On the use of matrix profiles and optimal transport theory for multivariate time series anomaly detection within structural health monitoring
Mechanical Systems and Signal Processing(2023)
摘要
In order for a practical application of structural health monitoring to be considered successful, not only is the detection of anomalies important but so is the tracking of various other behaviors, which may be induced by changing operational conditions or environmental variations. Rather than being problems of anomaly detection, these are known as problems of changing contexts or changing semantics. In this paper, we propose a novel, and efficient method which is able to perform both tasks: anomaly detection, and semantic analysis, simultaneously, and which is also able to scale to a multi-sensor network array. The proposed methodology works to combine a recent development from the field of time series data mining known as matrix profiling, as well as ideas from a popular area of mathematics known as optimal transport theory. In particular, matrix profiles will be used to generate curves of semantic segmentation, over multivariate time series data. From here, it will be demonstrated that by finding the unbalanced optimal transport barycenter one can successfully pool together information from a sensor array network in such a way that the presence of false positive transition points is reduced significantly when compared to the baseline mean. Moreover, this will be demonstrated to occur in a hierarchical fashion by employing a variational mode decomposition across each sensor in the network array. Overall, the proposed methodology: (i) Can work in the small data context without requiring a large amount of data for model training and validation, (ii) Applies readily to unsupervised and semi-supervised learning settings, (iii) Can extend to the online learning setting, (iv) Is adaptable and robust for sensor array network problems, (v) Does not require specifying any threshold, and (vi) Is highly interpretable. Finally, the proposed methodology will be validated on three different levels of investigation: (i) A numerical dataset of an aerospace model based on a two-dimensional plate model, (ii) An experimental dataset of a building from Los Alamos National Laboratories, and finally, (iii) A field investigation on a cable-stayed bridge located in Sydney, Australia.
更多查看译文
关键词
Structural health monitoring (SHM),Damage identification,Time series,Similarity joins,Variational mode decomposition,Cable-stayed bridge
AI 理解论文
溯源树
样例
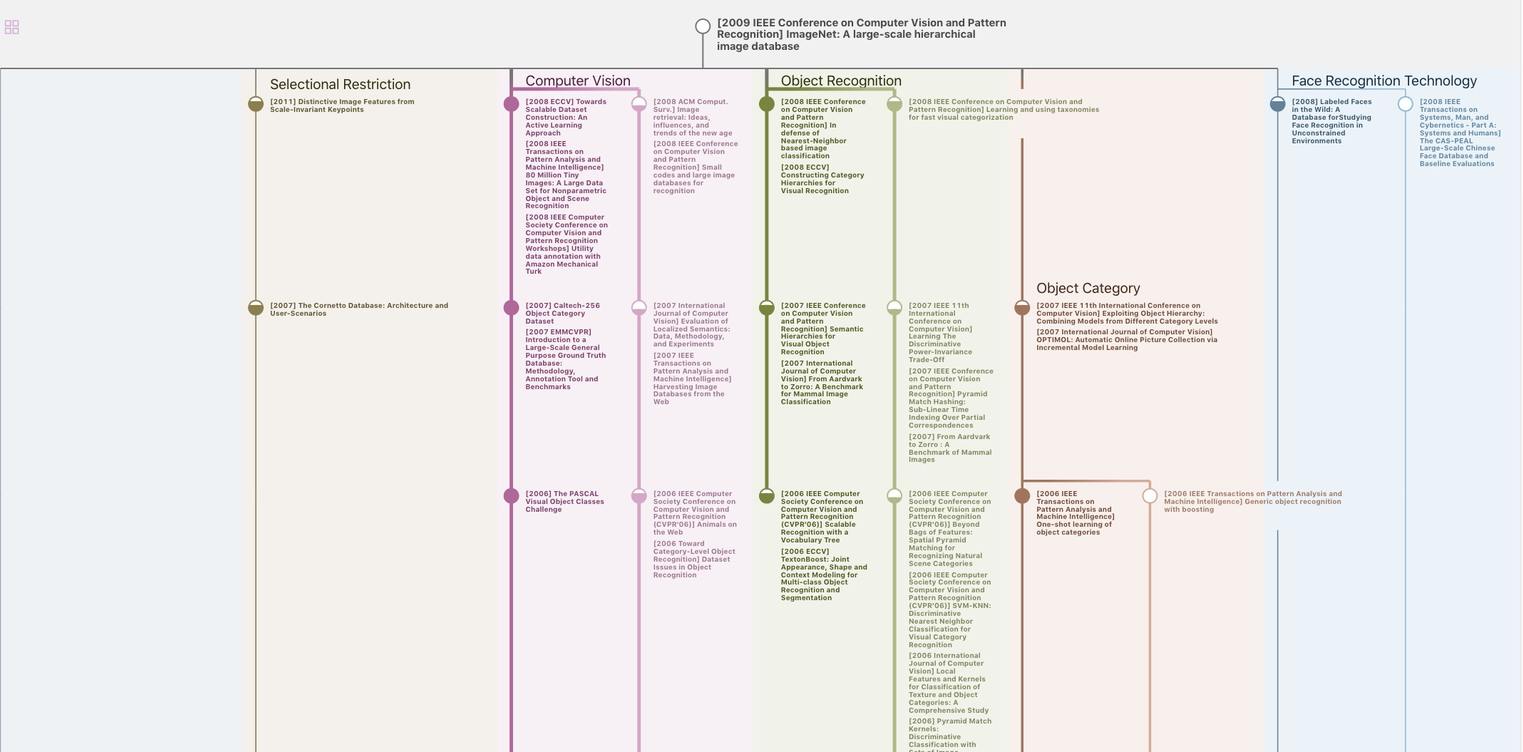
生成溯源树,研究论文发展脉络
Chat Paper
正在生成论文摘要