GSM: A generalized approach to Supervised Meta-blocking for scalable entity resolution
INFORMATION SYSTEMS(2024)
摘要
Entity Resolution (ER) constitutes a core data integration task that relies on Blocking in order to tame its quadratic time complexity. Schema-agnostic blocking achieves very high recall, requires no domain knowledge and applies to data of any structuredness and schema heterogeneity. This comes at the cost of many irrelevant candidate pairs (i.e., comparisons), which can be significantly reduced through Meta-blocking techniques, i.e., techniques that leverage the co-occurrence patterns of entities inside the blocks: first, a weighting scheme assigns a score to every pair of candidate entities in proportion to the likelihood that they are matching and then, a pruning algorithm discards the pairs with the lowest scores. Supervised Meta-blocking goes beyond this approach by combining multiple scores per comparison into a feature vector that is fed to a binary classifier. By using probabilistic classifiers, Generalized Supervised Meta-blocking associates every pair of candidates with a score that can be used: (i) by any pruning algorithm for retaining the set of candidate comparisons; and (ii) by state-of-the-art progressive ER methods to identify the most promising candidates as early as possible (when time is a critical component for the downstream applications that consume the data). For higher effectiveness, new weighting schemes are examined as features. Through an extensive experimental analysis, we identify the best pruning algorithms, their optimal sets of features as well as the minimum possible size of the training set. The resulting approaches achieve excellent performance across several established benchmark datasets.
更多查看译文
关键词
Entity resolution,Data integration,Progressive entity resolution,Meta-blocking
AI 理解论文
溯源树
样例
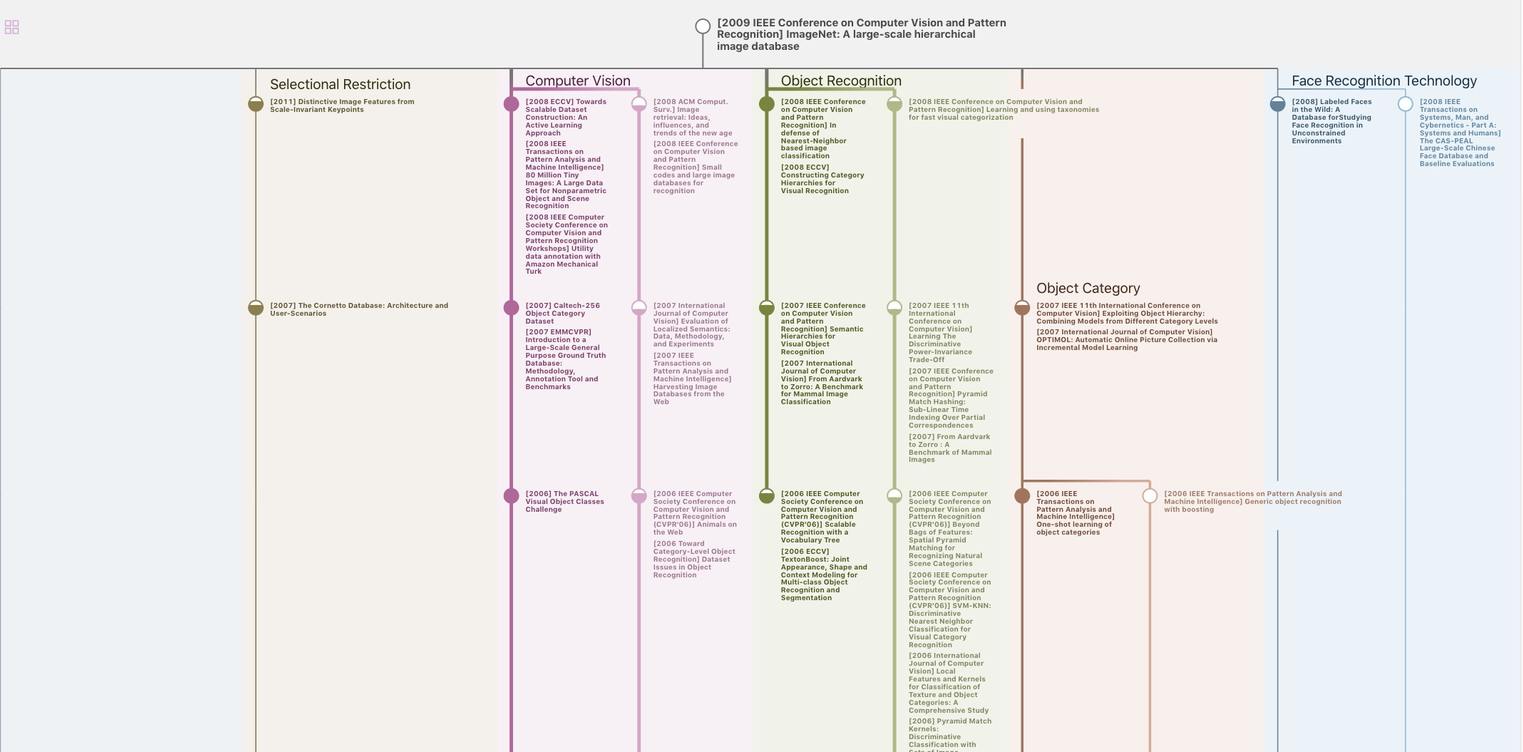
生成溯源树,研究论文发展脉络
Chat Paper
正在生成论文摘要