A deep model towards accurate boundary location and strong generalization for medical image segmentation
BIOMEDICAL SIGNAL PROCESSING AND CONTROL(2024)
摘要
Accurate medical image segmentation plays a crucial role in computer-assisted diagnosis and monitoring. However, due to the complexity of medical images and the limitations of image acquisition, most of the current segmentation models still face the challenges of imprecise boundary positioning and poor generalization ability, which greatly affect their application in clinical practices. To address these issues, we propose a new deep learning-based medical image semantic segmentation network (BLG-Net). To improve the accuracy of object boundary location, a Local-global module (Logo) is proposed. It uses the combination of a self-attention mechanism and a cross-attention mechanism to model intra-sample and inter-sample long-range dependencies from local and global perspectives, respectively, to help recover the spatial structures. To further enhance the generalization ability of the BLG-Net, we develop a generalization enhancement module (GEM), which can adaptively adjust the classifier weights of the network according to the features of the test images extracted during the test process to alleviate the distribution shift issues. Besides, we design a multi-granularity loss (MGL) function, which can guide the network to learn the object region and its boundary details at the pixel, patch, and map levels, respectively. Extensive experiments on five medical image segmentation tasks demonstrate the improvements of our method. We further verify the model's generalization ability to unseen data on two other popular datasets with different modalities.
更多查看译文
关键词
Attention mechanism,Convolutional neural network,Medical image segmentation,Model generalization
AI 理解论文
溯源树
样例
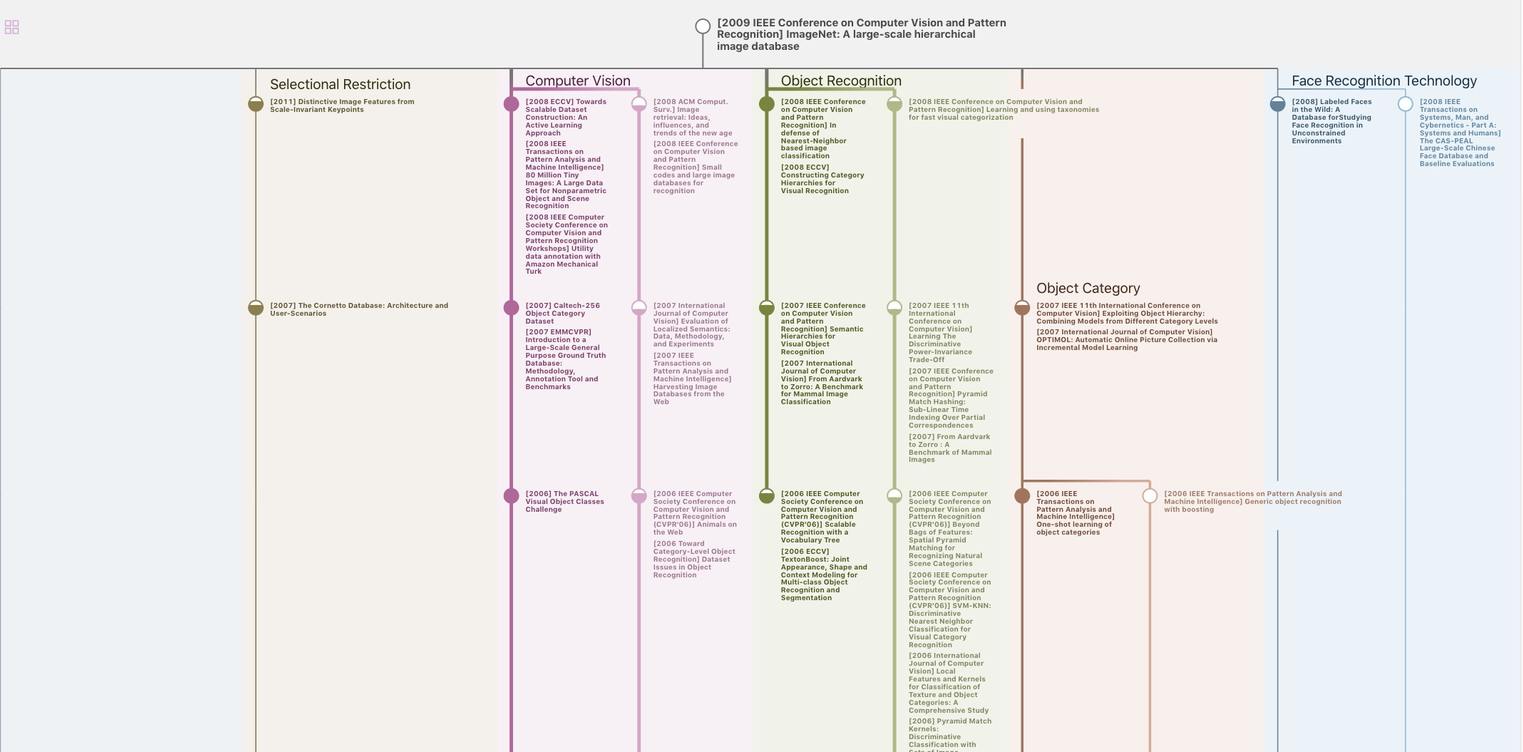
生成溯源树,研究论文发展脉络
Chat Paper
正在生成论文摘要