Detection of Karenia brevis red tides on the West Florida Shelf using VIIRS observations: Accounting for spatial coherence with artificial intelligence
REMOTE SENSING OF ENVIRONMENT(2023)
摘要
Harmful algal blooms (HABs) of the toxic dinoflagellate Karenia brevis (K. brevis) occur annually on the West Florida Shelf (WFS). Detection of these blooms using satellite observations often suffers from two problems: lack of accurate algorithms to identify phytoplankton blooms in optically complex waters and patchiness (i.e., heterogeneity) of K. brevis during blooms. Here, using data collected by the Visible Infrared Imaging Radiometer Suite (VIIRS) on the Suomi National Polar-orbiting Partnership (SNPP) between 2017 and 2019, we develop a practical approach to overcome these difficulties despite the lack of a chlorophyll-a fluorescence band on VIIRS. The approach is based on artificial intelligence (specifically, a deep-learning (DL) convolutional neural network model), which uses spatial coherence of bloom patches to account for the patchiness of K. brevis concentrations. After proper training, the overall performance (i.e., F1 score) of the deep learning model is 89%. Extracted K. brevis patches were consistent with those derived from the Moderate Resolution Imaging Spectroradiometer (MODIS) on the Aqua satellite, which has a fluorescence band. Furthermore, the wider swath of VIIRS over MODIS (3040-km versus 2330-km) led to more valid observations of bloom extent, enabling improved near-realtime applications. The results not only demonstrate the capacity of VIIRS in HABs monitoring, but also show the value of the DL model in extracting K. brevis bloom patches for both near real-time applications and retrospective analysis.
更多查看译文
关键词
Karenia brevis,Red tide,Bloom detection,Deep learning,Remote sensing,VIIRS,MODIS
AI 理解论文
溯源树
样例
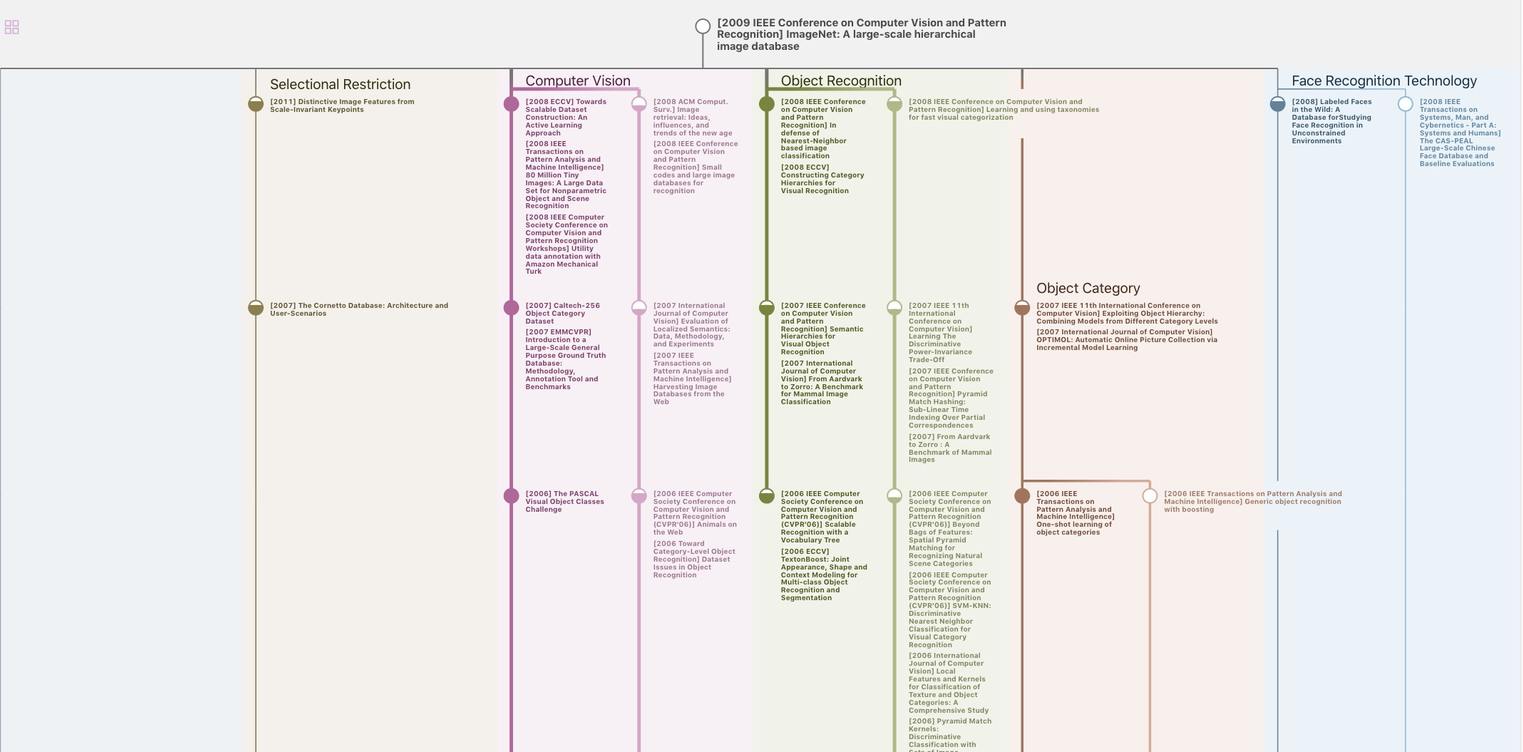
生成溯源树,研究论文发展脉络
Chat Paper
正在生成论文摘要