Privately Aligning Language Models with Reinforcement Learning
arXiv (Cornell University)(2023)
摘要
Positioned between pre-training and user deployment, aligning large language models (LLMs) through reinforcement learning (RL) has emerged as a prevailing strategy for training instruction following-models such as ChatGPT. In this work, we initiate the study of privacy-preserving alignment of LLMs through Differential Privacy (DP) in conjunction with RL. Following the influential work of Ziegler et al. (2020), we study two dominant paradigms: (i) alignment via RL without human in the loop (e.g., positive review generation) and (ii) alignment via RL from human feedback (RLHF) (e.g., summarization in a human-preferred way). We give a new DP framework to achieve alignment via RL, and prove its correctness. Our experimental results validate the effectiveness of our approach, offering competitive utility while ensuring strong privacy protections.
更多查看译文
关键词
language models,reinforcement learning
AI 理解论文
溯源树
样例
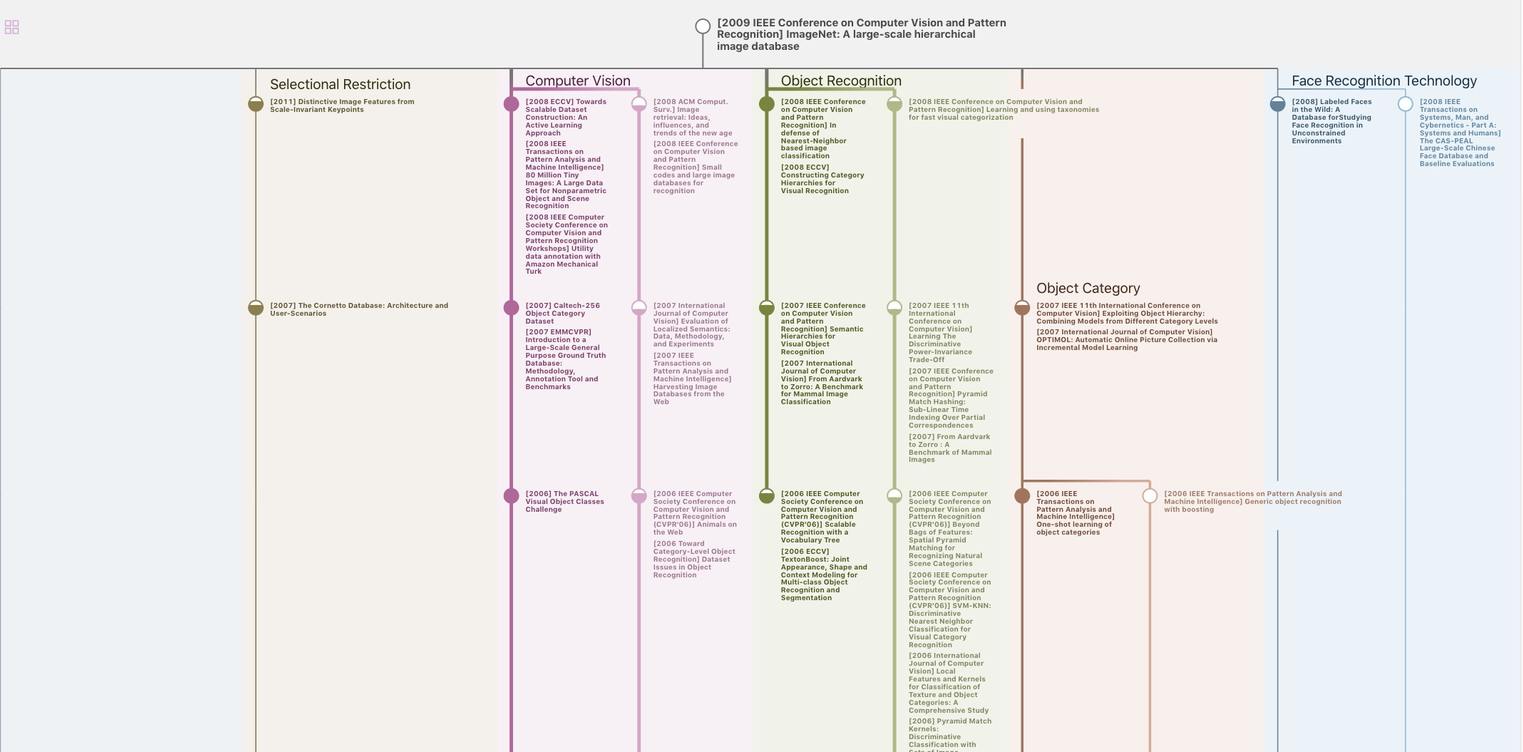
生成溯源树,研究论文发展脉络
Chat Paper
正在生成论文摘要