A Deep Dive into Single-Cell RNA Sequencing Foundation Models
bioRxiv (Cold Spring Harbor Laboratory)(2023)
摘要
Large-scale foundation models, which are pre-trained on massive, unlabeled datasets and subsequently fine-tuned on specific tasks, have recently achieved unparalleled success on a wide array of applications, including in healthcare and biology. In this paper, we explore two foundation models recently developed for single-cell RNA sequencing data, scBERT and scGPT. Focusing on the fine-tuning task of cell type annotation, we explore the relative performance of pre-trained models compared to a simple baseline, L1-regularized logistic regression, including in the few-shot setting. We perform ablation studies to understand whether pretraining improves model performance and to better understand the difficulty of the pre-training task in scBERT. Finally, using scBERT as an example, we demonstrate the potential sensitivity of fine-tuning to hyperparameter settings and parameter initializations. Taken together, our results highlight the importance of rigorously testing foundation models against well established baselines, establishing challenging fine-tuning tasks on which to benchmark foundation models, and performing deep introspection into the embeddings learned by the model in order to more effectively harness these models to transform single-cell data analysis. Code is available at https://github.com/clinicalml/sc-foundation-eval.
### Competing Interest Statement
G.G. receives research funds from IBM & Pharmacyclics, and is a founder, consultant, and has privately held equity in Scorpion Therapeutics; G.G is also an inventor on patent applications filed by the Broad Institute related to MSMuTect and MSMutSig (WO 2019/083594); POLYSOLVER (US-2016-0298185); SignatureAnalyzer-GPU (US-2021-0358574); and MSIDetect (WO 2022/098997 and WO 2022/099004); D.S. is President and CEO of Layer Health, has privately held equity in Curai and ASAPP, and receives research funds from Takeda and IBM. N.S. is funded by a PhD fellowship from Google. The remaining authors declare no competing interests.
更多查看译文
关键词
single-cell single-cell rna,sequencing
AI 理解论文
溯源树
样例
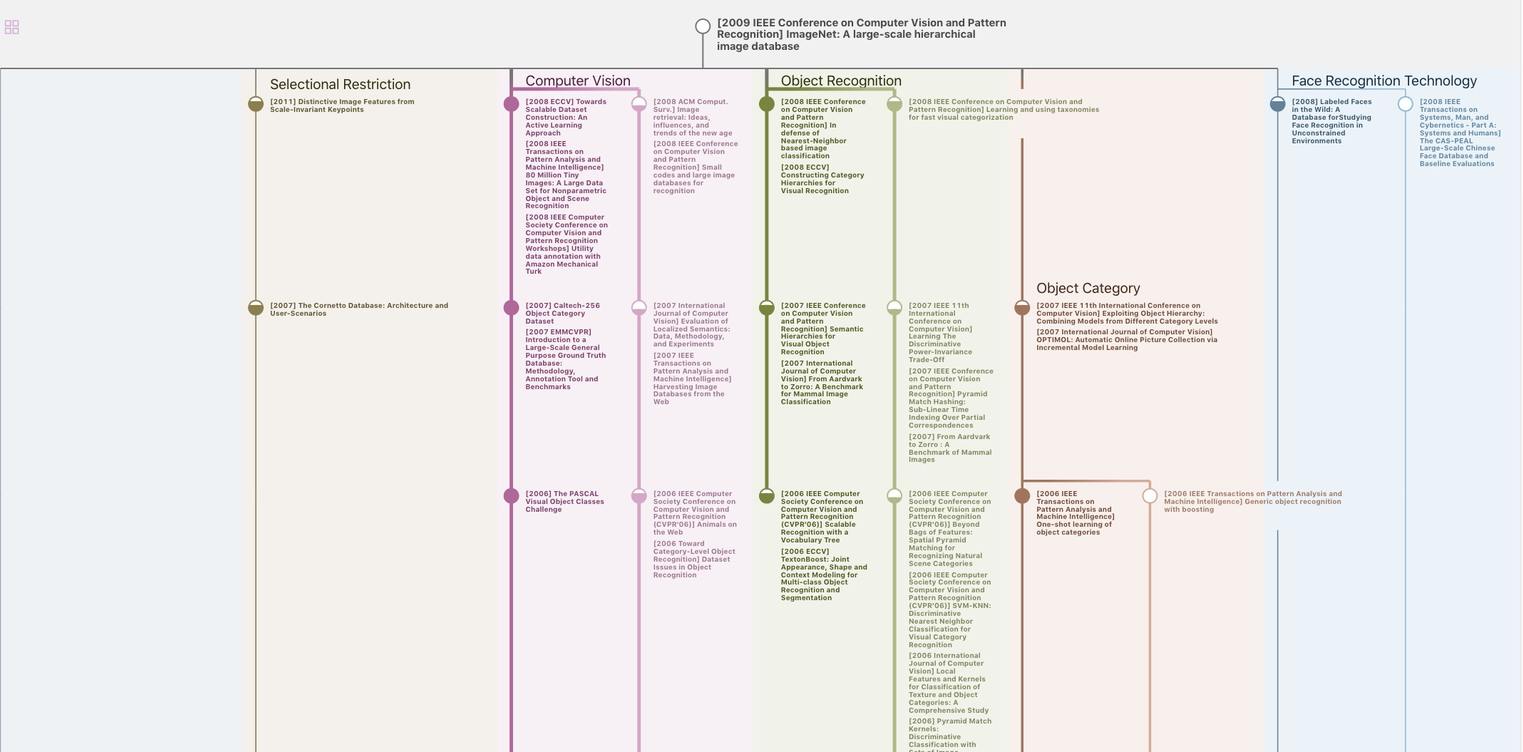
生成溯源树,研究论文发展脉络
Chat Paper
正在生成论文摘要