A zero-shot learning boosting framework via concept-constrained clustering
PATTERN RECOGNITION(2024)
摘要
Zero-shot learning (ZSL) aims to recognize novel classes that have no labeled samples during the training phase, which leads to the domain shift problem. In reality, there exists a large number of compounded unlabeled samples. Therefore, it is crucial to accurately estimate the data distribution of these compounded unlabeled samples and improve the performance of ZSL. This paper proposes a zero-shot learning boosting framework. Specifically, ZSL is transformed into a co-training problem between the data distribution estimation of the unlabeled samples and ZSL. The data distribution estimation is modeled as concept-constrained clustering. Furthermore, we design an alternative optimization strategy to realize mutual guidance between the two processes. Finally, systematic experiments verify the effectiveness of the proposed concept-constrained clustering for alleviating the domain shift problem in ZSL and the universality of the proposed framework for boosting different base ZSL models.
更多查看译文
关键词
Zero-shot learning,Concept-constrained clustering,Co-training,Domain shift
AI 理解论文
溯源树
样例
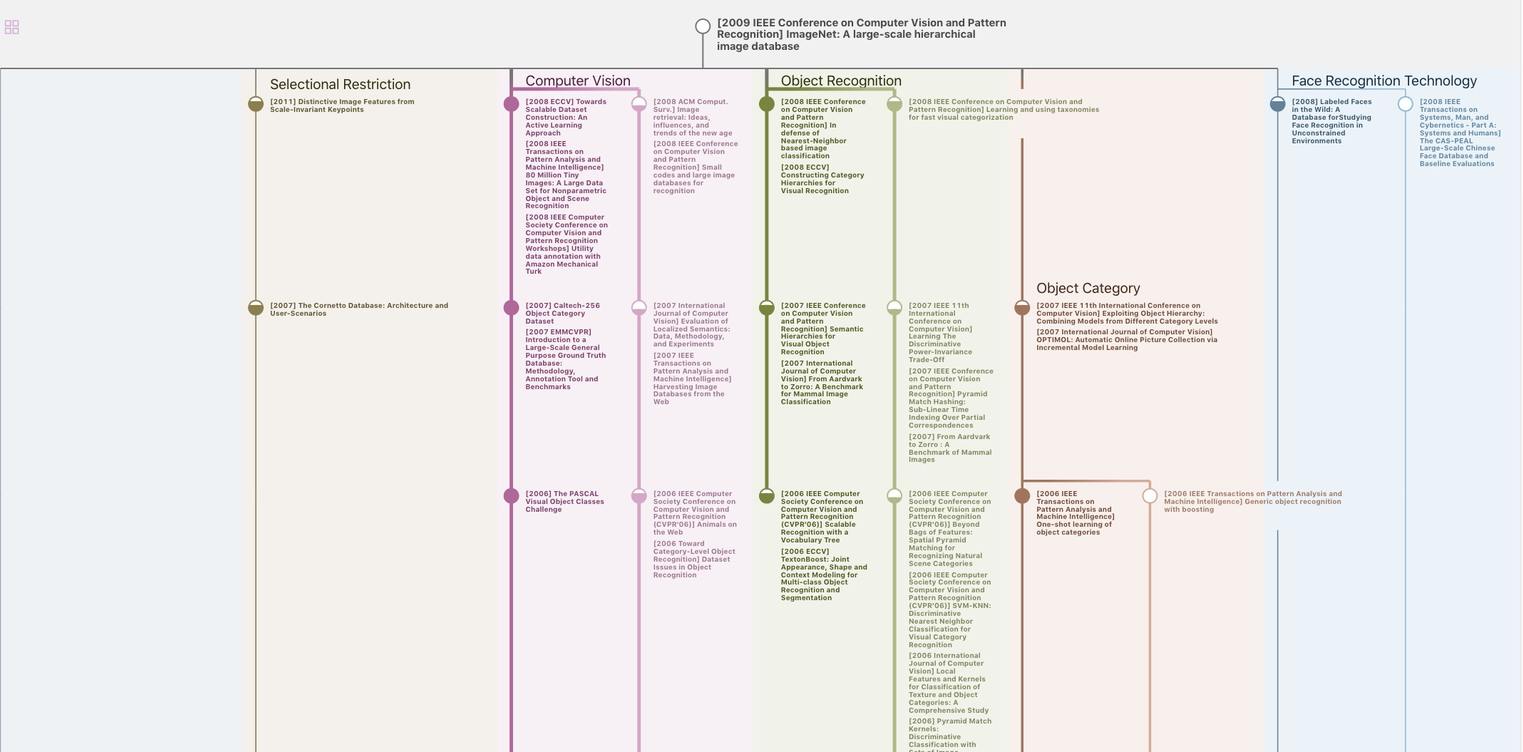
生成溯源树,研究论文发展脉络
Chat Paper
正在生成论文摘要