fairret: a Framework for Differentiable Fairness Regularization Terms
ICLR 2024(2023)
摘要
Current tools for machine learning fairness only admit a limited range of fairness definitions and have seen little integration with automatic differentiation libraries, despite the central role these libraries play in modern machine learning pipelines. We introduce a framework of fairness regularization terms (fairrets) which quantify bias as modular objectives that are easily integrated in automatic differentiation pipelines. By employing a general definition of fairness in terms of linear-fractional statistics, a wide class of fairrets can be computed efficiently. Experiments show the behavior of their gradients and their utility in enforcing fairness with minimal loss of predictive power compared to baselines. Our contribution includes a PyTorch implementation of the fairret framework.
更多查看译文
关键词
fairness,statistics,differentiation,regularization,classification
AI 理解论文
溯源树
样例
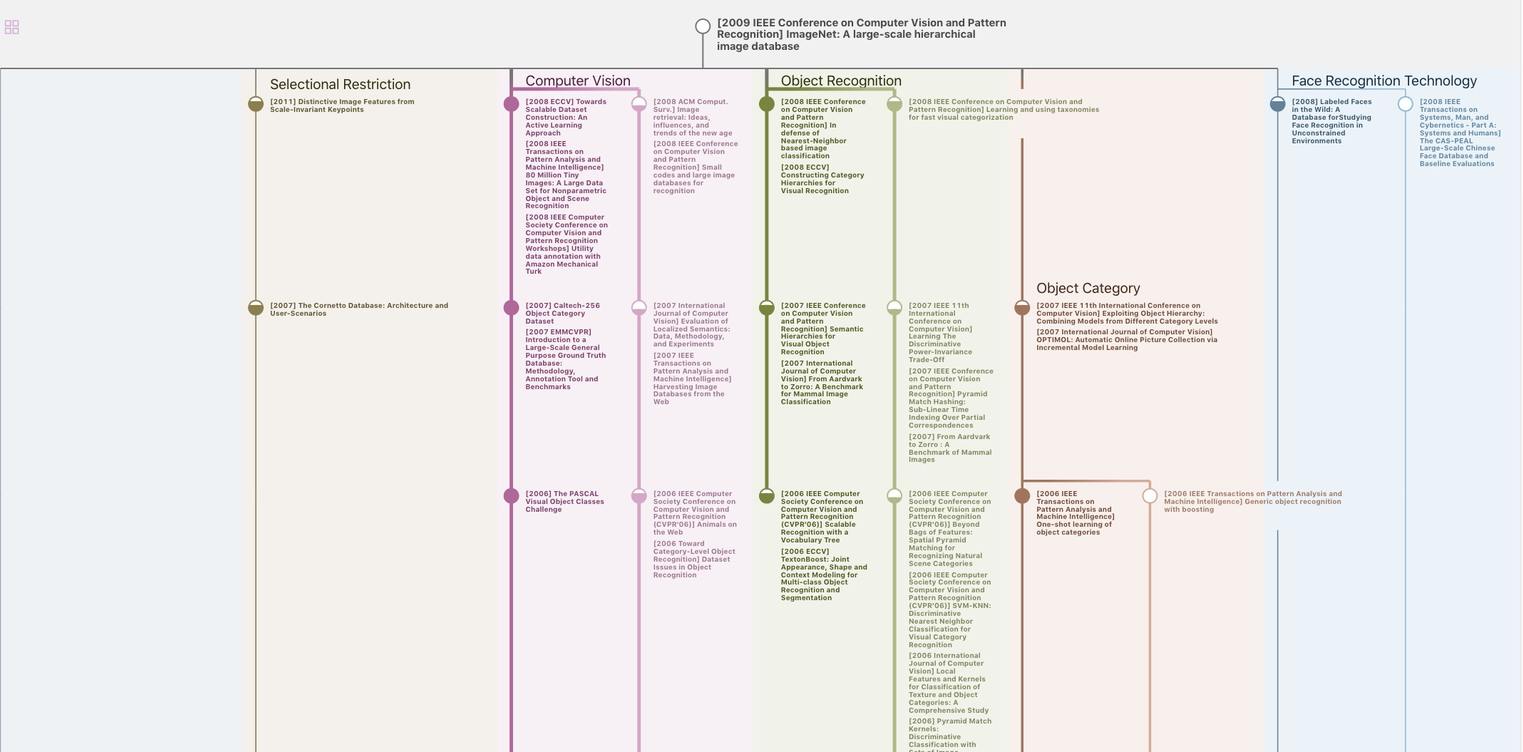
生成溯源树,研究论文发展脉络
Chat Paper
正在生成论文摘要