Multi-scale reinforced profile for personalized recommendation with deep neural networks in MOOCs
Applied Soft Computing(2023)
摘要
Course recommendation technology plays a key role in online learning services. However, there are still two key issues that remain unresolved in practice. First, it is difficult to characterize learners’ multiple preferences in various courses, while existing course recommendation methods are incompetent to address it. Second, there is a critical challenge to learn effective recommendation strategies due to the few labeled data, since online course resources usually include insufficient information about learners’ behaviors (e.g., lack of ratings and comments). To deal with these issues, we propose a joint learning framework, namely multi-scale Reinforced Profile for Personalized Recommendation (RPPR). Specifically, we design a multi-scale deep reinforcement learning method to construct learners’ multiple profiles according to coarse-grained and fine-grained semantics. Moreover, to adaptively adjust the recommendation strategies from limited interactive information, we develop an attention-based recommendation model with multivariable perceptron, which leverages related parameters of both neural networks and data structure to control the direction of loss optimization. Empirical results demonstrate that our RPPR significantly outperforms some competitive baselines on two real-world MOOCs (Massive Open Online Courses) datasets.
更多查看译文
关键词
Personalized recommendation,Course recommendation,Reinforcement learning,Attention networks
AI 理解论文
溯源树
样例
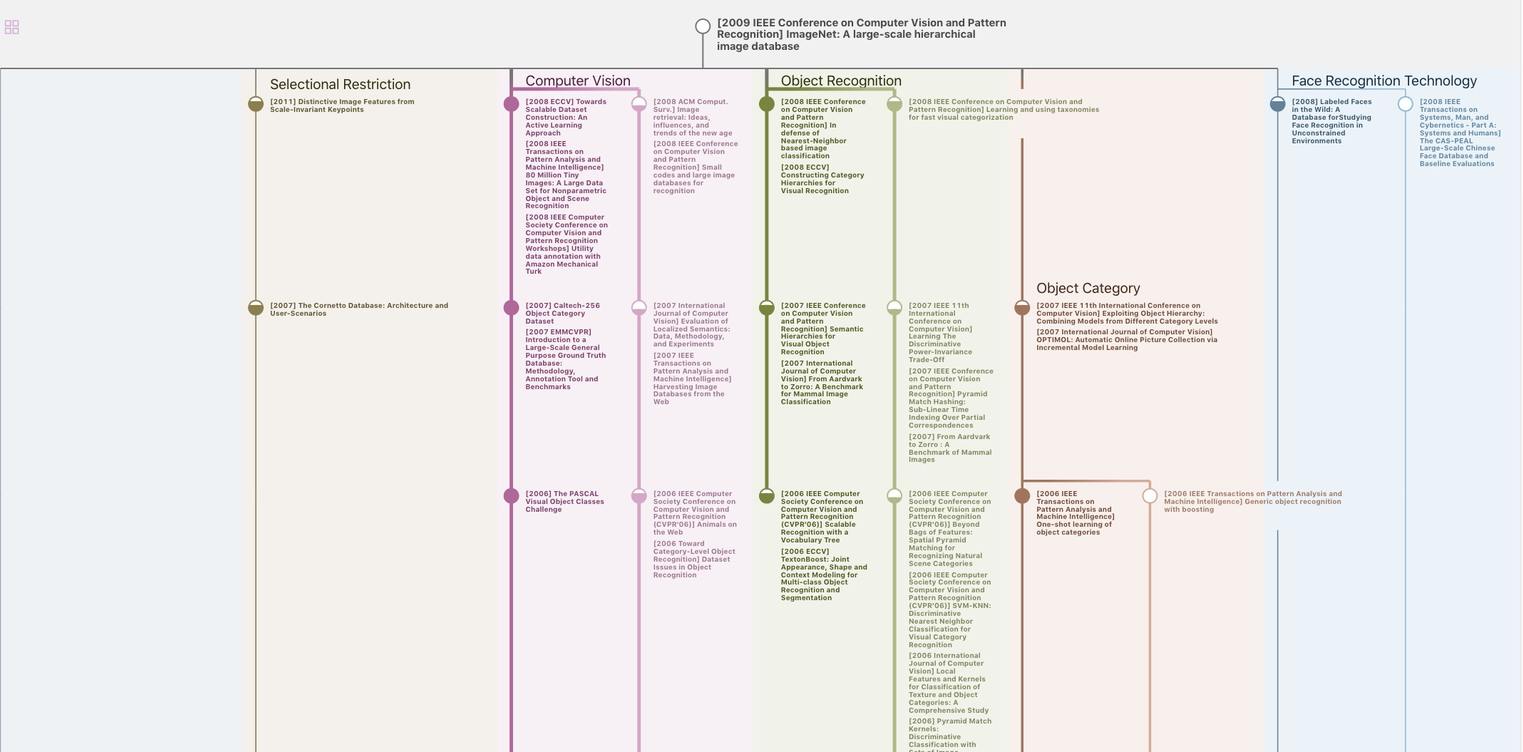
生成溯源树,研究论文发展脉络
Chat Paper
正在生成论文摘要