176P Enhancing immunotherapy response prediction via multimodal integration of radiology and pathology deep learning models
Annals of Oncology(2023)
摘要
The growing need for better predictive biomarkers in immunotherapy has driven the development of AI models across various fields. Despite this progress, most studies focus on single modalities, such as pathology or radiology. This study emphasizes the importance of multimodal biomarkers, combining radiology and pathology data, to optimize treatment outcomes and advance personalized medicine in immunotherapy. We developed weakly-supervised deep learning (DL) models for CT and PDL1-stained immunohistochemistry (IHC) images. We trained a CT-based model to predict immunotherapy clinical benefit (PFS > 5 months) in a pan-cancer cohort from VHIO(n=369). Simultaneously, we trained a DL method to predict PD-L1 status from IHC images in an open source NSCLC cohort (n=233), validated in a pan-cancer cohort from VHIO (n=106). The model predictions of both modalities were aggregated and utilized for patient stratification in immunotherapy response. In the overlapping subset with both modalities' data (n=75), we explored the association between PFS and the combination of predicted clinical benefit by CT and predicted PDL1 status by IHC using Cox regression and log-rank test. The CT-based and IHC-based DL models exhibited effective performance in predicting PFS for immunotherapy response in the pan-cancer cohort, with hazard ratios (HR) of 0.62 [95% CI 0.5-0.78], p <0.05 and 0.66 [95% CI 0.44-1], p <0.05, respectively. In the multi-modal data subsets, patients classified as clinical benefit prediction by the CT-based model and PDL1 high by the IHC-based model (n = 20) exhibited better immunotherapy response (HR 0.44 [0.25-0.78], p <0.05) with longer PFS (median PFS 4.08 [2.70-10.91] months) compared to the rest (median PFS 1.81 [1.68-2.96] months). Integrating radiology and pathology data improves response prediction compared to single modality models, emphasizing the potential of a multimodal approach for better patient stratification in immunotherapy.
更多查看译文
关键词
immunotherapy response prediction,deep learning models,deep learning,multimodal integration
AI 理解论文
溯源树
样例
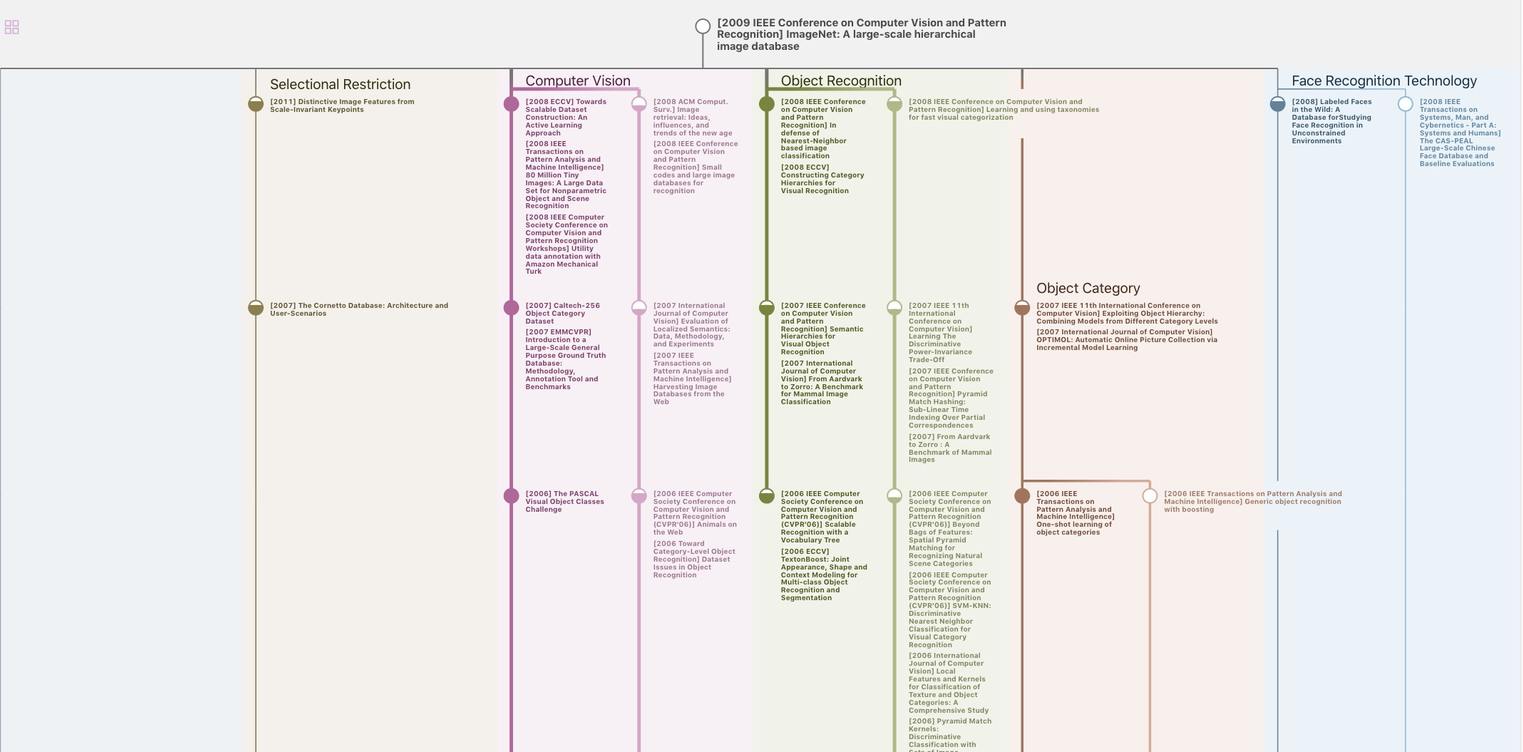
生成溯源树,研究论文发展脉络
Chat Paper
正在生成论文摘要