Learning-based actuator selection for increased attack resilience of uncertain systems
AUTOMATICA(2024)
摘要
In this paper, we consider a security-aware and learning-based actuator selection formulation for cyber-physical systems. A set of actuators is chosen to be used by the system so that a metric of controllability and actuation attack resilience is maximized, given only partial knowledge of the physics of the system. Such a metric is related to the trace of a game theoretic Gramian, which is obtained by solving a zero-sum game between a defender who acts as a minimizer and wants to regulate the system, and an attacker who acts as a maximizer with an eventual goal to disrupt the regulation. To account for the lack of full knowledge of the system dynamics, we design an estimator that can learn the trace of the proposed game theoretic Gramian online, by utilizing data obtained across the system's trajectories. Subsequently, we propose a scheduling algorithm that utilizes the estimator to select actuators for the system in an online manner. In fact, we prove that the set of actuators that optimize the game-based metric will be learnt and scheduled permanently in finite time. Finally, we show that the learning-based actuator placement procedure can be utilized, in its steady state, to detect a large class of actuation attacks in a partially model-free manner. We carry out a simulation on an aircraft model to show the efficacy of our framework. (c) 2023 Elsevier Ltd. All rights reserved.
更多查看译文
关键词
Actuator selection,Learning,Partially unknown systems,Game theory,Cyber-physical systems
AI 理解论文
溯源树
样例
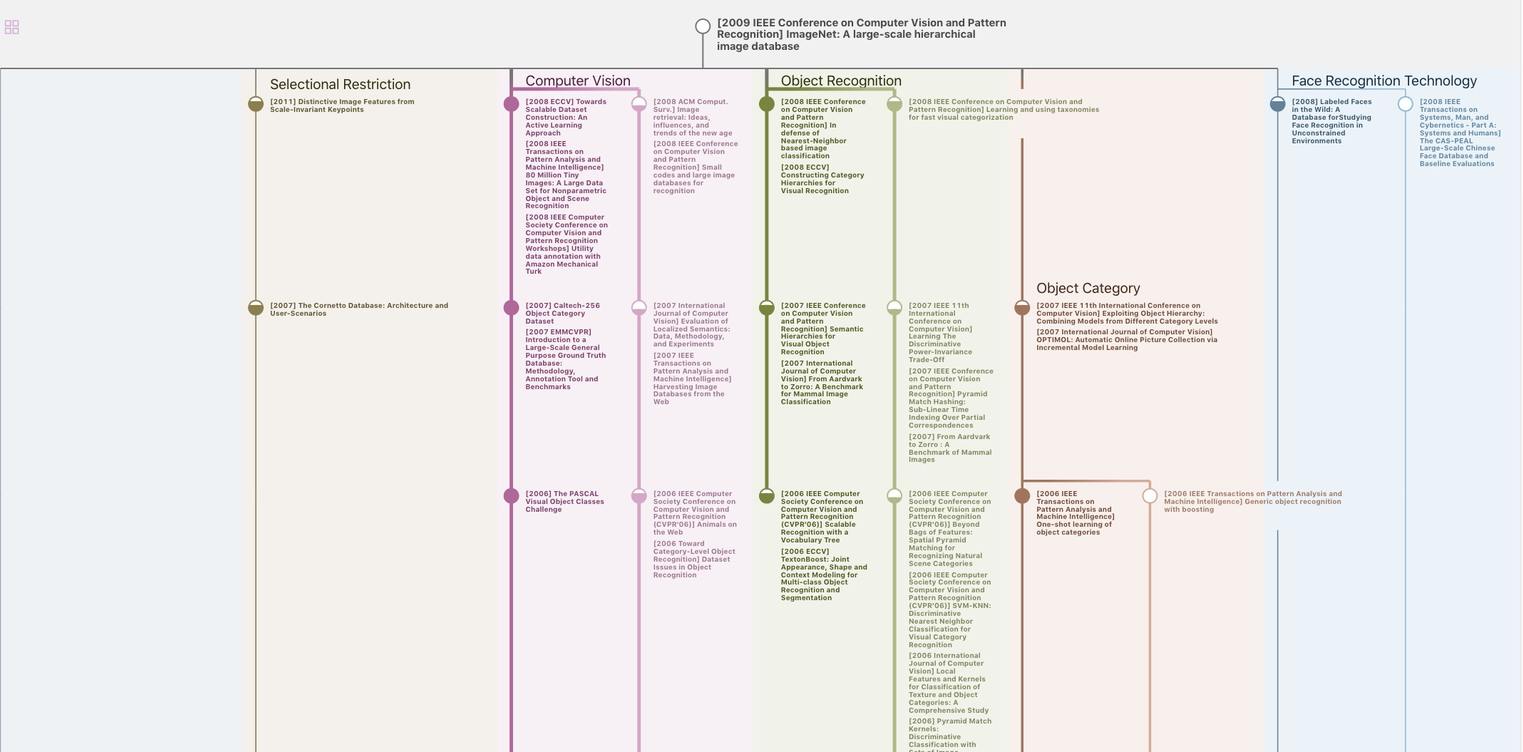
生成溯源树,研究论文发展脉络
Chat Paper
正在生成论文摘要