Commonsense-based adversarial learning framework for zero-shot stance detection
NEUROCOMPUTING(2024)
摘要
Zero-shot stance detection (ZSSD) aims to identify the stance for a diverse range of topics with limited or no training data. It is more suitable for evolving real-world topics that are constantly evolving. However, ZSSD faces major challenges which are the lack of unseen target information and the inability to generalize training information to unseen targets effectively. To overcome these challenges, we propose a commonsense-based adversarial learning framework that comprises a commonsense graph encoder and a feature separation adversarial network. Specifically, our approach utilizes an external commonsense graph encoder to learn unseen target information. Moreover, we design a novel feature separation adversarial network to learn target-invariant and target-specific features, which enhances the model's ability to reason beyond the seen targets. Experiments on two benchmark datasets show that our proposed framework achieves state-of-the-art performance.
更多查看译文
关键词
Natural language process,Stance detection,Deep learning,Zero-shot,Adversarial learning
AI 理解论文
溯源树
样例
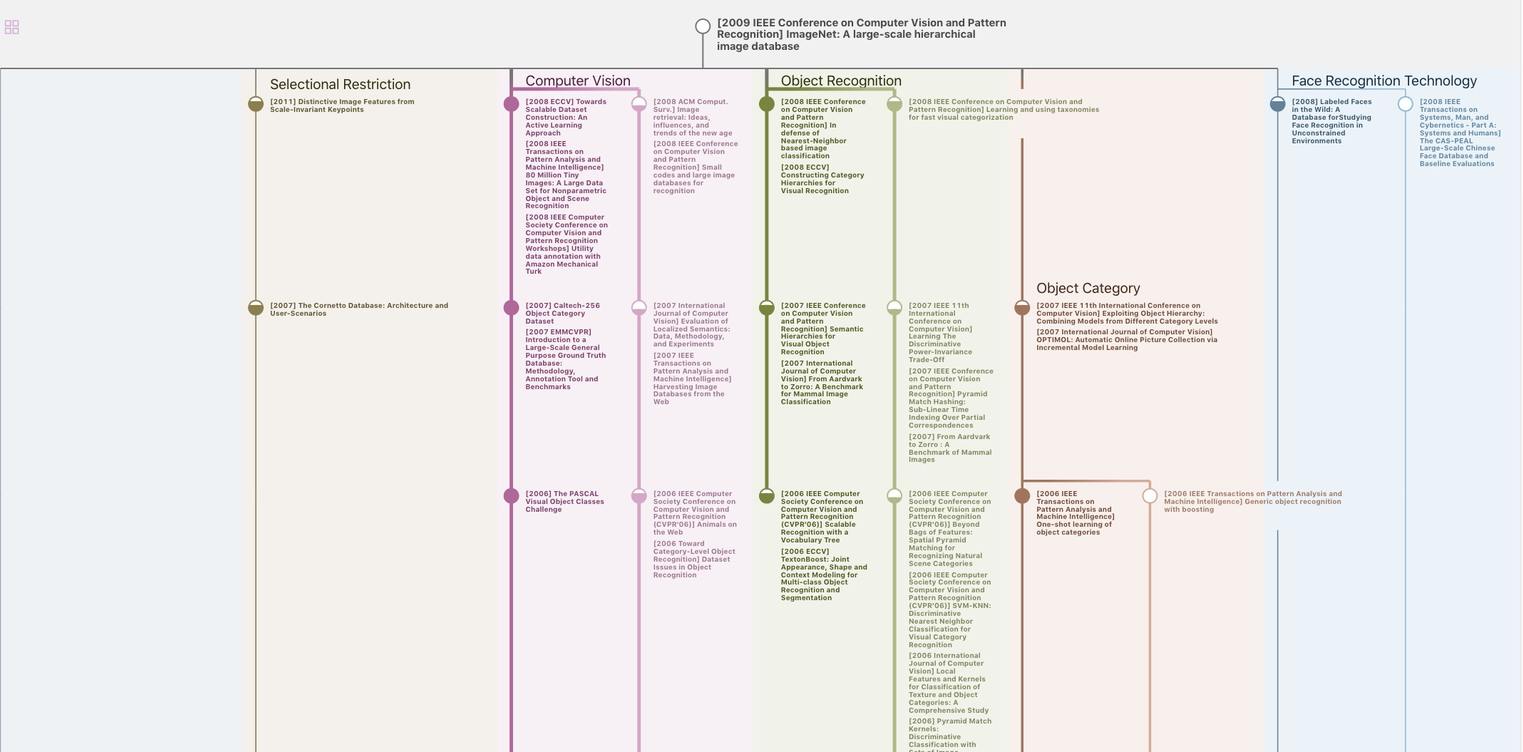
生成溯源树,研究论文发展脉络
Chat Paper
正在生成论文摘要