Hydrogen leakage location prediction at hydrogen refueling stations based on deep learning
Energy(2023)
摘要
Accurate and efficient localization of hydrogen leakage is crucial for ensuring the safe and stable operation of hydrogen refueling stations. In this paper, a hybrid model (CEEMDAN-CNN-LSTM) based on data noise reduction and deep learning is proposed to predict the location of hydrogen leakage in hydrogen refueling stations. The model employs the complete ensemble empirical mode decomposition with adaptive noise (CEEMDAN) algorithm to effectively reduce noise and process 720 hydrogen leakage condition datasets. The CNN-LSTM model is constructed by synergistically combining the spatiotemporal feature extraction capabilities of the convolutional neural network (CNN) with the temporal processing capabilities of the long-short-term memory neural network (LSTM) while optimizing hyperparameter combinations through GridSearch. Compared with other models, the proposed CEEMDAN-CNN-LSTM model demonstrates superior prediction performance, achieving an accuracy of 99.54 %, precision of 99.42 %, recall of 99.54 %, and F1-score of 99.46 %. Moreover, when utilizing a 5-s duration of leakage data as input, the model attains an impressive accuracy of 97.64 %. These results underscore the favorable application prospects of the CEEMDAN-CNN-LSTM model in the field of gas leak localization.
更多查看译文
关键词
Hydrogen leakage,Leakage location,Hydrogen refueling station,CNN,LSTM,CEEMDAN
AI 理解论文
溯源树
样例
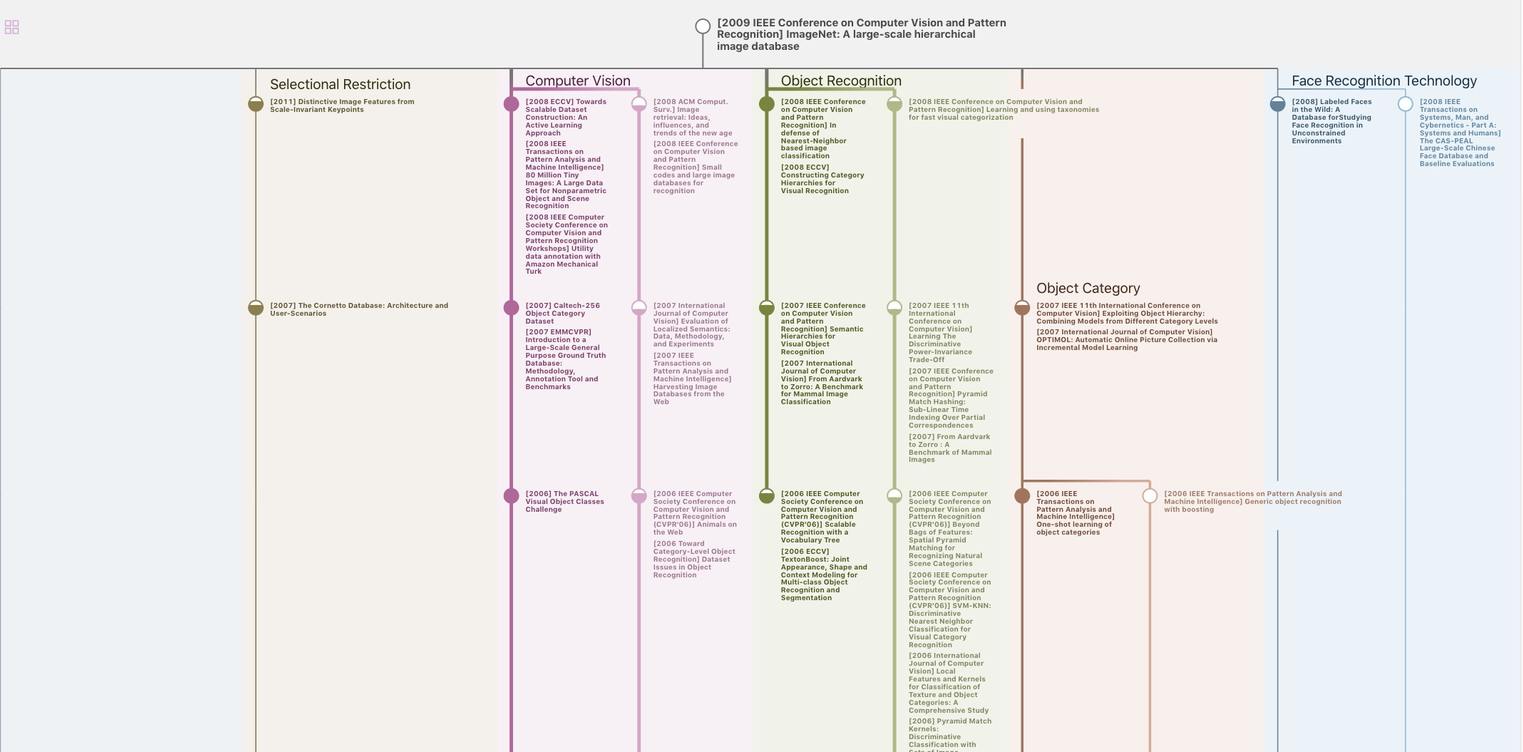
生成溯源树,研究论文发展脉络
Chat Paper
正在生成论文摘要