Adaptive local Principal Component Analysis improves the clustering of high-dimensional data
PATTERN RECOGNITION(2024)
摘要
In local Principal Component Analysis (PCA), a distribution is approximated by multiple units, each repre-senting a local region by a hyper-ellipsoid obtained through PCA. We present an extension for local PCA which adaptively adjusts both the learning rate of each unit and the potential function which guides the competition between the local units. Our local PCA method is an online neural network method where unit centers and shapes are modified after the presentation of each data point. For several benchmark distributions, we demonstrate that our method improves the overall quality of clustering, especially for high-dimensional distributions where many conventional methods do not perform satisfactorily. Our online method is also well suited for the processing of streaming data: The two adaptive mechanisms lead to a quick reorganization of the clustering when the underlying distribution changes.
更多查看译文
关键词
High-dimensional clustering,Potential function,Adaptive learning rate,Ranking criteria,Neural network-based PCA,Mixture PCA,Local PCA
AI 理解论文
溯源树
样例
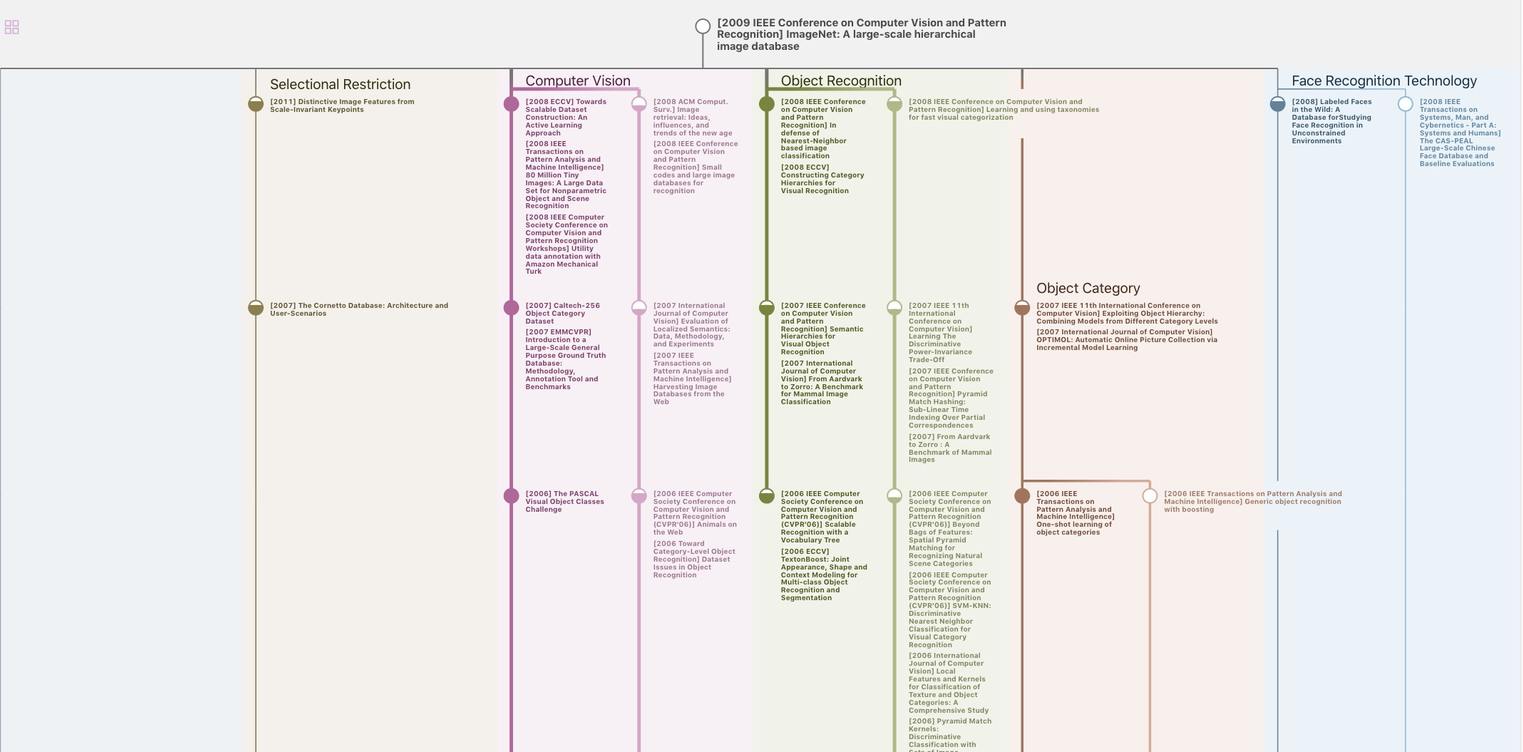
生成溯源树,研究论文发展脉络
Chat Paper
正在生成论文摘要