Constrained Bayesian optimization algorithms for estimating design points in structural reliability analysis
RELIABILITY ENGINEERING & SYSTEM SAFETY(2024)
摘要
Estimating the design points with high accuracy is a historical and key issue for many reliability analysis and reliability-based design optimization methods. Indeed, it is still a challenge especially when the limit state functions (LSFs) show highly nonlinear behaviors, and/or the reliability index is large, and/or the gradients of LSF are not available. To fill the above gap, two acquisition functions incorporating both the objective function and constraints are devised, and based on which, a Constrained Bayesian Optimization (ConBayOpt) method is firstly developed for actively learning the design points with high accuracy and global convergence. Further, an improved algorithm, called Constrained Bayesian Subset Optimization (ConBaySubOpt) is devised for adaptively learning the design points far away from the origin of the standard normal space. Similar to subset simulation, the ConBaySubOpt algorithm automatically produces a set of intermediate failure surfaces and feasible regions for approaching the true design point, but does not require Markov Chain Monte Carlo simulation for conditional sampling. The efficiency, accuracy and wide applicability of the proposed methods are demonstrated with two test examples and three engineering examples.
更多查看译文
关键词
Bayesian optimization,Design point,Acquisition function,Gaussian process regression,Feasible regions
AI 理解论文
溯源树
样例
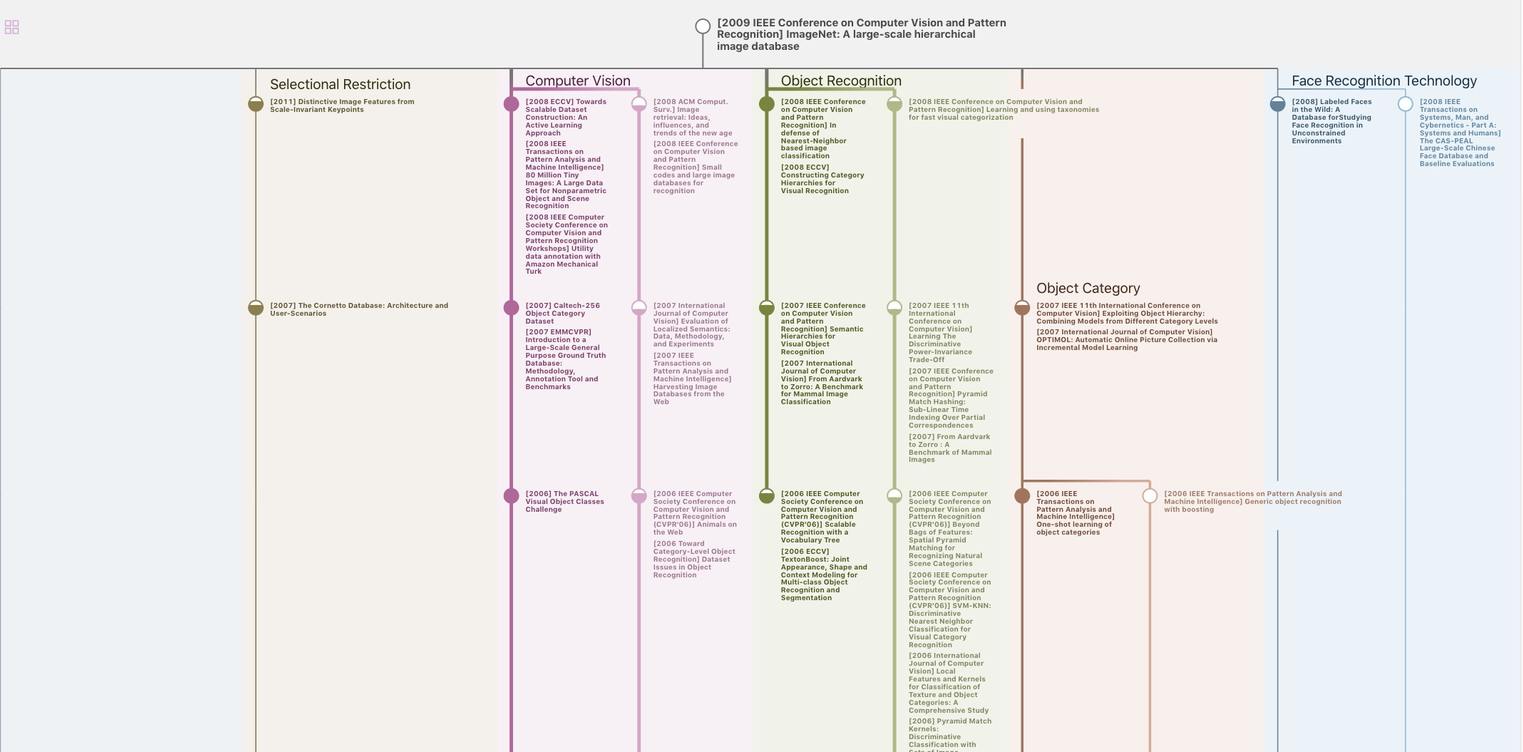
生成溯源树,研究论文发展脉络
Chat Paper
正在生成论文摘要