MCA-DTCN: A novel dual-task temporal convolutional network with multi-channel attention for first prediction time detection and remaining useful life prediction
RELIABILITY ENGINEERING & SYSTEM SAFETY(2024)
摘要
First prediction time (FPT) detection is a significant task when conducting remaining useful life (RUL) prediction for mechanical equipment. Nevertheless, many existing works conducts these two tasks separately, resulting in ignoring the relationships between FPT and RUL. To address the issue, a novel dual-task temporal convolution neural network with multi-channel attention (MCA-DTCN) is proposed to integrate FPT detection and RUL prediction into one framework for making the monitoring more sensitive to healthy stage and deterioration stage. First, MCA-TCN is designed as the feature extractor to extract representative degradation features from multi-dimensional time-series monitoring data. The introduction of MCAs allows MCA-TCN to automatically highlight both usefulness monitoring parameters and degradation features. Second, a novel dual-task learning mechanism is developed to accomplish FPT detection and RUL prediction in parallel, in order to complement each other to achieve better maintenance decision-making. The dual-task learning mechanism consists of two subnetworks, i.e., a classification subnetwork is used to detect the FPT and a regression subnetwork is used to predict the RUL, and they are jointly trained by optimizing a novel fusion loss function. Finally, the outstanding performance of MCA-DTCN is validated through a series of experiments on a public C-MAPSS dataset.
更多查看译文
关键词
Deep learning,Attention mechanism,Temporal convolutional network (TCN),Remaining useful life (RUL),First prediction time (FPT)
AI 理解论文
溯源树
样例
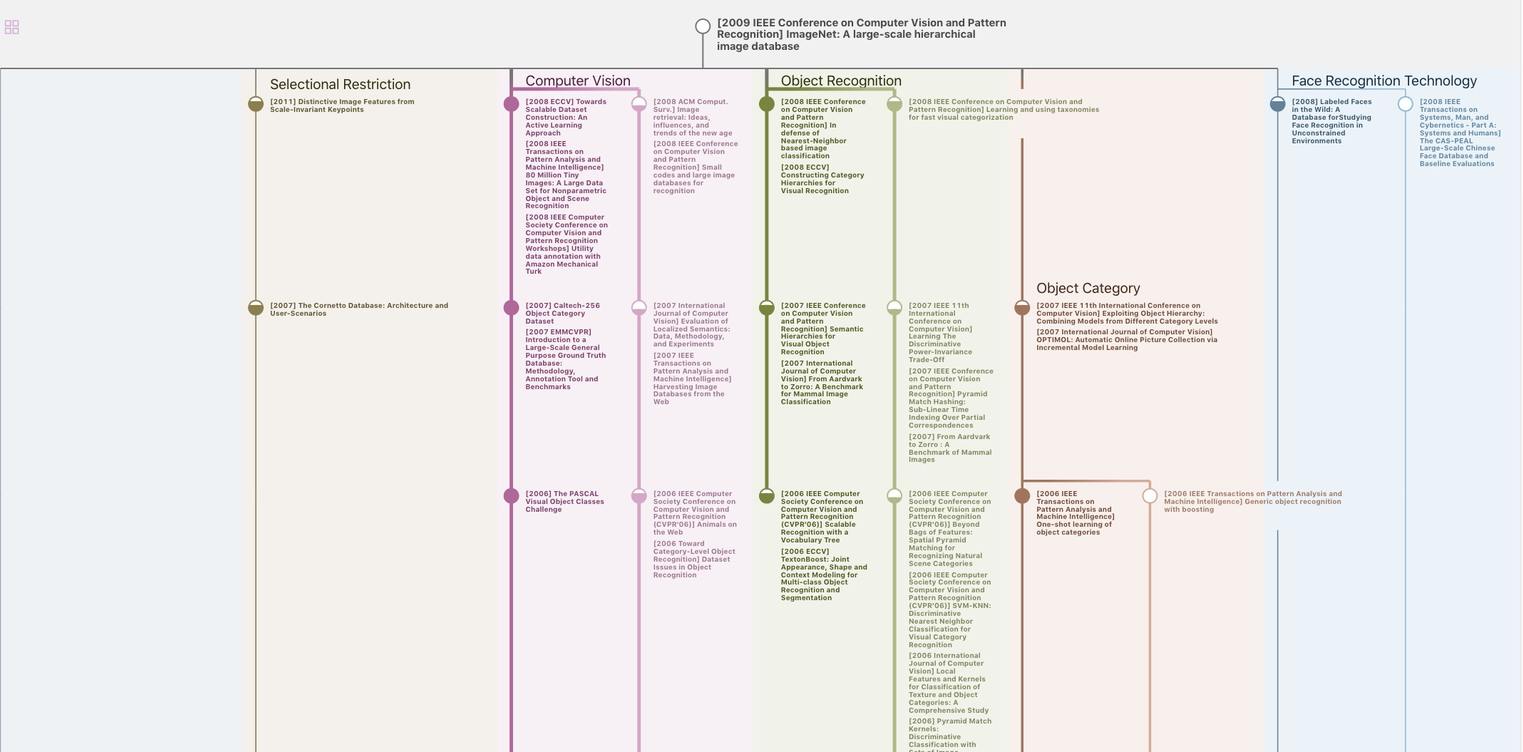
生成溯源树,研究论文发展脉络
Chat Paper
正在生成论文摘要