An information entropy-driven evolutionary algorithm based on reinforcement learning for many-objective optimization
EXPERT SYSTEMS WITH APPLICATIONS(2024)
摘要
Many-objective optimization problems (MaOPs) are challenging tasks involving optimizing many conflict-ing objectives simultaneously. Decomposition-based many-objective evolutionary algorithms have effectively maintained a balance between convergence and diversity in recent years. However, these algorithms face challenges in accurately approximating the complex geometric structure of irregular Pareto fronts (PFs). In this paper, an information entropy-driven evolutionary algorithm based on reinforcement learning (RL-RVEA) for many-objective optimization with irregular Pareto fronts is proposed. The proposed algorithm leverages reinforcement learning to guide the evolution process by interacting with the environment to learn the shape and features of PF, which adaptively adjusts the distribution of reference vectors to cover the PFs structure effectively. Moreover, an information entropy-driven adaptive scalarization approach is designed in this paper to reflect the diversity of nondominated solutions, which facilitates the algorithm to balance multiple competing objectives adaptively and select solutions efficiently while maintaining individual diversity. To verify the effectiveness of the proposed algorithm, the RL-RVEA compared with seven state-of-the-art algorithms on the DTLZ, MaF, and WFG test suites and four real-world MaOPs. The results of the experiments demonstrate that the suggested algorithm provides a novel and practical method for addressing MaOPs with irregular PFs.
更多查看译文
关键词
Many-objective optimization,Evolutionary algorithm,Reinforcement learning,Irregular pareto fronts,Information entropy
AI 理解论文
溯源树
样例
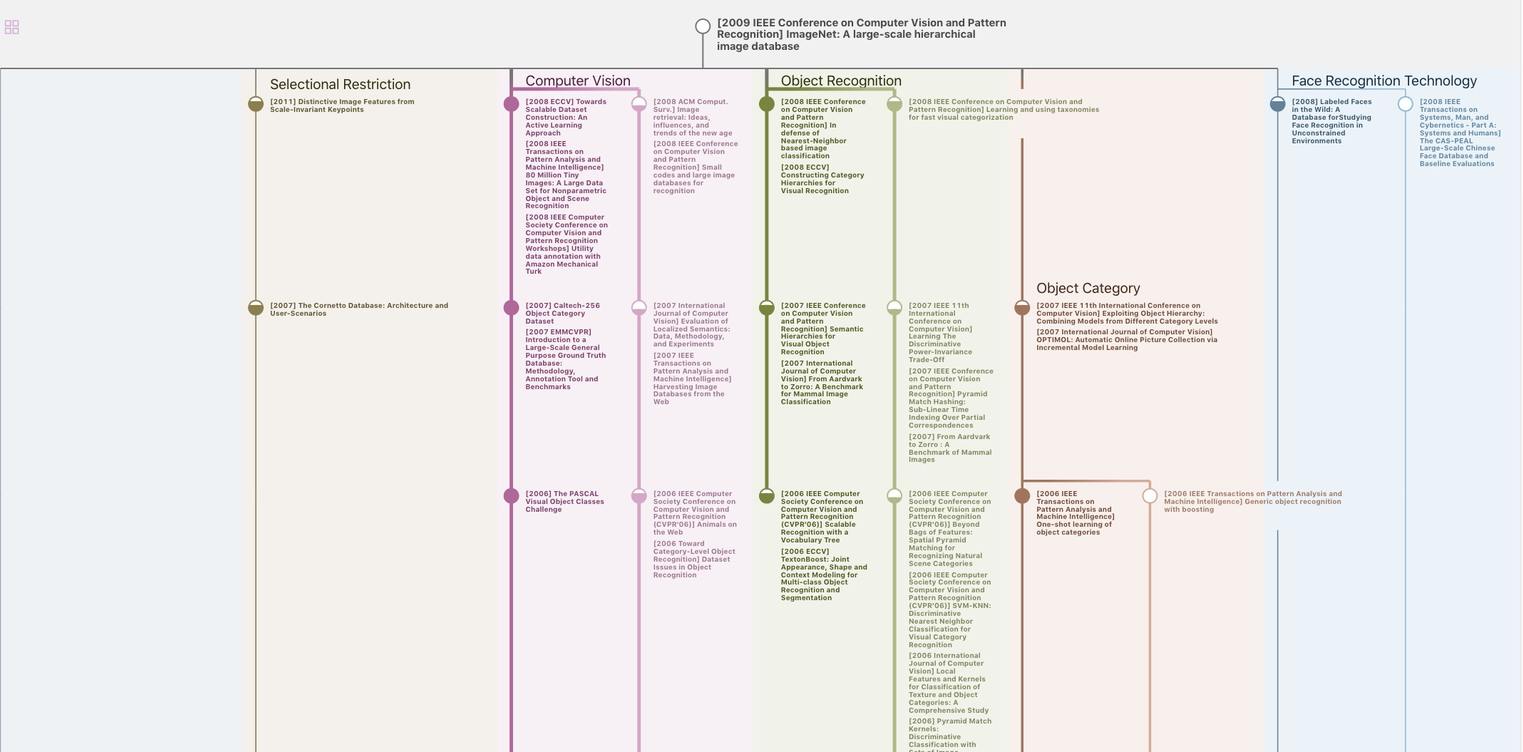
生成溯源树,研究论文发展脉络
Chat Paper
正在生成论文摘要