Deep Dual Graph attention Auto-Encoder for community detection
EXPERT SYSTEMS WITH APPLICATIONS(2024)
摘要
Community detection that tries to partition nodes with higher similarity in attributes and topology structures into clusters has garnered substantial attention in the last several decades. However, most of the existing methods (i) lack an effective module to learn a more meaningful representation for nodes by fusing information across different views, and (ii) neglect the higher-order modularity information. To address these shortcomings, we present a novel approach based on a Deep Dual Graph attention Auto-Encoder (DDGAE) for community detection. For a graph, the DDGAE incorporates its high-order modularity information and attribute information each as a separate view, and learns the latent representation of nodes by reconstructing its topology structure, attribute and modularity information. To acquire a clustering-friendly representation, a self-training method is designed to optimize the learning process, and during which the community assignment can also be directly determined. Experimental results on five publicly available datasets demonstrate that our approach outperforms the state-of-the-art algorithms. The code used for analyses is accessible as a Code Ocean capsule at https://doi.org/10.24433/CO.2474269.v1.
更多查看译文
关键词
Community detection,Graph attention auto-encoder,Self-training
AI 理解论文
溯源树
样例
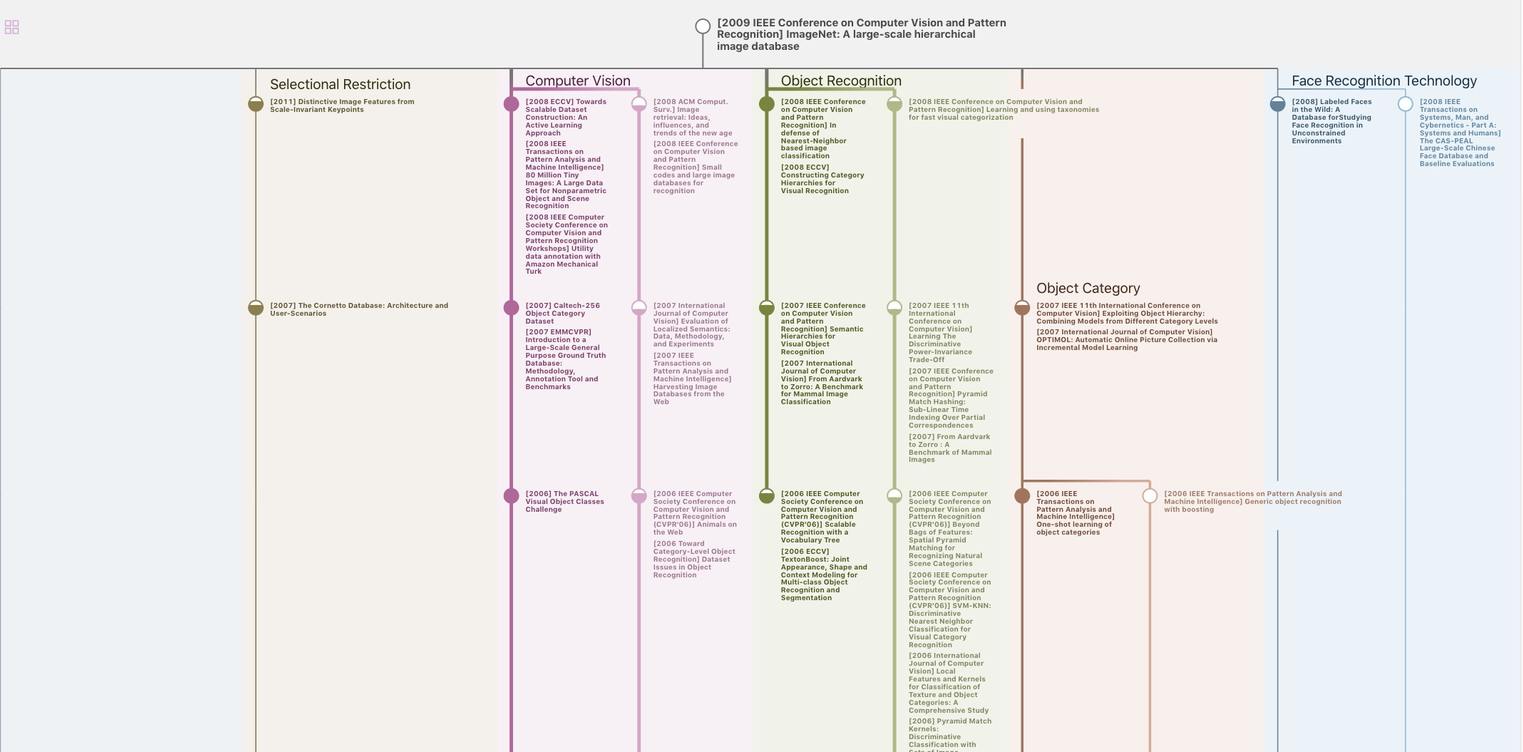
生成溯源树,研究论文发展脉络
Chat Paper
正在生成论文摘要