WCDForest: a weighted cascade deep forest model toward the classification tasks
APPLIED INTELLIGENCE(2023)
摘要
The deep forest model, a random forest (RF) ensemble approach and an alternative to Deep Neural Network (DNN), has performance highly competitive to DNN in many classification tasks. However, deep forest model may encounter overfitting and characteristic dispersion issues as processing small-scale, class-imbalance or high-dimension data. Therefore, this paper proposes a Weighted Cascade Deep Forest framework, called WCDForest. In WCDForest, an equal multi-grained scanning module is used to scan each feature equally. Meanwhile, this framework adopts a class vector weighting module to emphasis the performance of each forest and each sliding window by weight. Furthermore, this study proposes a feature enhancement module to reduce the information loss in the first few cascade layers to improve the classification accuracy. Subsequently, systematic comparison experiments on 18 widely used public datasets demonstrate that the proposed model outperforms the state-of-the-art model. In particular, WCDForest improves the accuracy, precision, recall and F1-score by an average of 5.47%,7.04%,8.23% and 8.94%,respectively.
更多查看译文
关键词
Ensemble method,Deep learning,Deep forest model,Classification tasks
AI 理解论文
溯源树
样例
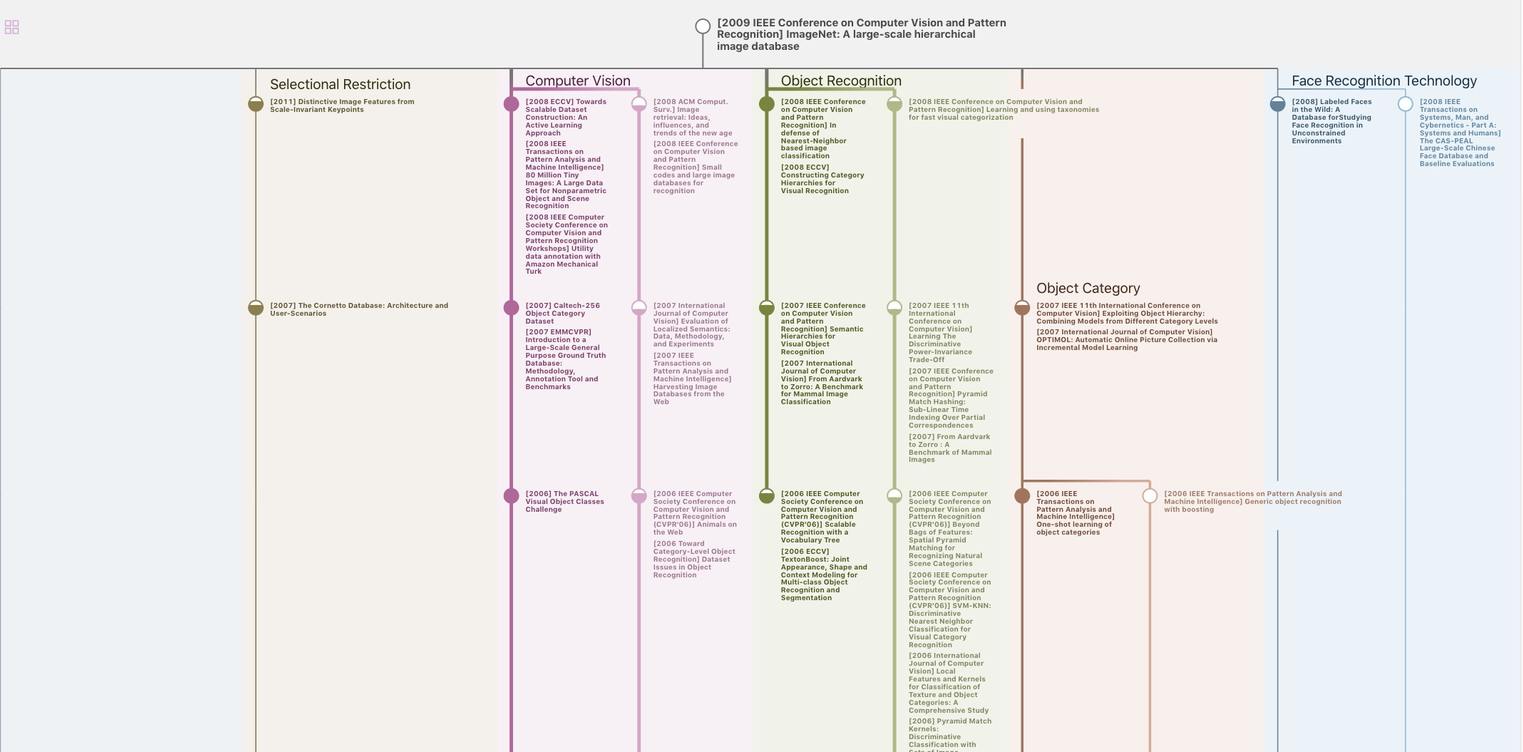
生成溯源树,研究论文发展脉络
Chat Paper
正在生成论文摘要