Improving Conversational Recommendation Systems via Bias Analysis and Language-Model-Enhanced Data Augmentation.
CoRR(2023)
摘要
Conversational Recommendation System (CRS) is a rapidly growing research area that has gained significant attention alongside advancements in language modelling techniques. However, the current state of conversational recommendation faces numerous challenges due to its relative novelty and limited existing contributions. In this study, we delve into benchmark datasets for developing CRS models and address potential biases arising from the feedback loop inherent in multi-turn interactions, including selection bias and multiple popularity bias variants. Drawing inspiration from the success of generative data via using language models and data augmentation techniques, we present two novel strategies, 'Once-Aug' and 'PopNudge', to enhance model performance while mitigating biases. Through extensive experiments on ReDial and TG-ReDial benchmark datasets, we show a consistent improvement of CRS techniques with our data augmentation approaches and offer additional insights on addressing multiple newly formulated biases.
更多查看译文
关键词
conversational recommendation systems,bias analysis,augmentation,language-model-enhanced
AI 理解论文
溯源树
样例
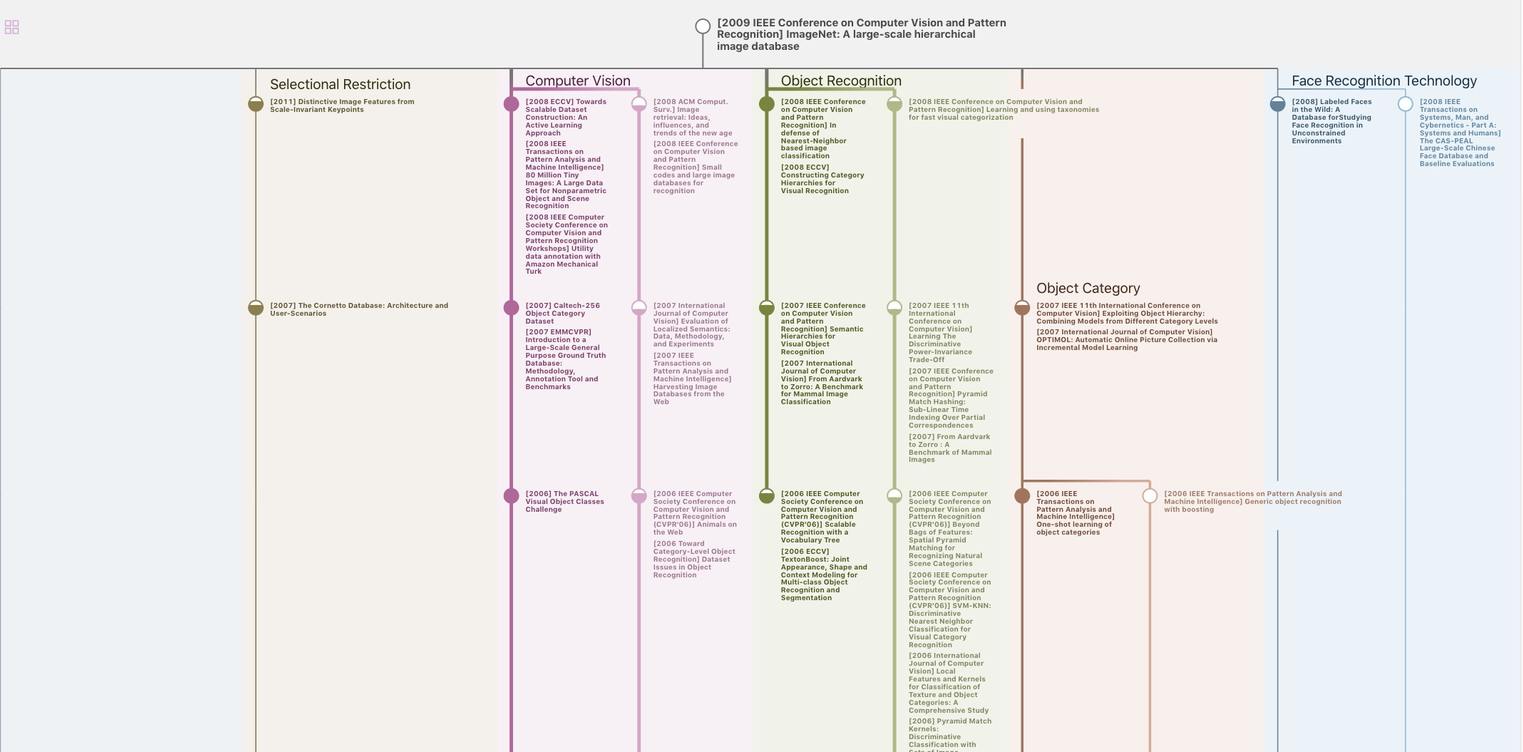
生成溯源树,研究论文发展脉络
Chat Paper
正在生成论文摘要