An artificial intelligence method for phenotyping of OCT scans using unsupervised and self-supervised deep learning
bioRxiv (Cold Spring Harbor Laboratory)(2023)
摘要
Artificial intelligence (AI) has been increasingly used to analyze optical coherence tomography (OCT) images to better understand physiology and genetic architecture of ophthalmic diseases. However, to date, research has been limited by the inability to transfer OCT phenotypes from one dataset to another. In this work, we propose a new AI method for phenotyping and clustering of OCT-derived retinal layer thicknesses using unsupervised and self-supervised methods in a large clinical dataset using glaucoma as a model disease and subsequently transfer our phenotypes to a large biobank. The model includes a deep learning model, manifold learning, and a Gaussian mixture model. We also propose a correlation analysis for the performance evaluation of our model based on Pearson correlation coefficients. Our model was able to identify clinically meaningful OCT phenotypes and successfully transfer phenotypes from one dataset to another. Overall, our results will contribute to stronger research methodologies for future research in OCT imaging biomarkers, augment testing of OCT phenotypes in multiple datasets, and ultimately improve our understanding of pathophysiology and genetic architecture of ocular diseases.
### Competing Interest Statement
The authors have declared no competing interest.
更多查看译文
关键词
oct scans,deep learning,phenotyping,artificial intelligence,artificial intelligence method,self-supervised
AI 理解论文
溯源树
样例
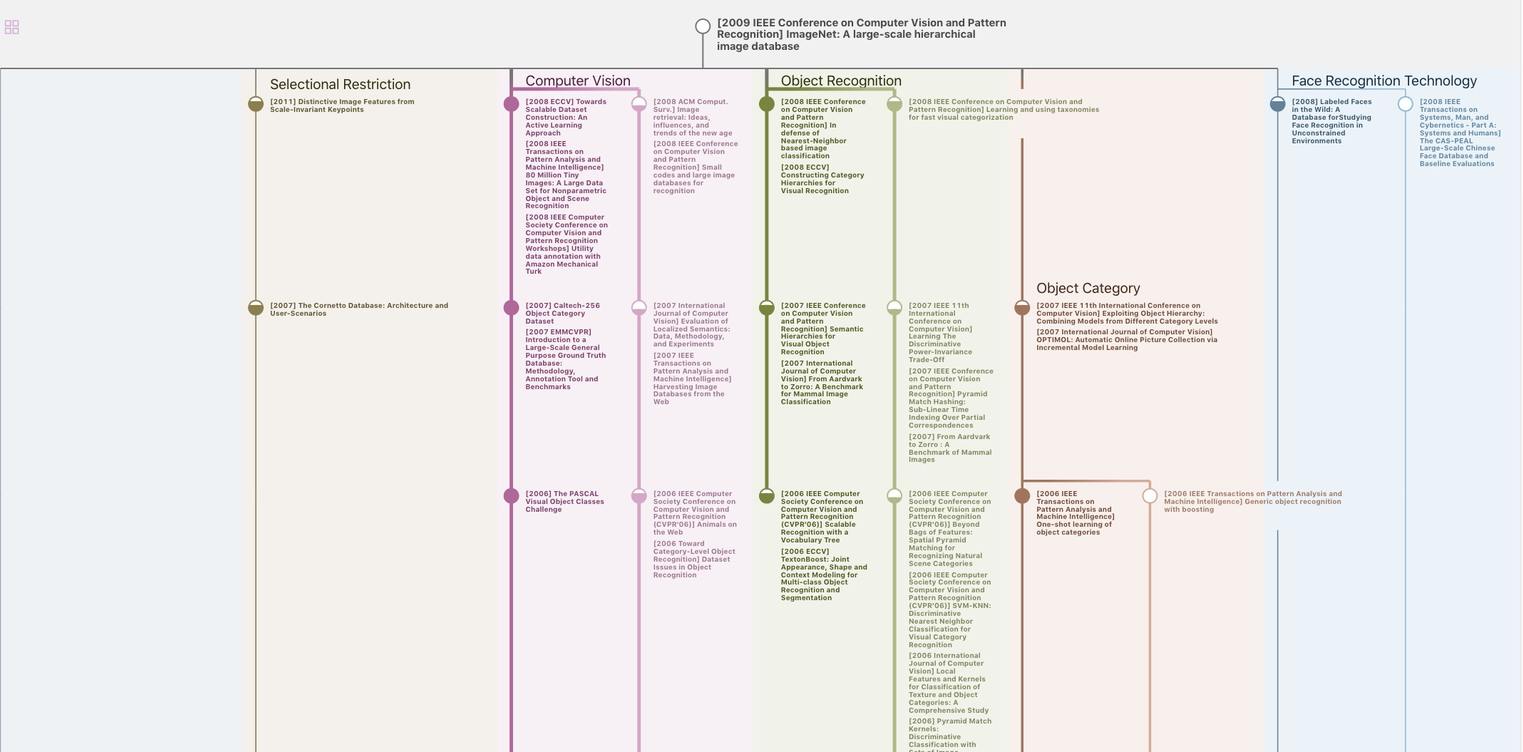
生成溯源树,研究论文发展脉络
Chat Paper
正在生成论文摘要