Multitask Online Learning: Listen to the Neighborhood Buzz
CoRR(2023)
摘要
We study multitask online learning in a setting where agents can only exchange information with their neighbors on an arbitrary communication network. We introduce $\texttt{MT-CO}_2\texttt{OL}$, a decentralized algorithm for this setting whose regret depends on the interplay between the task similarities and the network structure. Our analysis shows that the regret of $\texttt{MT-CO}_2\texttt{OL}$ is never worse (up to constants) than the bound obtained when agents do not share information. On the other hand, our bounds significantly improve when neighboring agents operate on similar tasks. In addition, we prove that our algorithm can be made differentially private with a negligible impact on the regret when the losses are linear. Finally, we provide experimental support for our theory.
更多查看译文
关键词
learning,neighborhood buzz
AI 理解论文
溯源树
样例
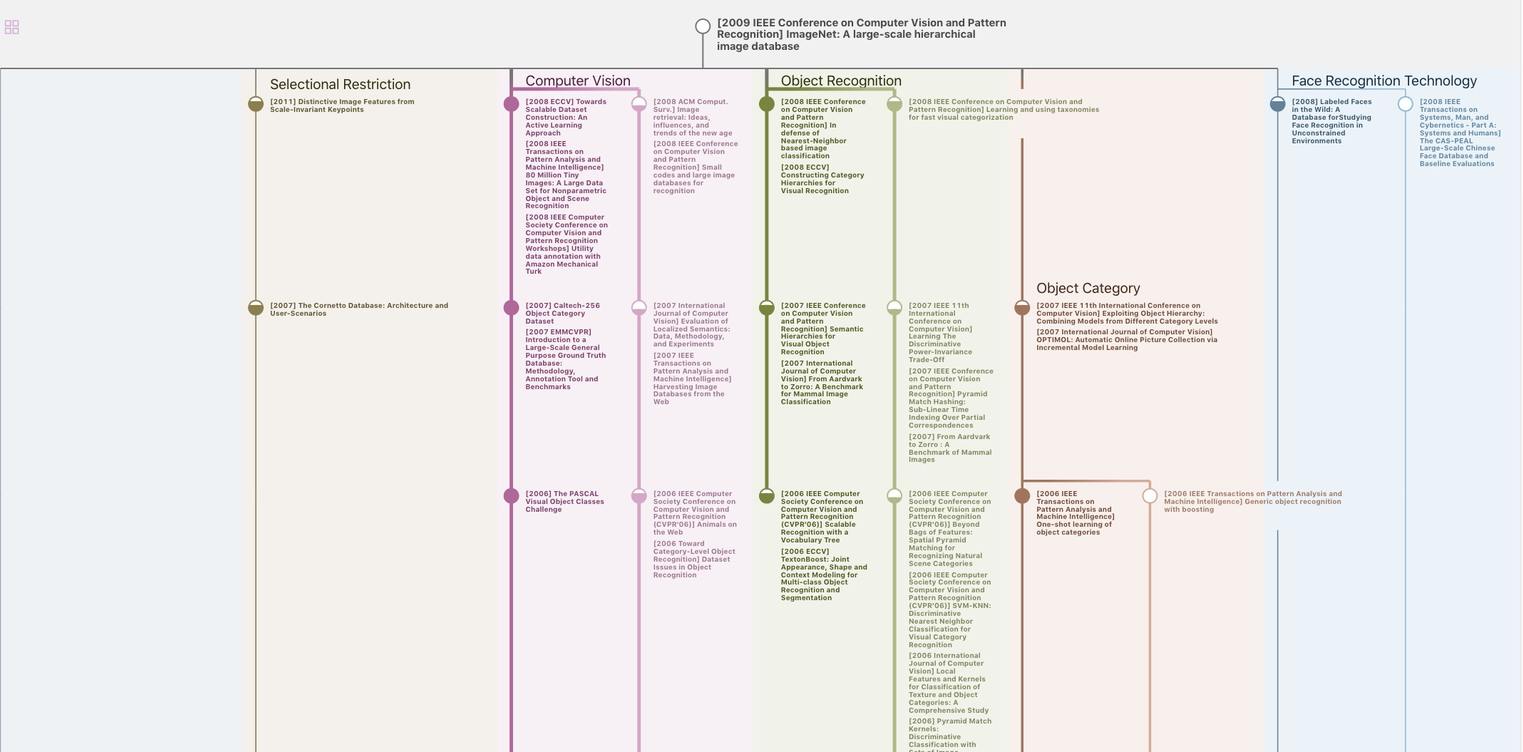
生成溯源树,研究论文发展脉络
Chat Paper
正在生成论文摘要