Improving a Named Entity Recognizer Trained on Noisy Data with a Few Clean Instances
CoRR(2023)
摘要
To achieve state-of-the-art performance, one still needs to train NER models on large-scale, high-quality annotated data, an asset that is both costly and time-intensive to accumulate. In contrast, real-world applications often resort to massive low-quality labeled data through non-expert annotators via crowdsourcing and external knowledge bases via distant supervision as a cost-effective alternative. However, these annotation methods result in noisy labels, which in turn lead to a notable decline in performance. Hence, we propose to denoise the noisy NER data with guidance from a small set of clean instances. Along with the main NER model we train a discriminator model and use its outputs to recalibrate the sample weights. The discriminator is capable of detecting both span and category errors with different discriminative prompts. Results on public crowdsourcing and distant supervision datasets show that the proposed method can consistently improve performance with a small guidance set.
更多查看译文
关键词
named entity recognizer,noisy data,clean instances
AI 理解论文
溯源树
样例
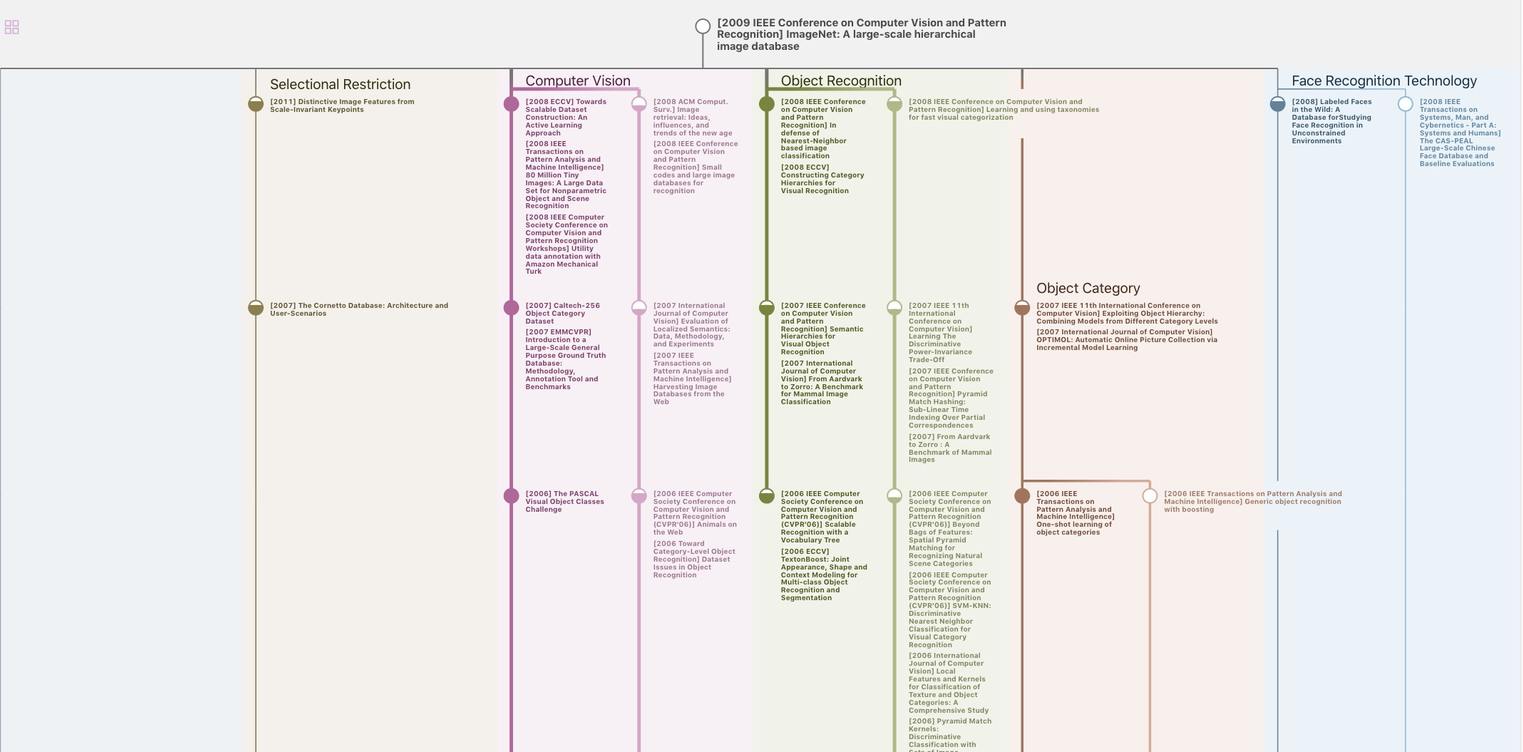
生成溯源树,研究论文发展脉络
Chat Paper
正在生成论文摘要