Hierarchical visual-semantic interaction for scene text recognition
INFORMATION FUSION(2024)
摘要
Proper interaction between visual and semantic features is crucial to obtain a powerful feature representation for scene text recognition (STR). The existing interaction methods usually treat visual and semantic features as distinct tokens and use transformers to learn contextual information and prior language knowledge, and they achieve promising performance for STR task. However, there still remain several issues needed to be further addressed such as the imbalance number and mis-alignment between visual and semantic features, and the necessarily of stacking transformers to a progressive improvement in accuracy. To this aim, this paper proposes a novel interaction manner namely hierarchical visual-semantic interaction (HVSI) which contains three novel modules including a hierarchical visual-semantic interaction module, fusion module, and visual-semantic alignment module. The hierarchical visual-semantic interaction module employs multiple visual-semantic interaction blocks in various scales to enhance the representation power of visual features and semantic features. To better exploit multi-scale visual and semantic features, the fusion module is introduced to fuse multiple semantic features based on attention mechanisms. Furthermore, our HVSI presents a simple plug-in block named visual-semantic alignment module to alleviate mis-alignment of semantic features by mapping them into a unified semantic space, which helps improve the performance of HVSI. Extensive experiments on multiple benchmarks including English and Chinese text recognition datasets show that our method obtains state-of-the-art or competitive performances.
更多查看译文
关键词
Scene text recognition,Visual semantic interaction,Scene text representation,Feature fusion
AI 理解论文
溯源树
样例
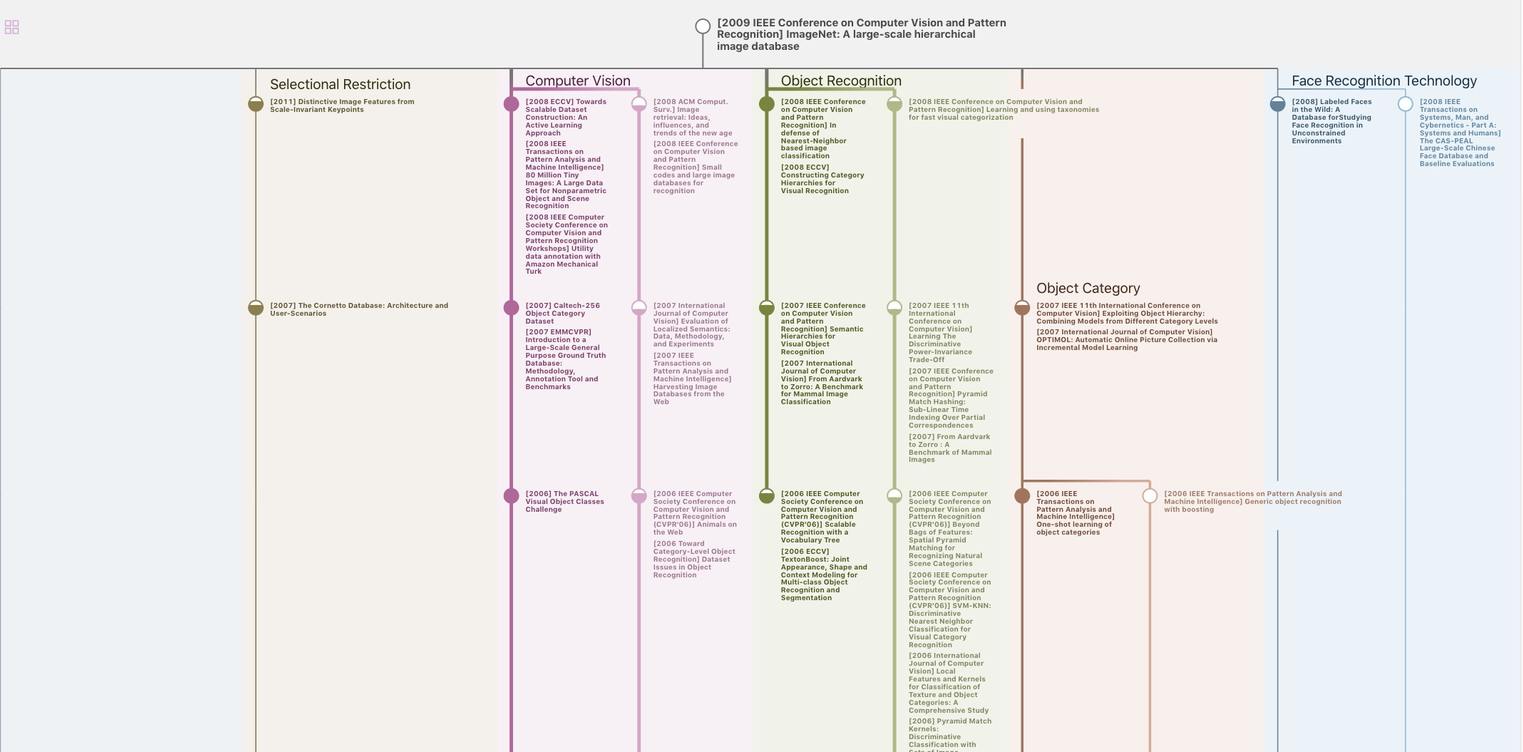
生成溯源树,研究论文发展脉络
Chat Paper
正在生成论文摘要