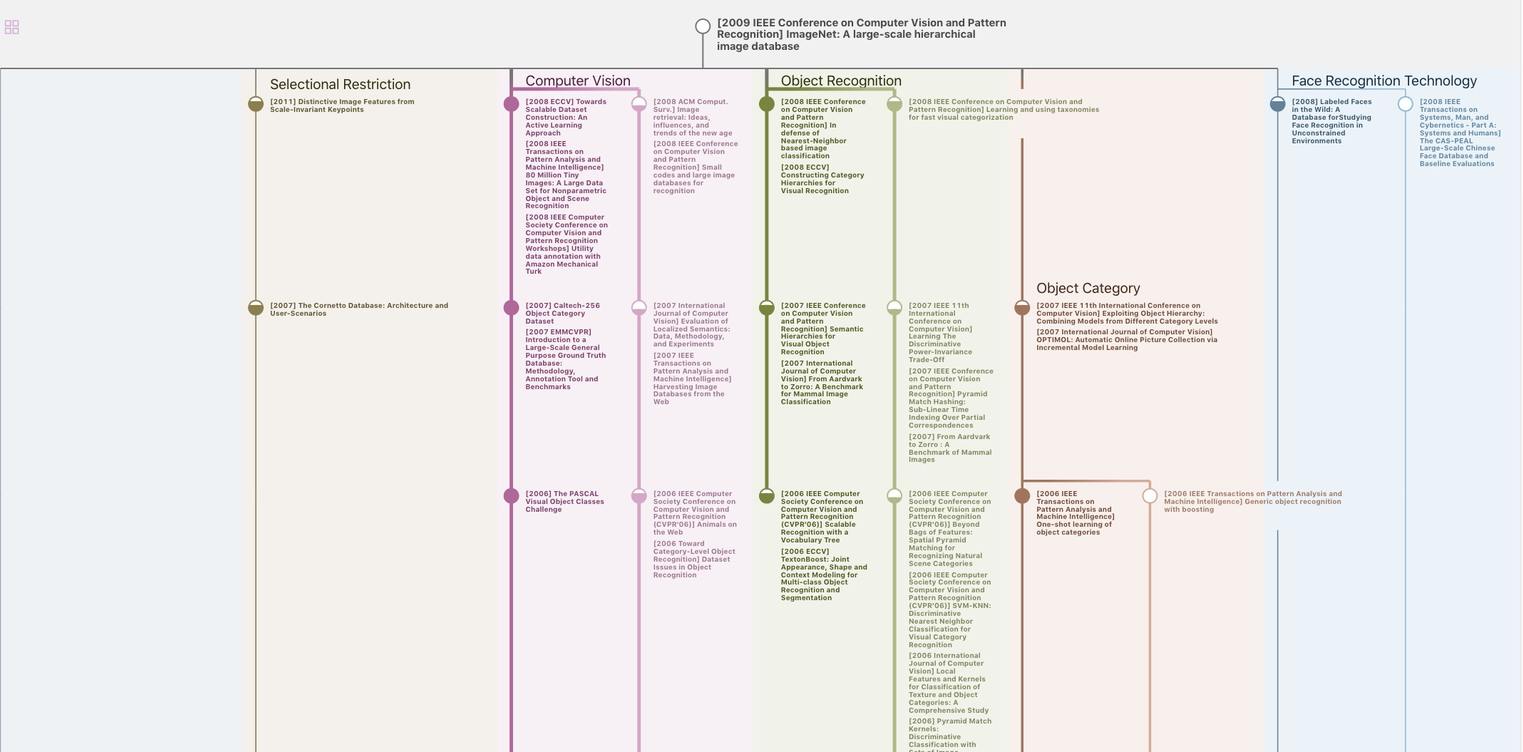
生成溯源树,研究论文发展脉络
Chat Paper
正在生成论文摘要