A Novel Motor Fault Diagnosis Method Based on Generative Adversarial Learning with Distribution Fusion of Discrete Working Conditions
CMES-COMPUTER MODELING IN ENGINEERING & SCIENCES(2023)
摘要
Many kinds of electrical equipment are used in civil and building engineering. The motor is one of the main power components of this electrical equipment, which can provide stable power output. During the long-term use of motors, various motor faults may occur, which affects the normal use of electrical equipment and even causes accidents. It is significant to apply fault diagnosis for the motors at the construction site. Aiming at the problem that signal data of faulty motor lack diversity, this research designs a multi-layer perceptron Wasserstein generative adversarial network, which is used to enhance training data through distribution fusion. A discrete wavelet decomposition algorithm is employed to extract the low-frequency wavelet coefficients from the original motor current signals. These are used to train the multi-layer perceptron Wasserstein generative adversarial model. Then, the trained model is applied to generate fake current wavelet coefficients with the fused distribution. A motor fault classification model consisting of a feature extractor and pattern recognizer is built based on perceptron. The data augmentation experiment shows that the fake dataset has a larger distribution than the real dataset. The classification model trained on a real dataset, fake dataset and combined dataset achieves 21.5%, 87.2%, and 90.1% prediction accuracy on the unseen real data, respectively. The results indicate that the proposed data augmentation method can effectively generate fake data with the fused distribution. The motor fault classification model trained on a fake dataset has better generalization performance than that trained on a real dataset.
更多查看译文
关键词
Motor fault diagnosis,data augmentation,wavelet decomposition,generative adversarial network,civil and building engineering
AI 理解论文
溯源树
样例
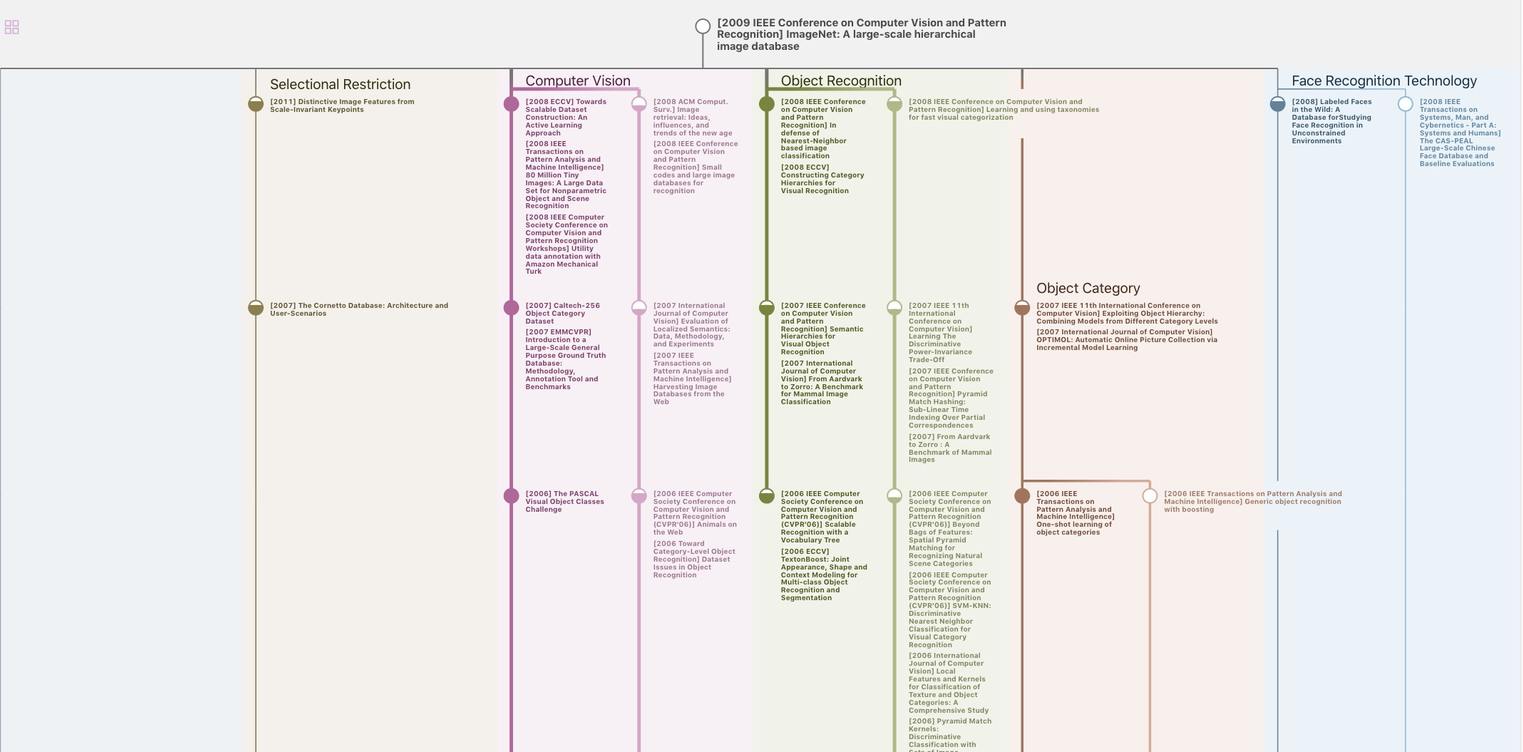
生成溯源树,研究论文发展脉络
Chat Paper
正在生成论文摘要