Personalized and adaptive neural networks for pain detection from multi-modal physiological features
EXPERT SYSTEMS WITH APPLICATIONS(2024)
摘要
Pain assessment is essential for pain diagnosis and treatment. Automating the assessment process from pain behaviors could be an alternative to self-report; however, inter-subject and time-dynamic differences in pain behaviors hinder pain recognition as generic patterns. To address this problem, we proposed a neural network method integrating pain sensitivity in personalized feature fusion and dynamic feature attention leveraging the Squeeze-and-Excitation block. Ablation results from our physiological pain data show that dynamic attention effectively improved prediction recall through soft physiological feature selection, and fusing pain sensitivity improved precision, yielding better F1-score together. By testing our trained models with external BioVid Heat Pain data, we observed better adaptivity to a different pain protocol with higher accuracy in time-continuous pain detection than simple neural networks. At last, we found our method outperformed SOTA works using the same public database in pain intensity classification and regression, reaching 84.58% accuracy in high pain detection with model pretraining.
更多查看译文
关键词
Pain assessment,Multi-modal fusion,Pain sensitivity,Attention mechanism,Neural networks
AI 理解论文
溯源树
样例
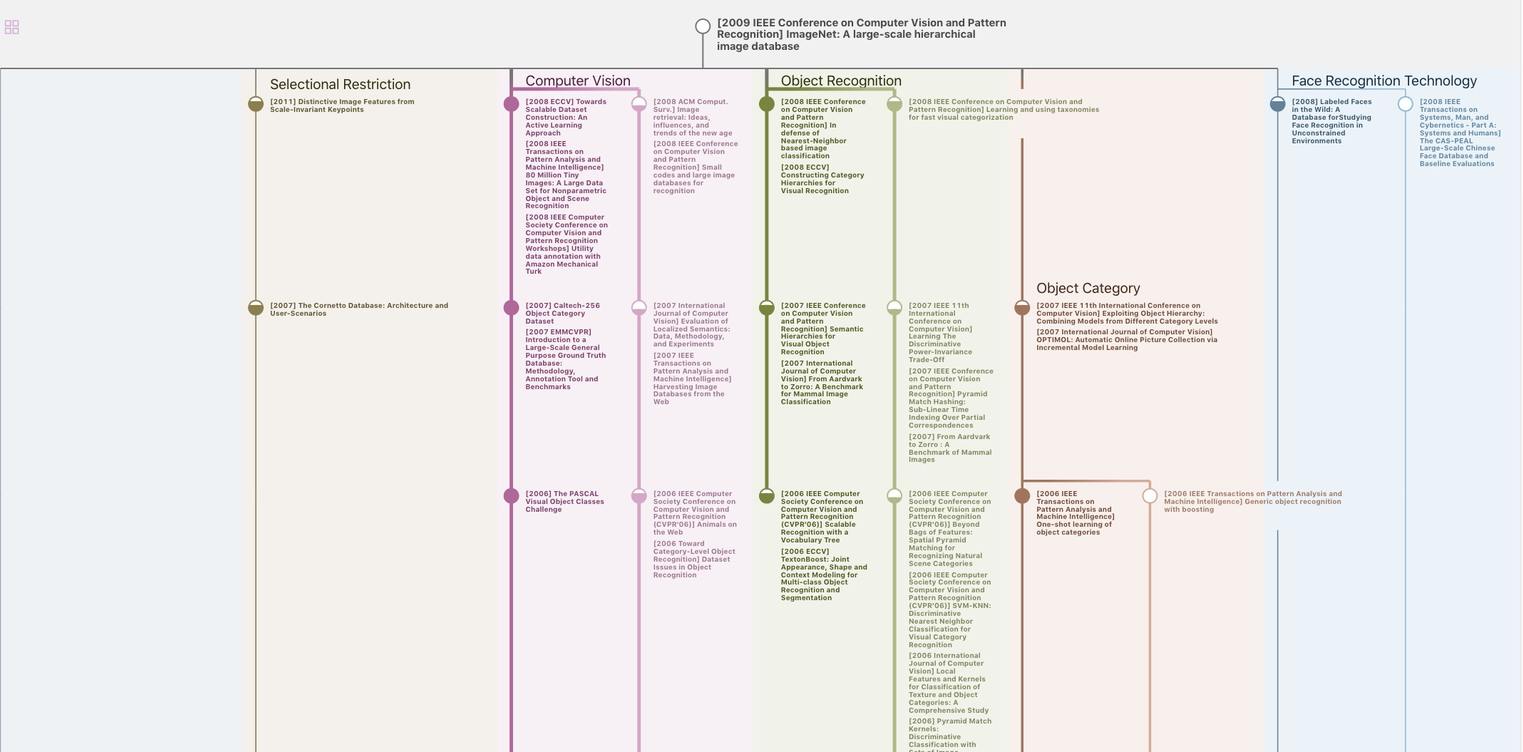
生成溯源树,研究论文发展脉络
Chat Paper
正在生成论文摘要