A feature-thresholds guided genetic algorithm based on a multi-objective feature scoring method for high-dimensional feature selection
APPLIED SOFT COMPUTING(2023)
摘要
The classical genetic algorithm utilizes random population initialization, an unguided crossover operator, and an unguided mutation operator for feature selection. However, this approach may be too stochastic and result in slow convergence. This paper proposes a hybrid feature selection algorithm named the Feature-Thresholds Guided Genetic Algorithm (FTGGA) to overcome this deficiency. FTGGA first employs ReliefF to filter out redundant features and retains crucial ones. Then, it generates a feature-thresholds set that contains all the feature thresholds. Each feature threshold represents the probability that the corresponding feature will be selected. The feature-thresholds set continuously updates to guide the iteration process of the genetic algorithm, accelerating its convergence. The experimental data demonstrates that FTGGA has a smaller feature subset and better classification accuracy compared to other algorithms.
更多查看译文
关键词
Gene selection,Feature selection,Genetic algorithm,Feature score,Feature threshold
AI 理解论文
溯源树
样例
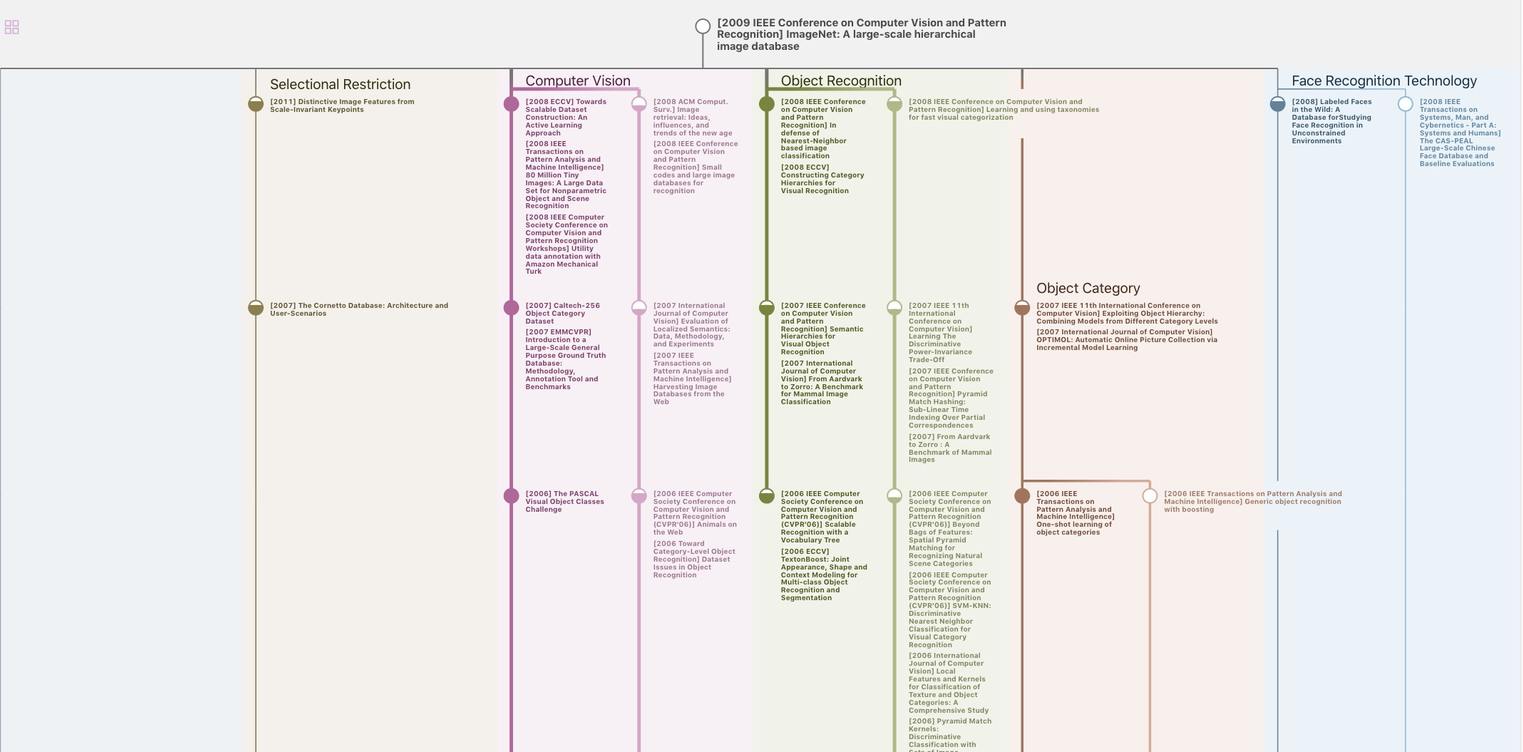
生成溯源树,研究论文发展脉络
Chat Paper
正在生成论文摘要