Using machine learning to predict the long-term performance of fibre-reinforced polymer structures: A state-of-the-art review
Construction and Building Materials(2023)
摘要
When exposed to environmental conditions, fibre-reinforced polymer (FRP) composites are prone to material degradation. The environmental reduction factor in different structural codes reflects the significant effect of the long-term durability of FRPs in aggressive environments. Traditional prediction methods rely on oversimplified premises, which may result in erroneous errors. Due to its proficiency in dealing with complex non-linear structural problems, machine learning (ML) offers a unique potential to increase the predictability of struc-tural engineering factors. This can be attributed to the recent advancements in ML techniques, which leverage their robustness when handling large datasets, as well as the increased processing power that facilitates more efficient data analysis. This article reviews the current implementation cases and capabilities of ML algorithms in overcoming the shortcomings of conventional models for predicting the durability performance of FRP systems. According to the literature, it was found that the efficiency of ML approach varies significantly depending on the quality and comprehensiveness of the database. While various researcher-employed algorithms generally yield accurate predictions for retaining mechanical properties in FRP composites with minor errors, sensitivity analysis highlights varied impacts of variables when using different datasets or machine learning algorithms. This vari-ance may arise from factors like inadequate or low-quality datasets, insufficient training, overfitting, and other influences. More experimental data are needed to enhance the current database to effectively apply ML in more applications for FRPs under different loading and environmental conditions. The paper ends by suggesting future research directions in this field.
更多查看译文
关键词
Machine learning, Artificial intelligence, FRP durability, Service-life
AI 理解论文
溯源树
样例
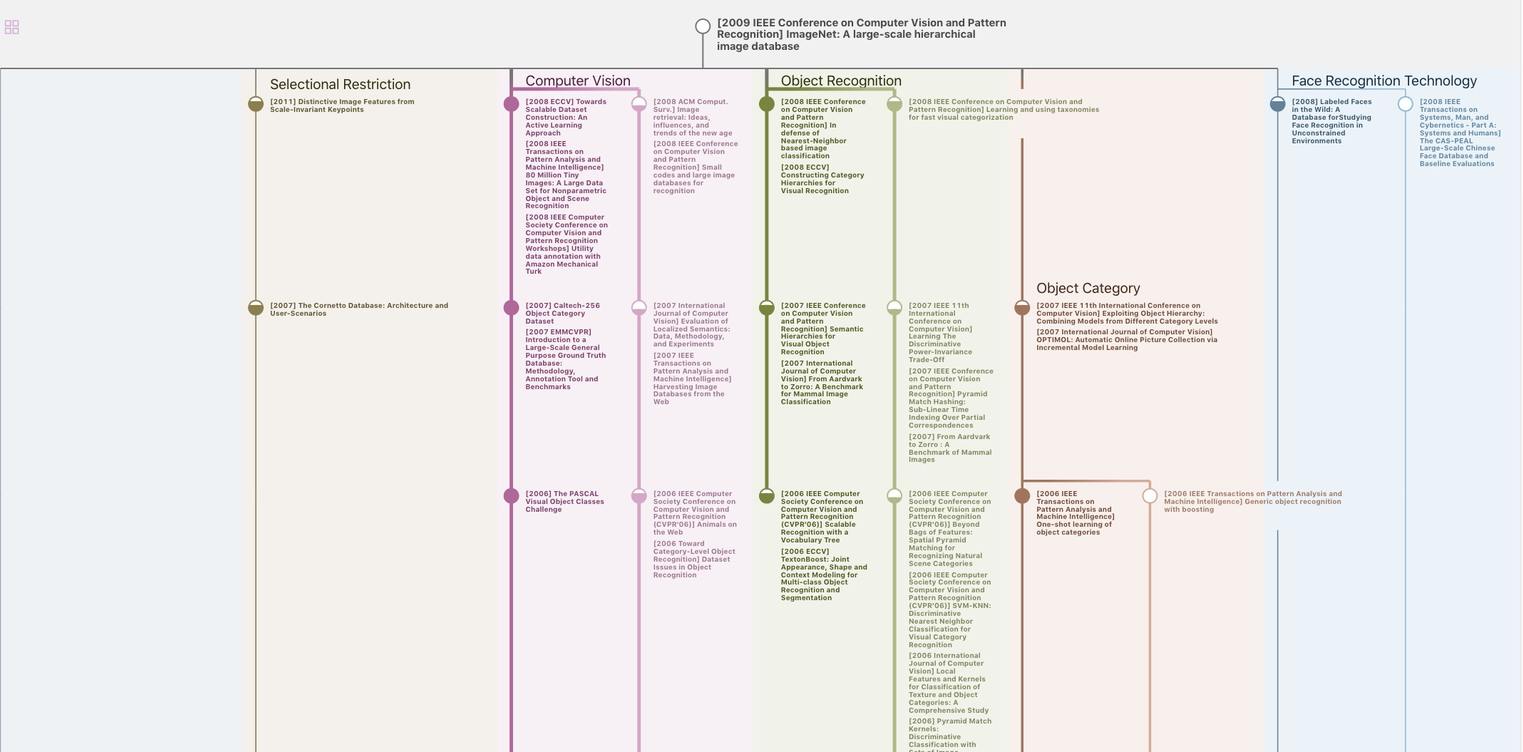
生成溯源树,研究论文发展脉络
Chat Paper
正在生成论文摘要