Self-supervised medical slice interpolation network using controllable feature flow
EXPERT SYSTEMS WITH APPLICATIONS(2024)
摘要
Deep learning-based image interpolation methods are confronted with various challenges in its application to anisotropic medical volumetric data (i.e., CT and MR images) out of the complex nonlinear deformation and the scarcity of high-quality images. This paper proposes a self-supervised multiple medical slice interpolation network using controllable feature flow. Firstly, a Controllable Feature Flow Network (CFFNet) is proposed to estimate the complex nonlinear deformation of medical images. In CFFNet, we first use a deformation-aware network and a spatial channel modulation module to predict the bi-directional feature flows from the source slices to the target slices by considering an additional position parameter, and then the learned feature flows are used to synthesize the target intermediate features by using the deformable convolution. Secondly, an improved two-stage self-supervised framework is proposed to alleviate the dependency on high-quality medical images. In the first stage, the synthesized training pairs along the dense sagittal and coronal directions are adopted to pre-train the CFFNet. In the second one, the sparse axial slices are used to fine-tune the CFFNet with the cycle-consistency constraint and a feature domain smooth loss. Experimental results illustrate that the proposed CFFNet assumes superior performance on medical slice interpolation tasks with fewer parameters and the proposed self-supervised CFFNet obtains competitive results compared with other fully supervised algorithms. We also evaluate our model on HepaticVessel segmentation task, the proposed method can effectively enhance the continuity and smoothness of the 3D vessel structures, showing promising results on clinical application. The code is available at: https://codeocean.com/capsule/8945297/tree/v1.
更多查看译文
关键词
Medical image interpolation,Super-resolution,Self-supervised learning,Deformable convolution
AI 理解论文
溯源树
样例
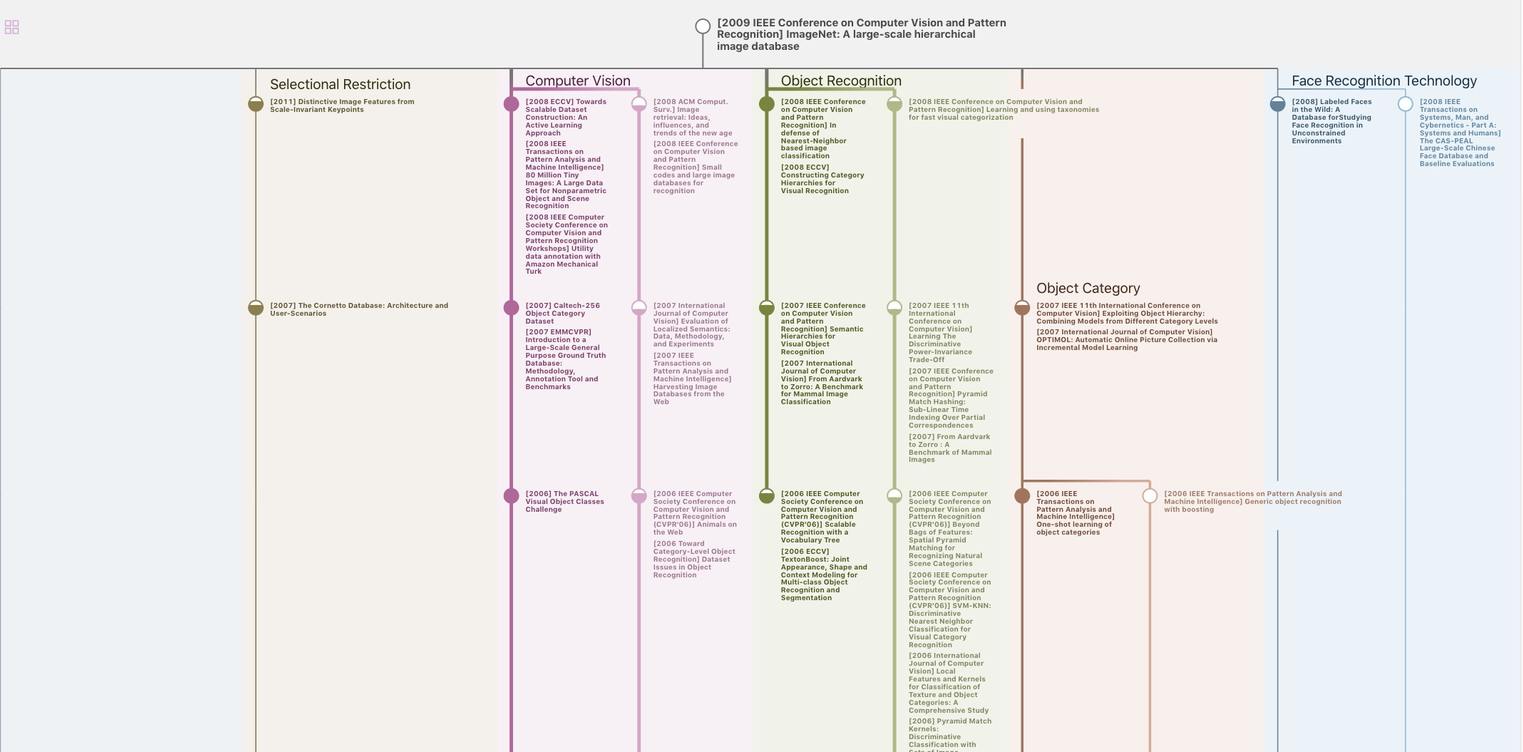
生成溯源树,研究论文发展脉络
Chat Paper
正在生成论文摘要