MixerFlow for Image Modelling
CoRR(2023)
摘要
Normalising flows are statistical models that transform a complex density into a simpler density through the use of bijective transformations enabling both density estimation and data generation from a single model. In the context of image modelling, the predominant choice has been the Glow-based architecture, whereas alternative architectures remain largely unexplored in the research community. In this work, we propose a novel architecture called MixerFlow, based on the MLP-Mixer architecture, further unifying the generative and discriminative modelling architectures. MixerFlow offers an effective mechanism for weight sharing for flow-based models. Our results demonstrate better density estimation on image datasets under a fixed computational budget and scales well as the image resolution increases, making MixeFlow a powerful yet simple alternative to the Glow-based architectures. We also show that MixerFlow provides more informative embeddings than Glow-based architectures.
更多查看译文
关键词
modelling,image
AI 理解论文
溯源树
样例
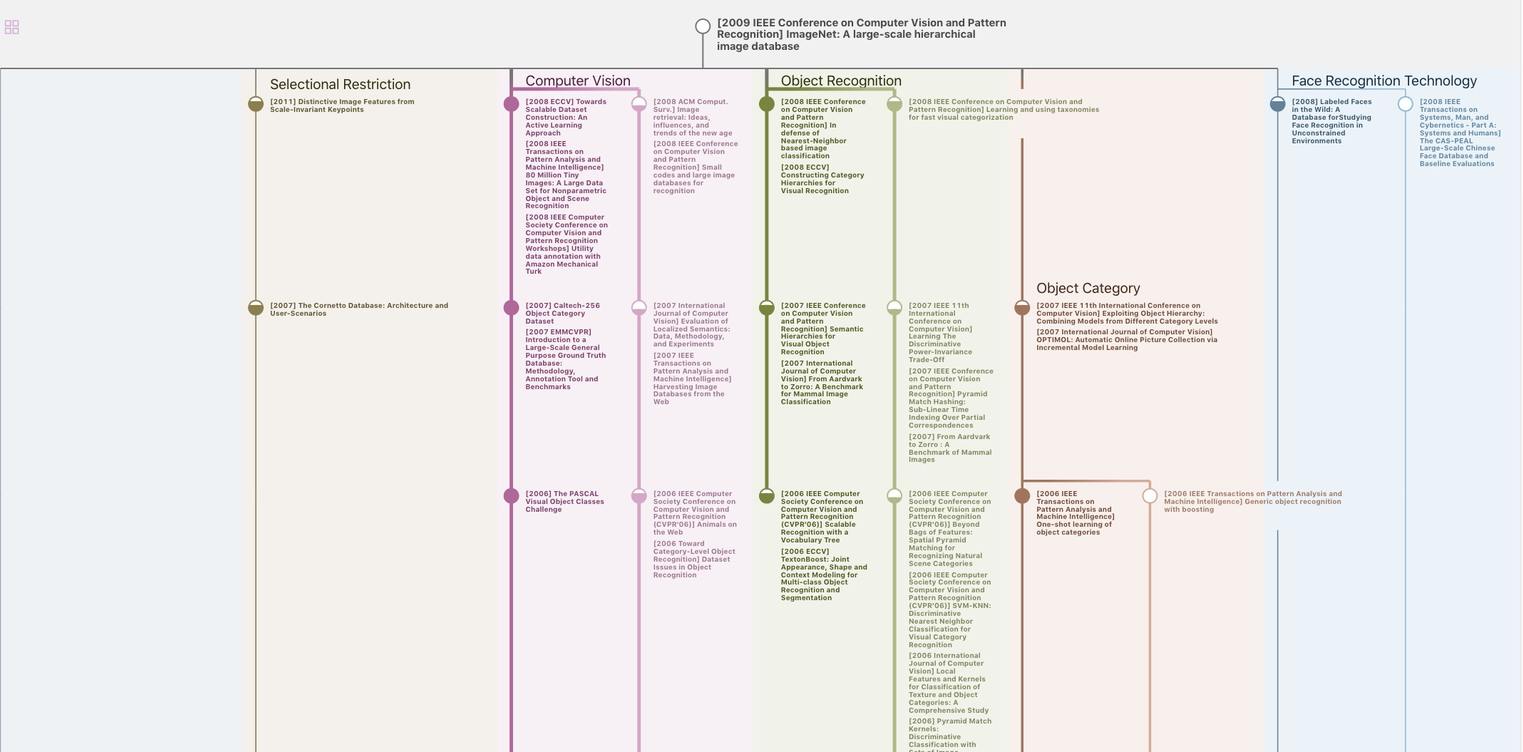
生成溯源树,研究论文发展脉络
Chat Paper
正在生成论文摘要