Hyperspectral classification method based on M-ResHSDC
MULTIMEDIA TOOLS AND APPLICATIONS(2023)
摘要
The hyperspectral image (HSI) classification method based on a three-dimensional convolutional neural network (3DCNN) has problems with overfitting in the training process, and difficulty in interacting with long-distance features, which decreases the classification accuracy. This paper proposes a hyperspectral classification method based on a mix-up algorithm and residual hybrid spectral dilated convolution (M-ResHSDC) to deal with the above problems. Firstly, the mix-up algorithm is utilized for increasing the original dataset to a larger number to alleviate the overfitting problem. Secondly, the hybrid spectral dilated convolution (HSDC) is built up through the combination of the spectral dilated convolution operators with different dilated rates. Each branch of HSDC has a different dilated rate, facilitating the extraction of spectral-spatial features at multiple scales. The ability to capture multiple scales could significantly enhance classification accuracy. On this basis, residual hybrid spectral dilated convolution (ResHSDC) is proposed by replacing the convolution layer of the residual block with HSDC. Thirdly, three ResHSDCs are introduced to replace the three convolution layers of 3DCNN respectively to solve the difficult interaction of long-distance features. Finally, the experiments are conducted on three HSI datasets (Indian Pines, University of Pavia, and Salinas). The results show a higher accuracy of 98.87%, 99.32%, and 99.47% respectively than the traditional methods.
更多查看译文
关键词
Hyperspectral Image Classification,Convolutional Neural Network,Mix-up algorithm,Hybrid spectral dilated convolution
AI 理解论文
溯源树
样例
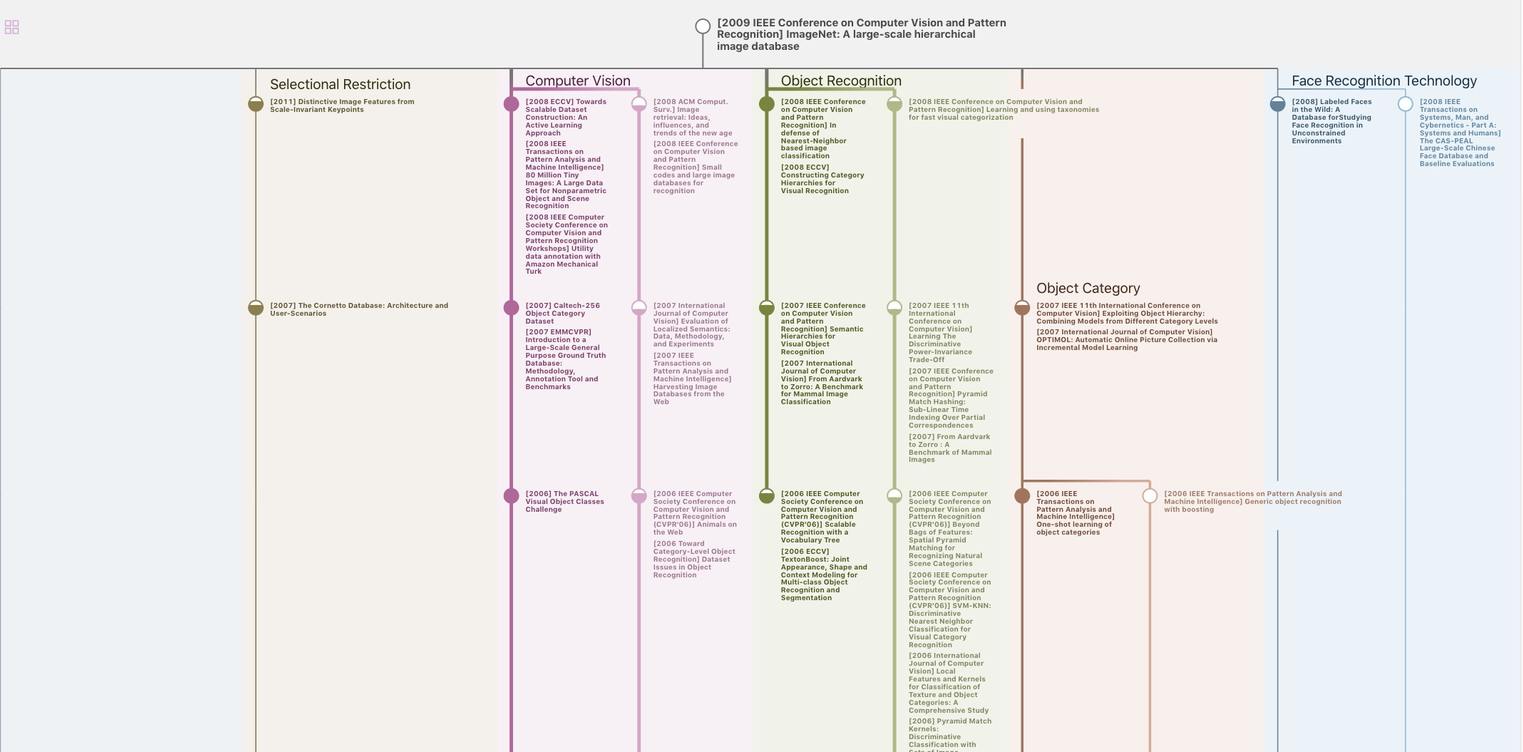
生成溯源树,研究论文发展脉络
Chat Paper
正在生成论文摘要