DA-DRN: A degradation-aware deep Retinex network for low-light image enhancement
DIGITAL SIGNAL PROCESSING(2024)
摘要
This paper proposes a Degradation-Aware Deep Retinex Network (DA-DRN) for enhancing low-light images. DA-DRN is composed of a decomposition U-Net and an enhancement U-Net, and can tackle various degradation issues commonly found in real-world low-light environments, such as low brightness, color distortion, unknown noise, invisible detail, and halo artifacts. To achieve noise removal while preserving details, we introduce a Degradation-Aware Module (DA Module) that guides the decomposer's training process and enables it to be a restorer during training without additional computational cost in the test phase. The DA Module also addresses color distortion and halo artifacts. To train the enhancement network, we use Perceptual Loss to generate brightness-improved illumination maps that are more consistent with human visual perception. Our proposed model is trained and evaluated on the popular LOL real-world and synthetic datasets, as well as several other frequently used datasets without Ground-Truth (LIME, DICM, MEF, and NPE datasets). The results demonstrate that our method achieves promising performance with good robustness and generalization, outperforming many other state-of-the-art methods both qualitatively and quantitatively. Additionally, our method only takes 7 ms to process an image with 600 x 400 resolution on a TITAN Xp GPU.
更多查看译文
关键词
Low-light image enhancement,Retinex decomposition,Image denoising,Color restoration
AI 理解论文
溯源树
样例
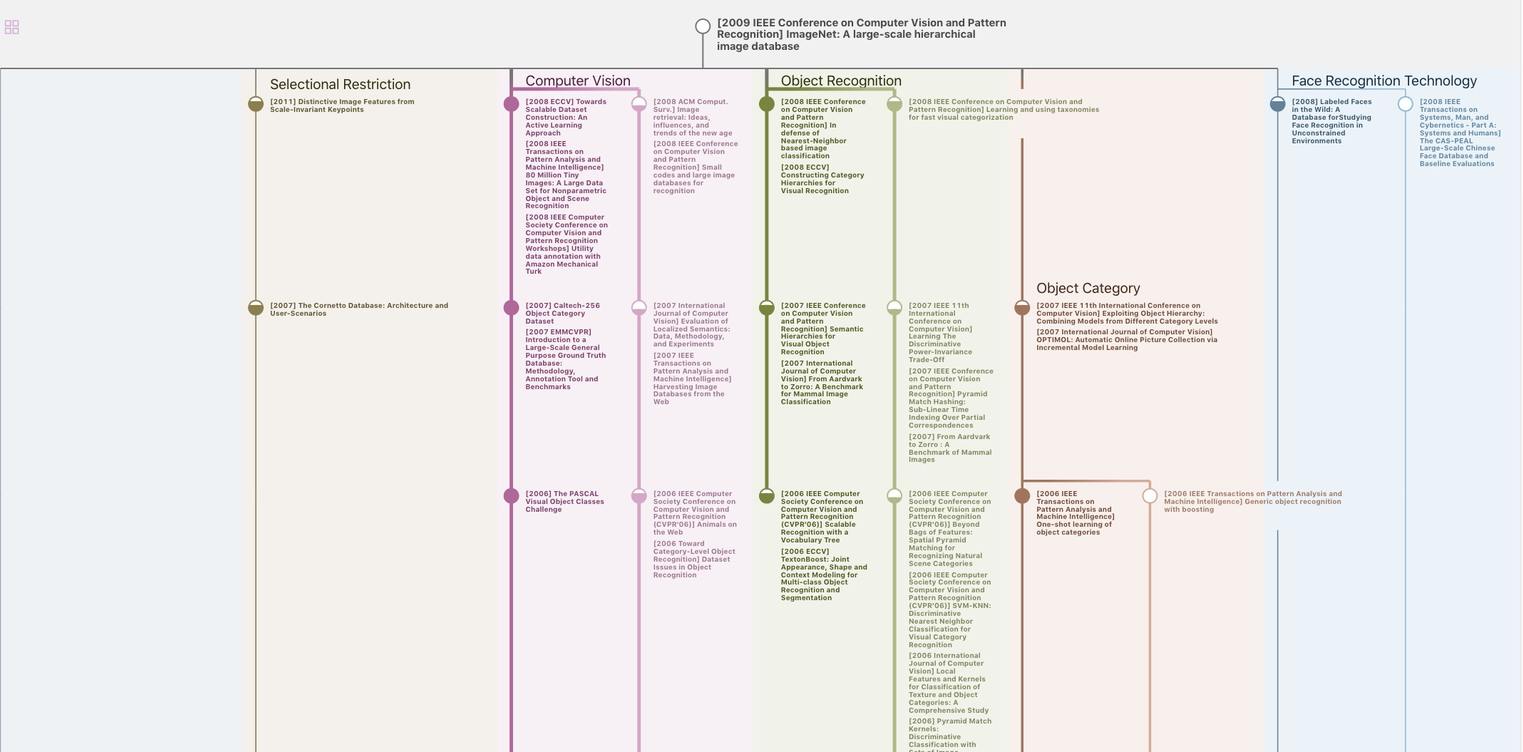
生成溯源树,研究论文发展脉络
Chat Paper
正在生成论文摘要