Automated detection of cough and throat clearing events using an artificial intelligence deep learning model
CHEST(2023)
摘要
SESSION TITLE: Cough: Nothing to Sneeze About SESSION TYPE: Rapid Fire Original Inv PRESENTED ON: 10/10/2023 12:00 pm - 12:45 pm PURPOSE: Evaluation of the severity of refractory chronic cough (RCC) is challenging and largely subjective. Objective assessment of RCC severity, as well as laryngeal injurious behavior (such as throat clearing) is important for management. Artificial intelligence (AI)/machine learning models can be trained to accurately recognize the acoustic signatures of throat clearing and chronic cough. We present preliminary findings from a deep learning model designed to detect throat clearing and chronic cough. METHODS: We obtained 24-hour audio recordings using a lapel microphone from participants in the MACS-1 &2 trials (NCT05110144, NCT05120934) using commercially available digital voice recorders (EVIDA V618 & Philips LFH0662/40). Two providers independently analyzed recordings and annotated cough and throat clearing events using Label Studio Software (https://labelstud.io). Discrepant annotations were jointly reviewed to reach consensus. An independent third reviewer reviewed a random sample of annotations to ensure data accuracy and resolved any discrepant annotations. Spectrograms (time-frequency maps) were generated for each annotated cough and throat clearing. These were matched 1:1 with non-cough and non-throat clearing sounds from the same recordings. A convoluted neural network was used to train the model with mfcc (Mel-frequency cepstrum) with 80% of the data used for training, 10% for validation and 10% as a test set RESULTS: A total of 348 hours of recordings were manually analyzed. A total of 10,662 individual cough and 2,055 throat clearing events were identified. These were matched to 12,717 non-cough events. The deep learning model showed an accuracy, precision, recall and f1 score of 0.901, 0.901, 0.892 and 0.901, respectively. CONCLUSIONS: We report data showing that a deep learning model can be trained to detect both cough and throat clearing events with good accuracy. Further refinement and development of this model is in progress. CLINICAL IMPLICATIONS: This tool has the potential for use in clinical practice in the future after further development and refinement DISCLOSURES: No relevant relationships by Joshika Agarwal No relevant relationships by Vibhor Ahluwalia No relevant relationships by Vaibhav Ahluwalia No relevant relationships by Sumera Ahmad No relevant relationships by Hasan Ahmad Hasan Albitar No relevant relationships by Shivam Damani No relevant relationships by Sue Donlinger No relevant relationships by Ashley Egan No relevant relationships by Sumedh Hoskote No relevant relationships by Vivek Iyer No relevant relationships by Kinnera Jalagam No relevant relationships by Jeremiah Joyce monograph relationship with BMJ Please note: $1-$1000 by Karina Keogh, value=Honoraria Site PI for pharmaceutical trial relationship with AstraZeneca Please note: 2022 by Karina Keogh, value=Site PI for pharmaceutical tri Educational grant relationship with GSK/Potomac Center for Medical Education (PCME), Please note: 2019-2020 by Karina Keogh, value=Travel No relevant relationships by Kaiser Lim No relevant relationships by Ashokakumar Patel No relevant relationships by Shivaram Poigai Arunachalam No relevant relationships by Nanditha Venkatesan
更多查看译文
关键词
throat clearing events,deep learning,artificial intelligence,cough,automated detection
AI 理解论文
溯源树
样例
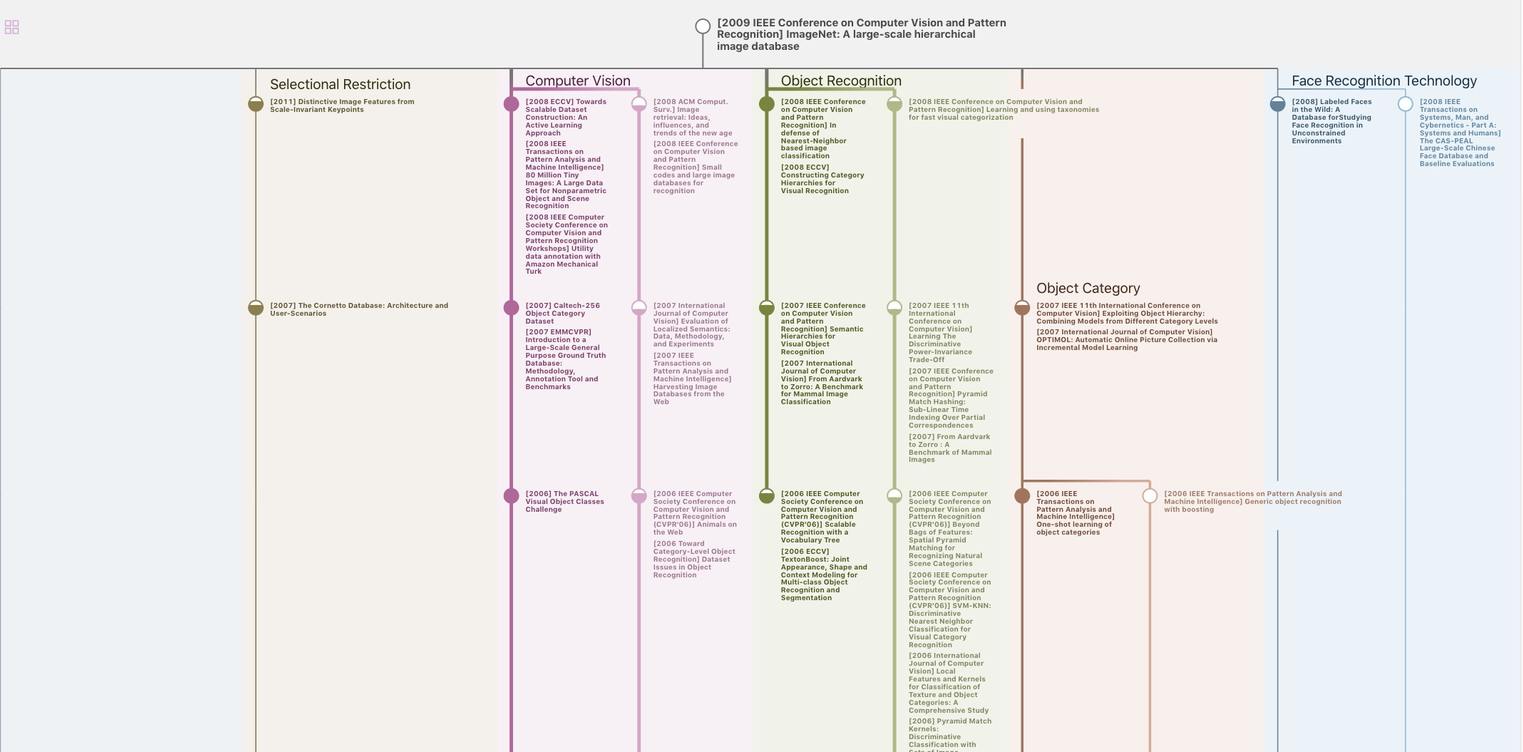
生成溯源树,研究论文发展脉络
Chat Paper
正在生成论文摘要