Multi-view Outlier Detection via Graphs Denoising
INFORMATION FUSION(2024)
摘要
Recently, multi-view outlier detection attracts increasingly more attention. Although existing multi-view outlier detection methods have demonstrated promising performance, they still suffer from some problems. Firstly, many methods make the assumption that the data have a clear clustering structure and detect the outliers by using some off-the-shelf clustering methods. Therefore, the performance of these methods depends on the clustering methods they used, and thus these methods are hard to handle complicated data. Secondly, some methods ignore the complicated structure or distribution of class outliers and directly learn a consensus representation by simply combining the representation of different views linearly. To tackle these problems, we propose a novel method named Multi-view Outlier Detection with Graph Denoising (MODGD). We first construct a graph for each view, and then learn a consensus graph by ensembling the multiple graphs. When fusing the multiple graphs, we explicitly characterize and extract the structured outliers on each graph and recover the multiple clean graphs for the ensemble. During the process of multiple graph denoising and fusion, we carefully design an outlier measurement criterion based on the characteristics of attribute and class outliers. The extensive experiments on benchmark data sets demonstrate the effectiveness and superiority of the proposed method. The codes of this paper are released in http://Doctor-Nobody.github.io/codes/MODGD.zip.
更多查看译文
关键词
Multi-view learning,Outlier detection,Multiple graph learning
AI 理解论文
溯源树
样例
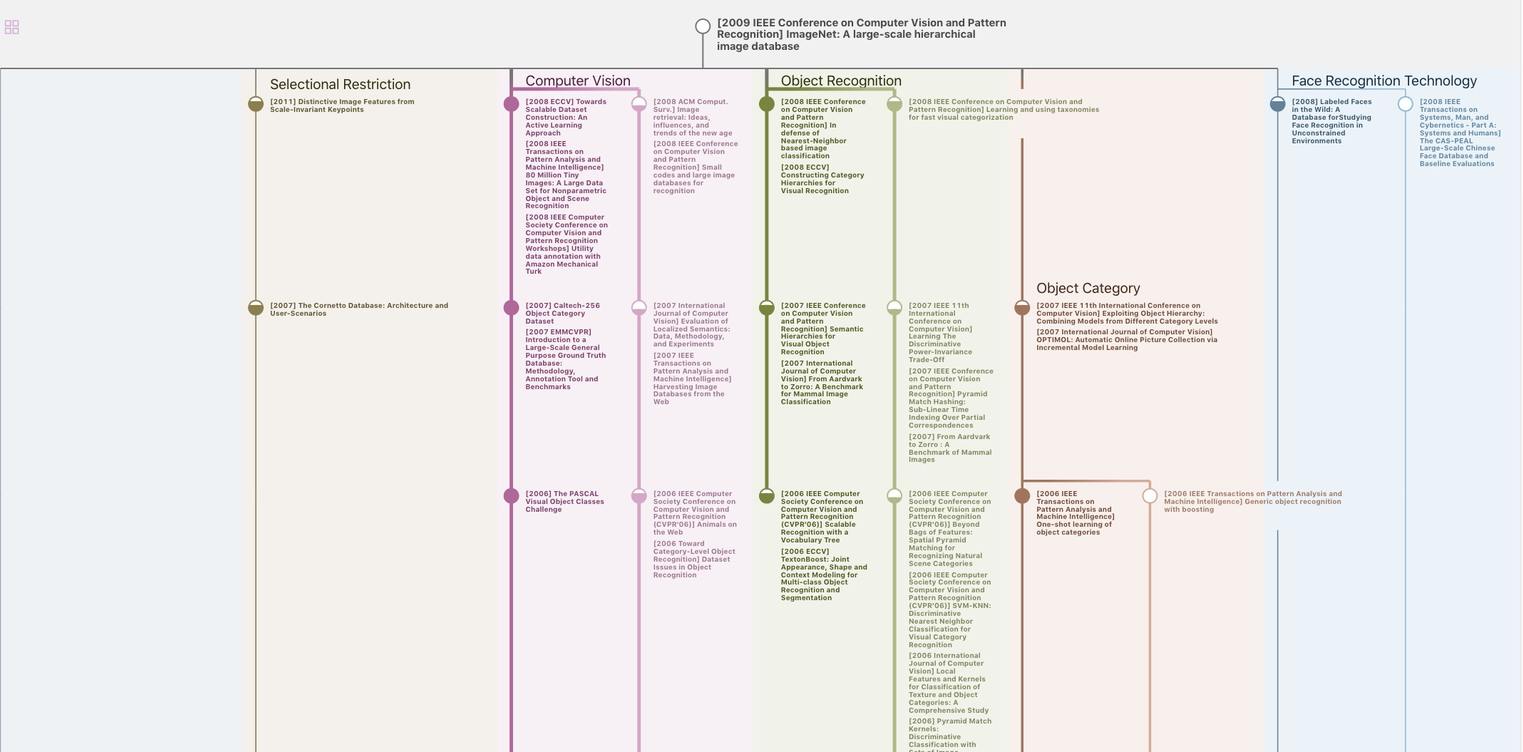
生成溯源树,研究论文发展脉络
Chat Paper
正在生成论文摘要