A novel well-logging data generation model integrated with random forests and adaptive domain clustering algorithms
GEOENERGY SCIENCE AND ENGINEERING(2023)
摘要
With geological evolution, reservoirs have hierarchical properties, leading to distinct domain characteristics in their logging curves, which can contribute to the reconstruction of unknown well logging curves. In recent years, many studies have used machine learning (ML) algorithms to reconstruct logging curves without incorporating domain features, which can cause their poor performance. Since decomposing raw data into domain data can optimize an expected variance and thus improves the accuracy and robustness of ML prediction models, we propose a random-forest-adaptive-domain-clustering (RF-ADC) model to generate unknown well logging curves. First, we present an adaptive domain clustering algorithm based on gap statistics (GS) and balanced iterative reducing and clustering using hierarchies (BIRCH). With the adaptive domain clustering process, well logging curves are automatically divided into microscope domains, and their corresponding domain models are constructed. Then we utilize the random forest (RF) algorithm to generate unknown well logging curves based on domain data. Taking logging curves of a shale gas reservoir as an example, our experiments show that the proposed RF-ADC model achieves promising results. Compared with the model without clustering operation, the RF-ADC model provides superior performance with its mean absolute error (MAE), mean squared error (MSE), root mean squared error (RMSE), and mean absolute percentage error (MAPE) values reducing by 15.55%, 39.30%, 18.95%, and 11.43%, respectively. In addition, the predictive performance of the proposed RF-ADC model is better than that of baseline models of long short-term memory network and convolutional neural network. Furthermore, after analyzing, the GS and BIRCH methods are applicable to obtaining an optimal number of domains and decomposing well logging data for horizontal wells. Our delineation results also provide insights into detailed reservoir recognition, which is important for further identification of reservoirs and stratification work. Moreover, the proposed RF-ADC model can also achieve effective prediction with a small dataset (MAE = 8.205, RMSE = 10.922, and MAPE = 0.039). In summary, this RF-ADC model provides a fast, economical, and effective tool for the generation of unknown well logging curves for horizontal wells in shale gas reservoirs. It will further facilitate the exploration and development of unconventional reservoirs.
更多查看译文
关键词
Well logs,Logs generation,Adaptive domain clustering,Gap statistic,BIRCH,Random forests
AI 理解论文
溯源树
样例
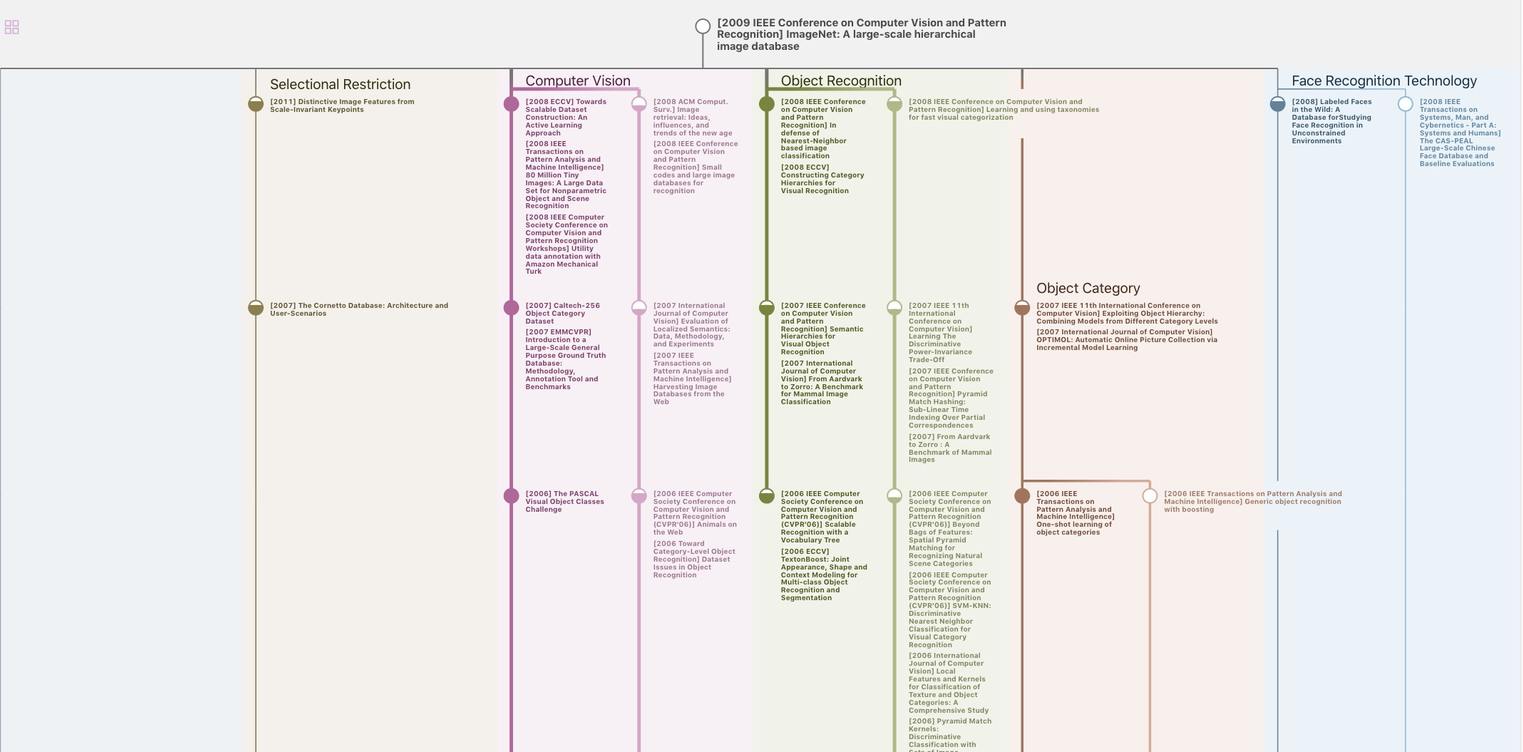
生成溯源树,研究论文发展脉络
Chat Paper
正在生成论文摘要