Feature relevance and redundancy coefficients for multi-view multi-label feature selection
INFORMATION SCIENCES(2024)
摘要
Multi-view and multi-label data offer a comprehensive perspective for learning models, but dimensionality poses a challenge for feature selection. Existing methods based on information theory solely focus on feature contribution to the entire label set, neglecting balanced information gain across labels and issues of feature overlap. They also miss the opportunity to exploit correlations between views, features, and labels. To address these challenges, our novel method combines feature relevance and redundancy terms. The feature relevance term uses label information coefficients to consider each label's information gain, while the feature redundancy term employs view redundancy coefficients to account for feature redundancy within and between views. This approach balances label information acquisition and reduces feature information redundancy. We evaluate our proposed method on six real-world datasets, comparing it with two existing multi-view multi-label feature selection methods and four multi-label feature selection methods. The results show that our method, RRFS, outperforms the other six methods overall.
更多查看译文
关键词
Multi-view multi-label learning,Feature selection,Information theory,Relevance based weight,Redundancy based weight
AI 理解论文
溯源树
样例
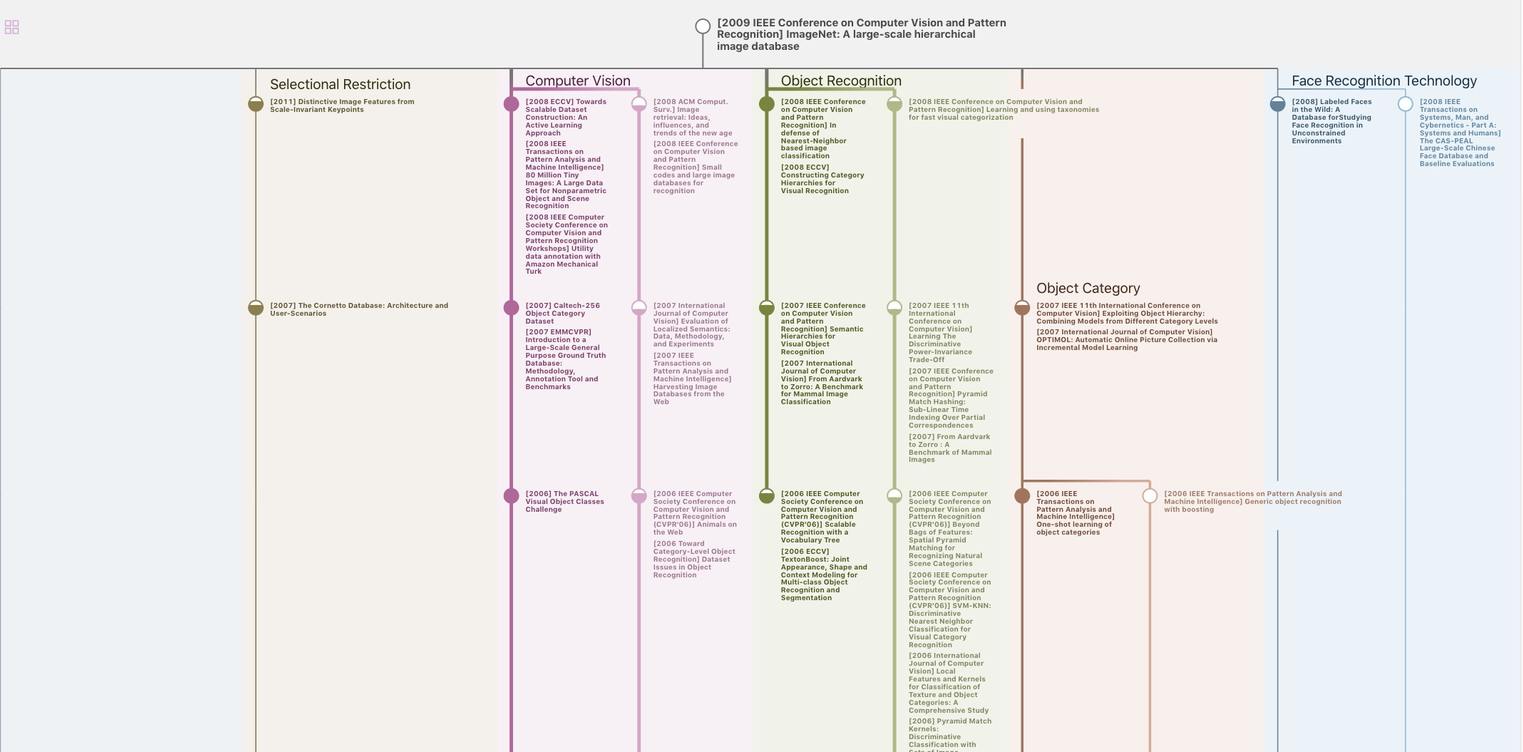
生成溯源树,研究论文发展脉络
Chat Paper
正在生成论文摘要