Subspace segmentation based co-evolutionary algorithm for balancing convergence and diversity in many-objective optimization
SWARM AND EVOLUTIONARY COMPUTATION(2023)
摘要
With the increase of the objective dimension of optimization problems, the effect of comparing individuals through Pareto dominance relation drops sharply. While some algorithms enhance Pareto dominance via diversity preservation strategies, performance indicators, and reference vectors, many of them encounter difficulties in balancing the convergence and diversity of populations. Therefore, this paper proposes a subspace segmentation based co-evolutionary algorithm for balancing convergence and diversity in many-objective optimization. First, the decision space is divided into a convergence subspace and a diversity subspace, which are searched in the early and late stages to improve the population convergence and diversity, respectively. Second, a capacity adaptively adjusted archive is used to retain elite individuals with better convergence in the population, which is further used to mate with the population. Moreover, an indicator with penalty factor is proposed to retain the boundary individuals so as to maintain the population diversity. Comparing with 6 advanced many-objective evolutionary algorithms on 63 benchmark cases, the proposed algorithm obtains smallest IGD on 36 benchmark cases, the experimental results show that the proposed algorithm can balance convergence and diversity well and has exhibit competitiveness.
更多查看译文
关键词
Many-objective optimization,Subspace segmentation,Co-evolution,Archive
AI 理解论文
溯源树
样例
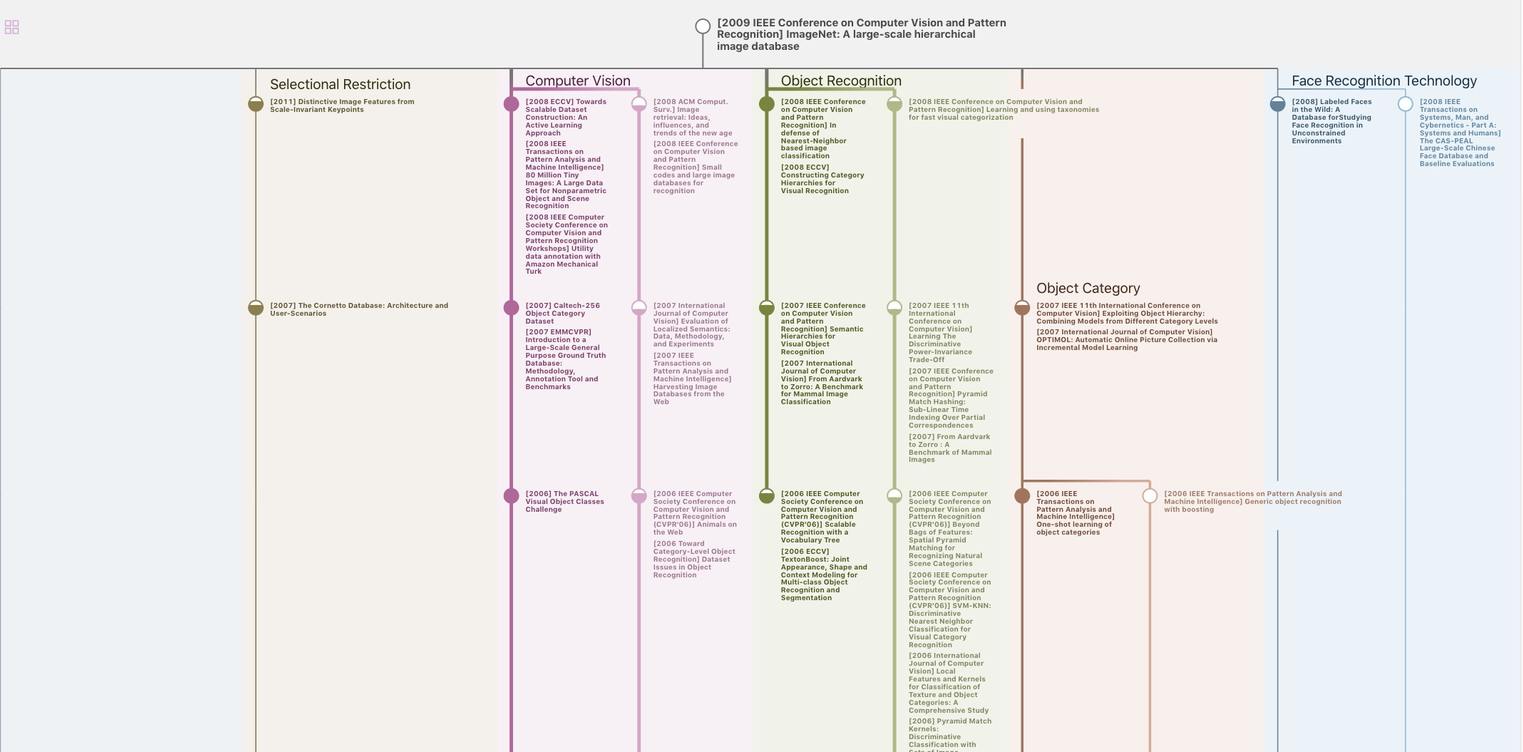
生成溯源树,研究论文发展脉络
Chat Paper
正在生成论文摘要