A data-driven discrete simulation-based optimization algorithm for car-sharing service design
TRANSPORTATION RESEARCH PART B-METHODOLOGICAL(2023)
摘要
This paper formulates a discrete simulation-based optimization (SO) algorithm for a family of large-scale car-sharing service design problems. We focus on the profit-optimal assignment of vehicle fleet across a network of two-way (i.e., round-trip) car-sharing stations. The proposed approach is a metamodel SO approach. A novel metamodel based on a mixed-integer program (MIP) is formulated. The metamodel is embedded within a general-purpose discrete SO algorithm. The proposed algorithm is validated with synthetic toy network experiments. The algorithm is then applied to a high-dimensional Boston case study using reservation data from a major US car-sharing operator. The method is benchmarked versus several algorithms, including stochastic programming. The experiments indicate that the analytical network model information, provided by the MIP to the SO algorithm, is useful both at the first iteration of the algorithm and across subsequent iterations. The solutions derived by the proposed method are benchmarked versus the solution deployed in the field by the car-sharing operator. Via simulation, the proposed solutions improve those deployed with an average improvement of profit of 6% and of vehicle utilization of 3%.The combination of the problem-specific analytical MIP with a general-purpose SO algorithm enables the discrete SO algorithm to: (i) address high-dimensional problems, (ii) become computationally efficient (i.e., it can identify good quality solutions within few simulation observations), (iii) become robust to the quality of the initial points and of the stochasticity of the simulator. More generally, the information provided by the MIP to the SO algorithm enables it to exploit problem-specific structural information. This leads to an algorithm with both asymptotic convergence guarantees as well as good short term performance (i.e., performance given few simulation observations). We view this general idea of combining analytical MIP formulations with general-purpose SO algorithms, or more broadly with general-purpose sampling strategies of high-resolution data, as an innovative and promising area of future research.
更多查看译文
关键词
Discrete simulation-based optimization,Metamodel,Large-scale car-sharing fleet allocation
AI 理解论文
溯源树
样例
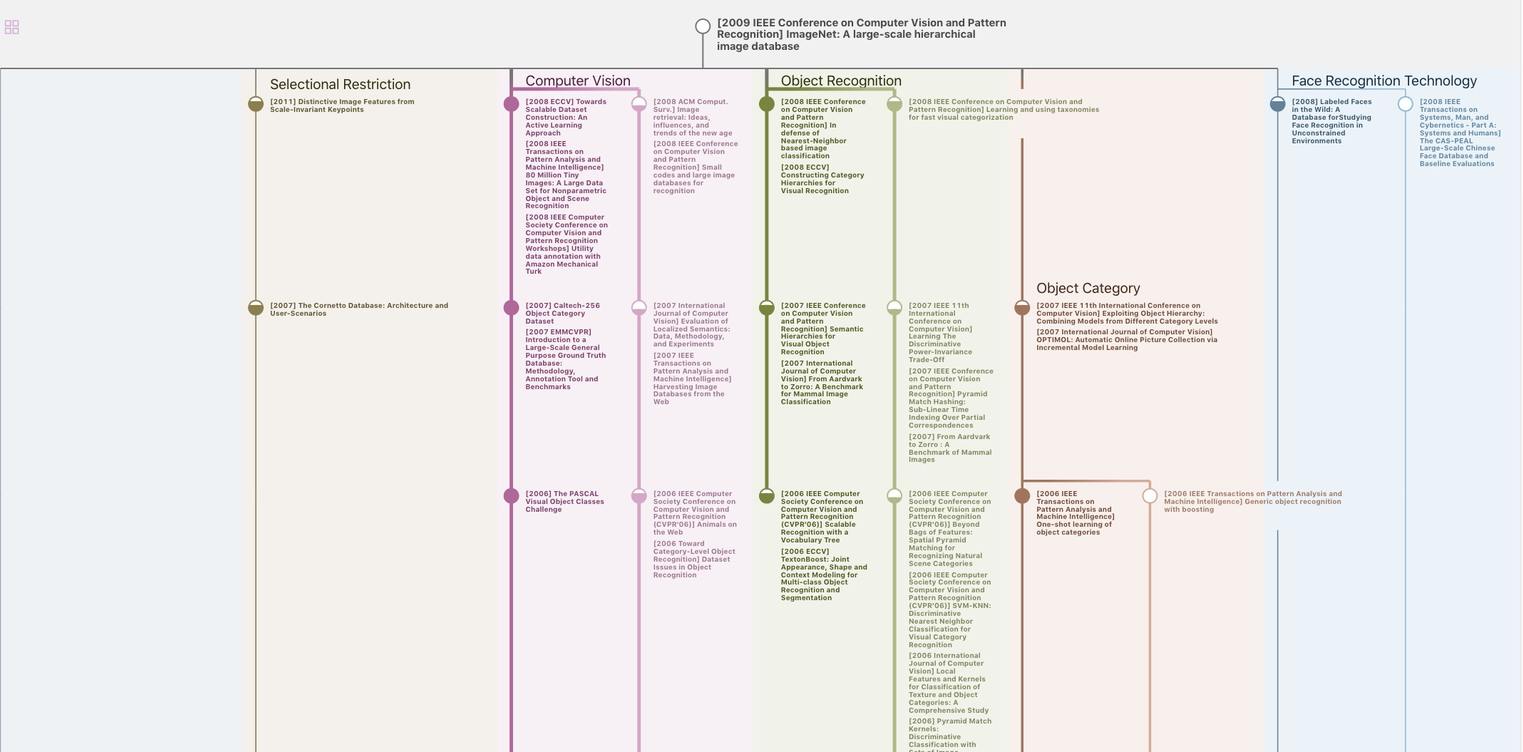
生成溯源树,研究论文发展脉络
Chat Paper
正在生成论文摘要